Solving Maxwell's Equation in 2D with Neural Networks with Local Converging Inputs
arxiv(2023)
摘要
In this paper we apply neural networks with local converging inputs (NNLCI), originally introduced in [arXiv:2109.09316], to solve the two dimensional Maxwell's equation around perfect electric conductors (PECs). The input to the networks consist of local patches of low cost numerical solutions to the equation computed on two coarse grids, and the output is a more accurate solution at the center of the local patch. We apply the recently developed second order finite difference method [arXiv:2209.00740] to generate the input and training data which captures the scattering of electromagnetic waves off of a PEC at a given terminal time. The advantage of NNLCI is that once trained it offers an efficient alternative to costly high-resolution conventional numerical methods; our numerical experiments indicate the computational complexity saving by a factor of $8^3$ in terms of the number of spatial-temporal grid points. In contrast with existing research work on applying neural networks to directly solve PDEs, our method takes advantage of the local domain of dependence of the Maxwell's equation in the input solution patches, and is therefore simpler, yet still robust. We demonstrate that we can train our neural network on some PECs to predict accurate solutions to different PECs with quite different geometries from any of the training examples.
更多查看译文
关键词
maxwell,neural networks
AI 理解论文
溯源树
样例
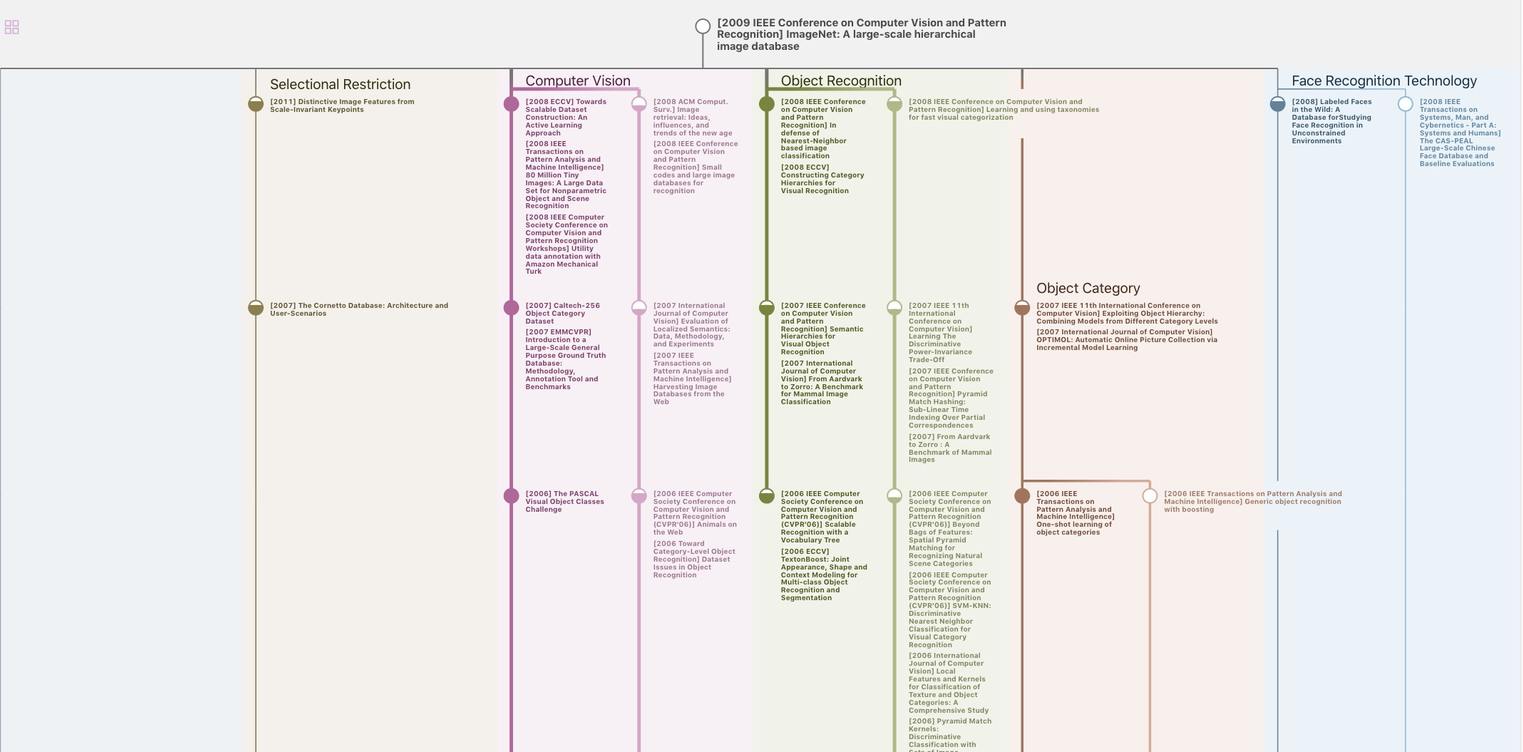
生成溯源树,研究论文发展脉络
Chat Paper
正在生成论文摘要