Uncoupled Learning of Differential Stackelberg Equilibria with Commitments
arxiv(2023)
摘要
A natural solution concept for many multiagent settings is the Stackelberg equilibrium, under which a ``leader'' agent selects a strategy that maximizes its own payoff assuming the ``follower'' chooses their best response to this strategy. Recent work has presented asymmetric learning updates that can be shown to converge to the \textit{differential} Stackelberg equilibria of two-player differentiable games. These updates are ``coupled'' in the sense that the leader requires some information about the follower's payoff function. Such coupled learning rules cannot be applied to \textit{ad hoc} interactive learning settings, and can be computationally impractical even in centralized training settings where the follower's payoffs are known. In this work, we present an ``uncoupled'' learning process under which each player's learning update only depends on their observations of the other's behavior. We prove that this process converges to a local Stackelberg equilibrium under similar conditions as previous coupled methods. We conclude with a discussion of the potential applications of our approach to human--AI cooperation and multi-agent reinforcement learning.
更多查看译文
关键词
differential stackelberg equilibria,learning
AI 理解论文
溯源树
样例
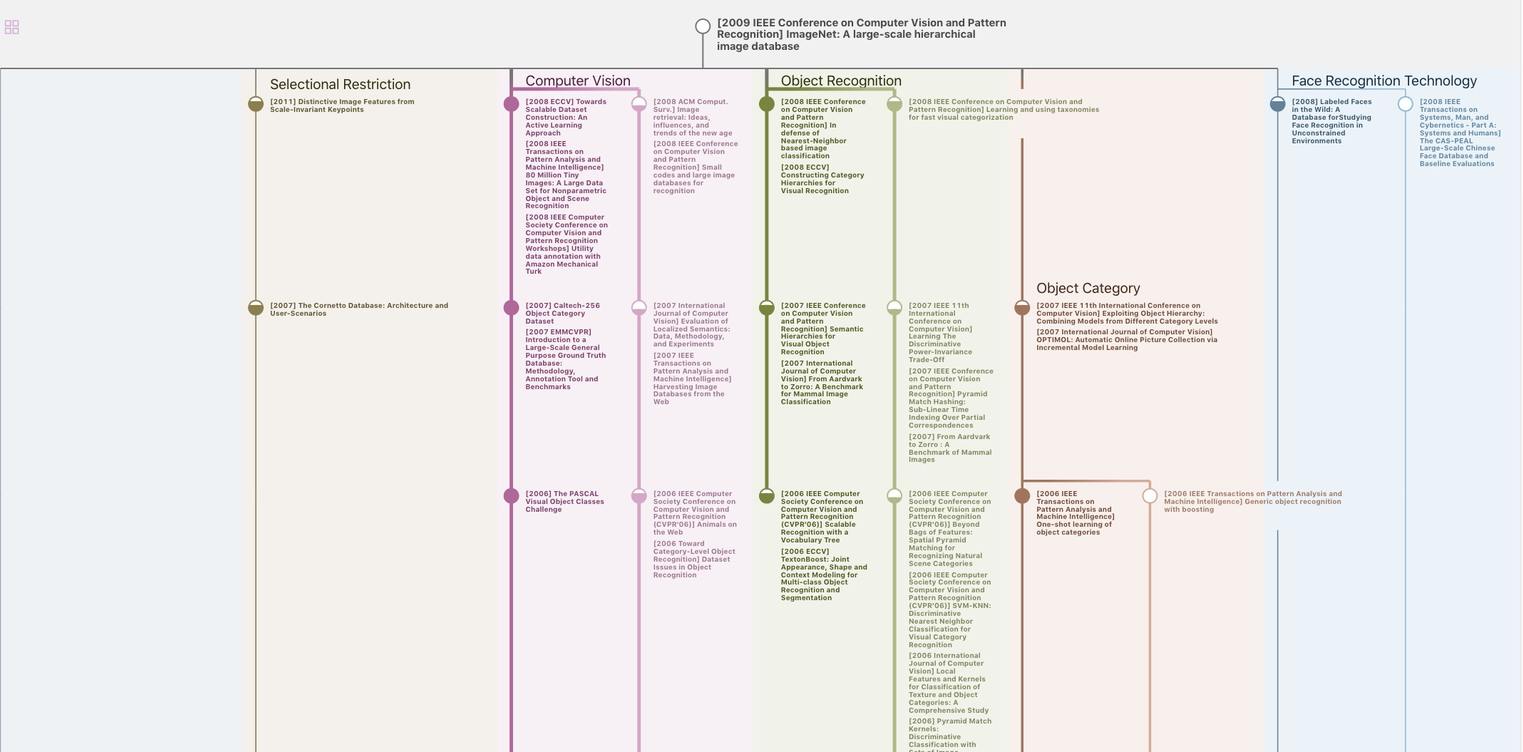
生成溯源树,研究论文发展脉络
Chat Paper
正在生成论文摘要