Students Temporal Profiling and e-Learning Resources Recommendation Based on NLP's Terms Extraction
2022 IEEE International Conference on Data Mining Workshops (ICDMW)(2022)
摘要
Online education has gained significant relevance over the last few years, and the pandemic situation has brought evidence that it plays a fundamental role nowadays. However, even with the increasing number of students enrolled in online courses, these still do not allow for enough personalization, often leading students to become demotivated and dropping out. The goal of better adapting online courses to students aims to support them in an inclusive and equitable way, since the learners are often students from quite diverse backgrounds. The continuous demand for online learning, and the need to customize it according to the students' profile has led to a succession of attempts at recommendation systems. Nevertheless, many of them were entirely based on collaborative filtering, almost ignoring profiling requirements. In this paper, we propose a recommendation system to be integrated into MOOCs (Massive Open Online Courses), following a hybrid architecture. In our proposal, learning resources are described by a set of terms, extracted directly from the supporting texts in the MOOC. From these terms, those which are included in the exercises will be used to specify the important skills learners must acquire, and the results achieved by each learner in them are used to characterize the particular student's state, at a given moment. Those states are then used to make the recommendation collaboratively, allowing for different recommendations for each particular student over time. The system is validated across several MOOCs.
更多查看译文
关键词
Recommendation System,MODCs,Learning Resource Recommendation
AI 理解论文
溯源树
样例
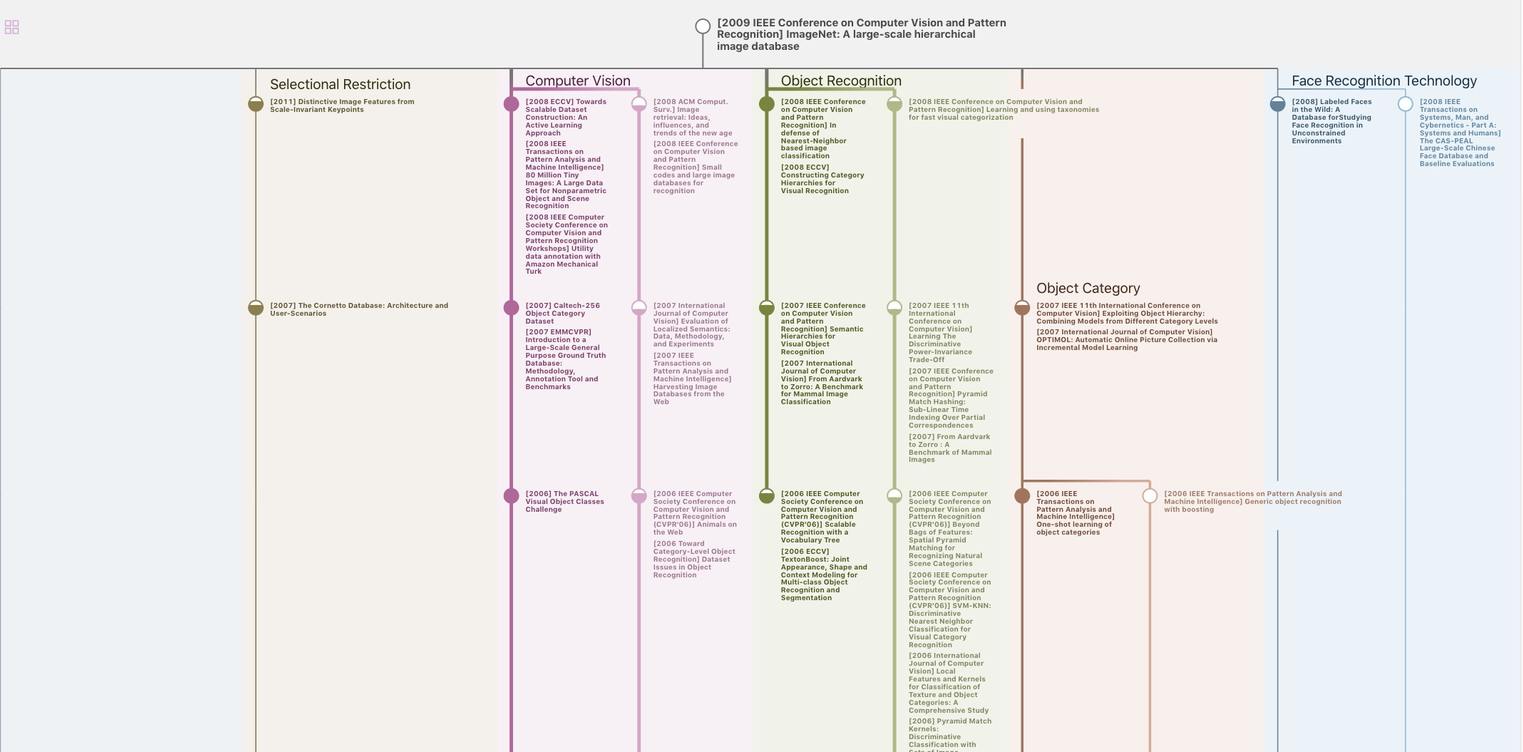
生成溯源树,研究论文发展脉络
Chat Paper
正在生成论文摘要