A Boolean-based machine learning framework identifies predictive biomarkers of HSP90-targeted therapy response in prostate cancer.
Frontiers in molecular biosciences(2023)
摘要
Precision medicine has emerged as an important paradigm in oncology, driven by the significant heterogeneity of individual patients' tumour. A key prerequisite for effective implementation of precision oncology is the development of companion biomarkers that can predict response to anti-cancer therapies and guide patient selection for clinical trials and/or treatment. However, reliable predictive biomarkers are currently lacking for many anti-cancer therapies, hampering their clinical application. Here, we developed a novel machine learning-based framework to derive predictive multi-gene biomarker panels and associated expression signatures that accurately predict cancer drug sensitivity. We demonstrated the power of the approach by applying it to identify response biomarker panels for an Hsp90-based therapy in prostate cancer, using proteomic data profiled from prostate cancer patient-derived explants. Our approach employs a rational feature section strategy to maximise model performance, and innovatively utilizes Boolean algebra methods to derive specific expression signatures of the marker proteins. Given suitable data for model training, the approach is also applicable to other cancer drug agents in different tumour settings.
更多查看译文
关键词
17-AAG,Boolean function minimization,Hsp90 inhibitor,feature selection,machine learning,precision oncology,predictive biomarker,prostate cancer
AI 理解论文
溯源树
样例
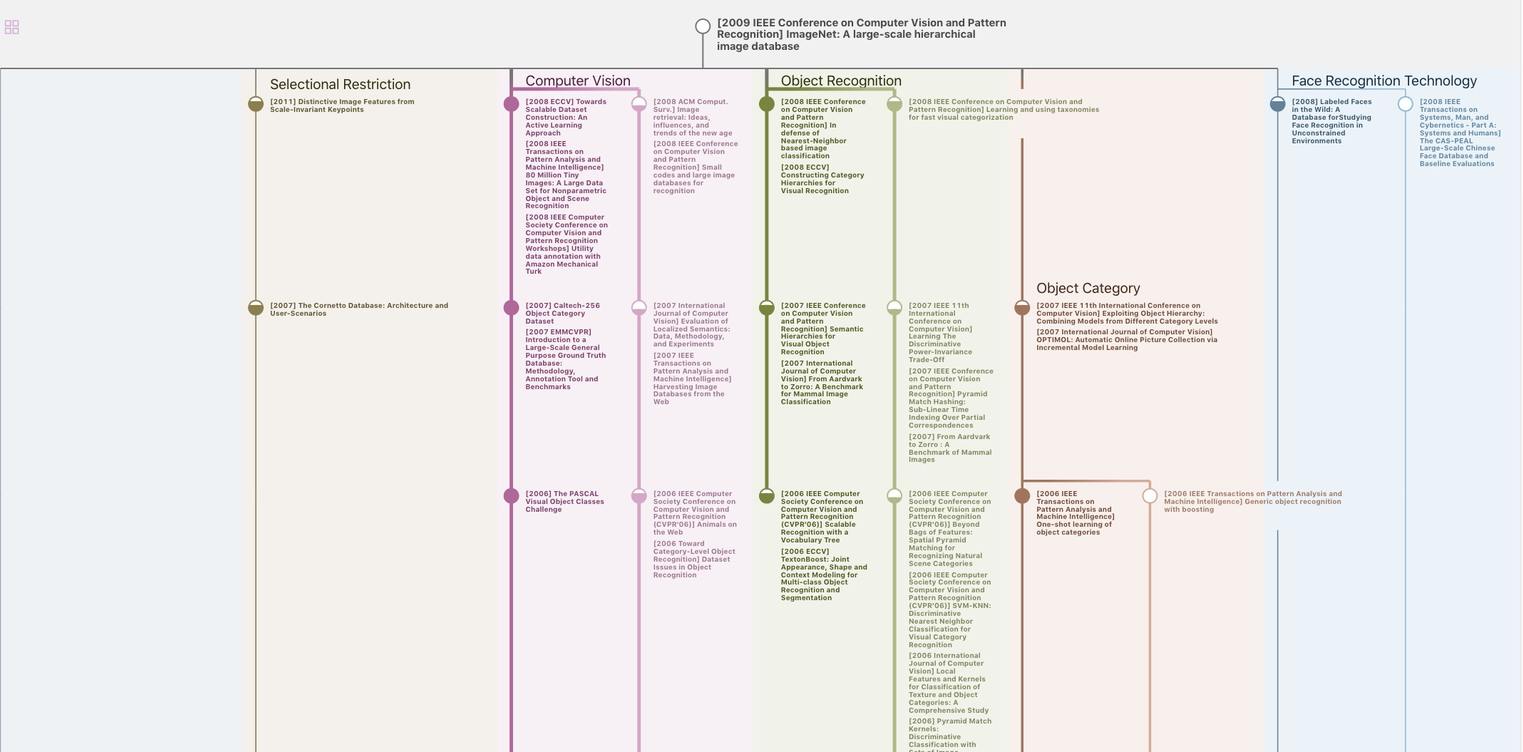
生成溯源树,研究论文发展脉络
Chat Paper
正在生成论文摘要