Convolutional Neural Networks Trained to Identify Words Provide a Surprisingly Good Account of Visual Form Priming Effects
arxiv(2023)
摘要
A wide variety of orthographic coding schemes and models of visual word identification have been developed to account for masked priming data that provide a measure of orthographic similarity between letter strings. These models tend to include hand-coded orthographic representations with single unit coding for specific forms of knowledge (e.g., units coding for a letter in a given position). Here we assess how well a range of these coding schemes and models account for the pattern of form priming effects taken from the Form Priming Project and compare these findings to results observed with 11 standard deep neural network models (DNNs) developed in computer science. We find that deep convolutional networks (CNNs) perform as well or better than the coding schemes and word recognition models, whereas transformer networks did less well. The success of CNNs is remarkable as their architectures were not developed to support word recognition (they were designed to perform well on object recognition), they classify pixel images of words (rather than artificial encodings of letter strings), and their training was highly simplified (not respecting many key aspects of human experience). In addition to these form priming effects, we find that the DNNs can account for visual similarity effects on priming that are beyond all current psychological models of priming. The findings add to the recent work of Hannagan et al. (2021) and suggest that CNNs should be given more attention in psychology as models of human visual word recognition.
更多查看译文
关键词
Orthographic coding,Deep neural networks,Human visual system,Form priming,Convolutional DNNs,Vision Transformers
AI 理解论文
溯源树
样例
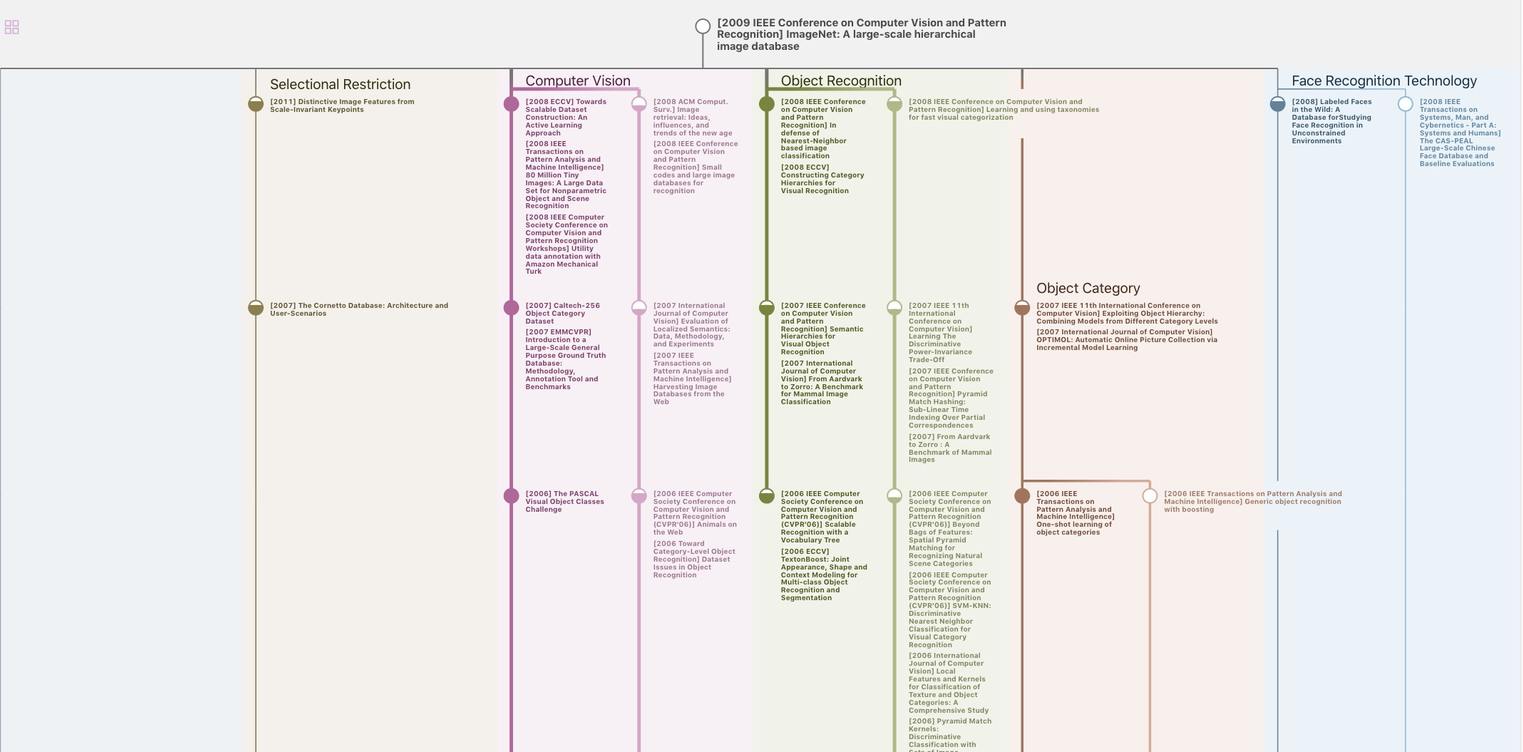
生成溯源树,研究论文发展脉络
Chat Paper
正在生成论文摘要