Dynamic Mixture of Counter Network for Location-Agnostic Crowd Counting
2023 IEEE/CVF Winter Conference on Applications of Computer Vision (WACV)(2023)
摘要
Crowd counting has attracted increasing attentions in recent years due to its challenges and wide societal applications. Despite persevering efforts made by the research community, most of existing methods require a large amount of location-level annotations. Collecting such type of fine-granularity supervisory signals is extremely time-consuming and labour-intensive, thereby hindering the well generalization of these location-adherent models. To shun this drawback, several pioneering studies open a promising research direction of location-agonistic crowd counting. Albeit the noticeable efforts, they somewhat ignore the merits of diverse learning paradigms and the issue of intractable density shift. To ameliorate these issues, in this paper, a novel Dynamic Mixture of Counter Network (DMCNet) is proposed for location-agnostic crowd counting. Specifically, our DMCNet inherits the hybrid advantages of CNNs (e.g. locality-oriented and pyramidal property) and MLP-based structure (e.g. global receptive fields and light weight). Particularly, the dynamic counter predictor and the mixture of counter heads are delicately designed to hammer at combating huge density shift and overfitting. Extensive experiments demonstrate that our DMCNet attains state-of-the-art performance against existing location-agnostic approaches and performs on par with many conventional location-adherent ones.
更多查看译文
关键词
Algorithms: Image recognition and understanding (object detection,categorization,segmentation),Machine learning architectures,formulations,and algorithms (including transfer,low-shot,semi-,self-,and un-supervised learning)
AI 理解论文
溯源树
样例
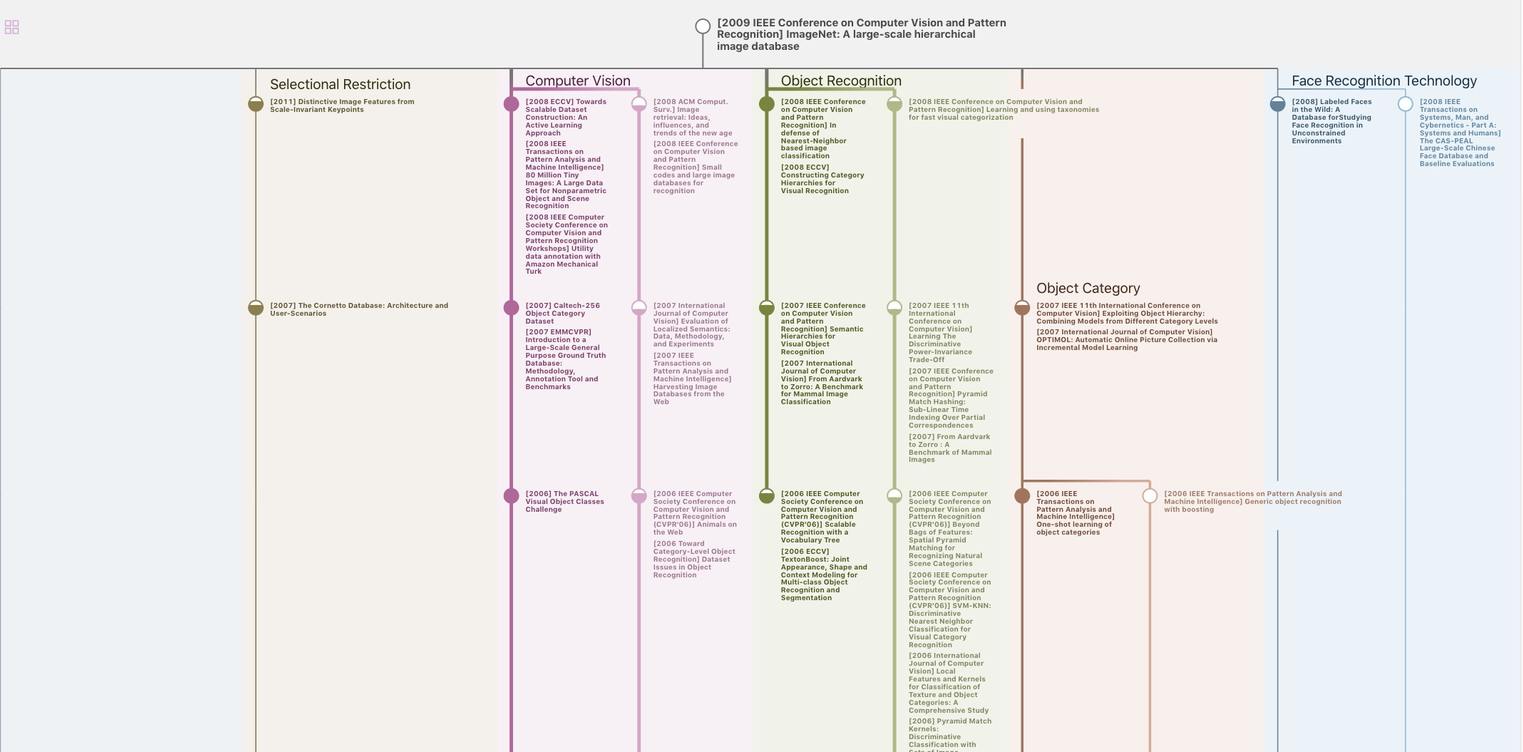
生成溯源树,研究论文发展脉络
Chat Paper
正在生成论文摘要