A Robust and Lightweight Environmental Sound Classification Technique with Adaptation to Microphone for AIoT Sound Sensing
2022 19th International SoC Design Conference (ISOCC)(2022)
摘要
In this work, we propose a robust and lightweight environmental sound classification (ESC) technique with adaptation to microphone. Firstly, a lightweight convolutional neural network (CNN) based ESC model with frame encoding is proposed to reduce the computational complexity while achieving high classification accuracy. Secondly, to address the accuracy degradation caused by microphone and improve the robustness, an adaptive-threshold trimming technique with incremental training is proposed. Compared with several state-of-the-art methods, the proposed ESC model reduces the number of parameters and FLOPs by up to 550 times and 1353 times while achieving a high classification accuracy of 88.05% on the ESC-50 dataset. With the adaptive-threshold trimming technique, the accuracy loss due to microphone is significantly reduced by 10.7%. The proposed ESC method is suitable for robust and lightweight AIoT sound sensing.
更多查看译文
关键词
ESC,CNN,Lightweight,Microphone Adaptation
AI 理解论文
溯源树
样例
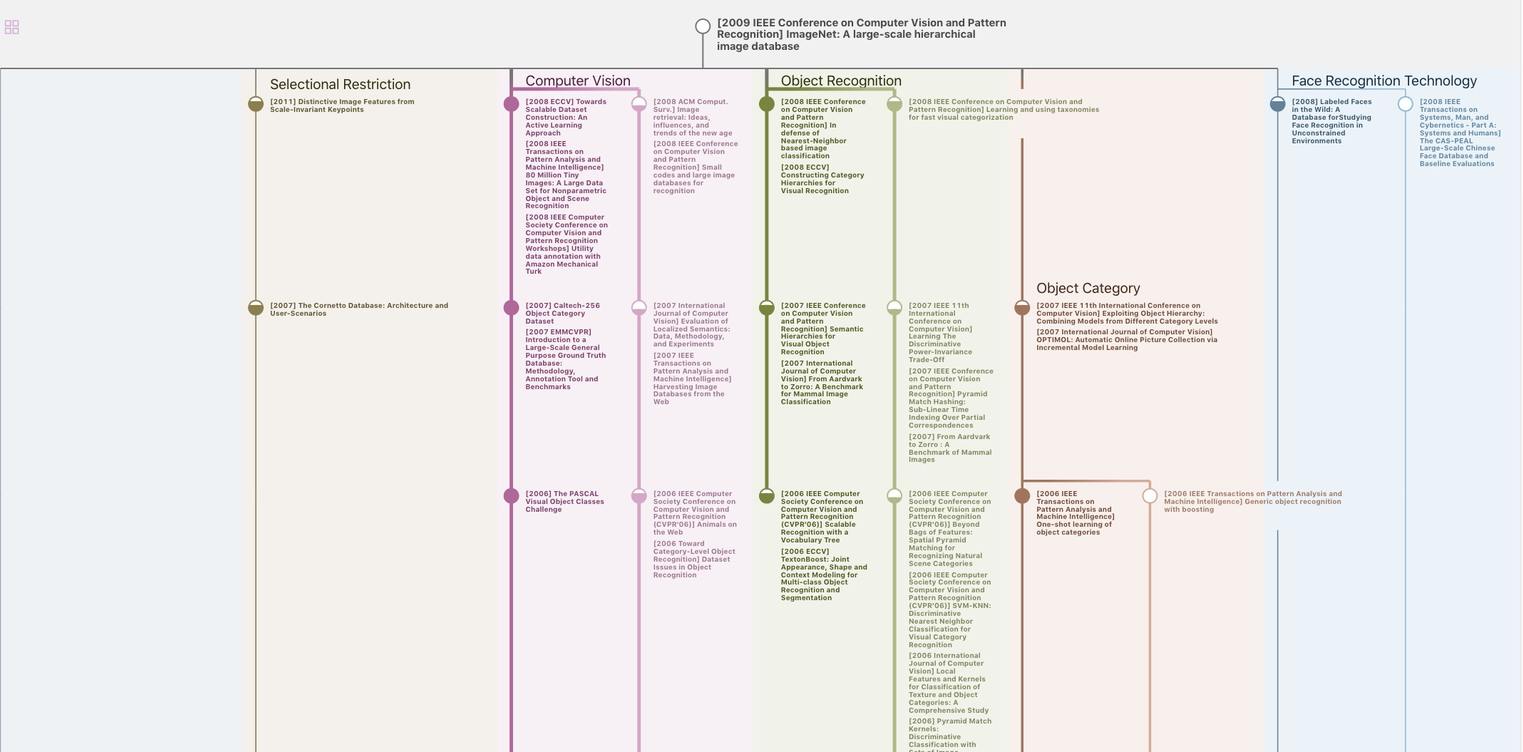
生成溯源树,研究论文发展脉络
Chat Paper
正在生成论文摘要