Less is More: Understanding Word-level Textual Adversarial Attack via n-gram Frequency Descend
arxiv(2023)
摘要
Word-level textual adversarial attacks have demonstrated notable efficacy in
misleading Natural Language Processing (NLP) models. Despite their success, the
underlying reasons for their effectiveness and the fundamental characteristics
of adversarial examples (AEs) remain obscure. This work aims to interpret
word-level attacks by examining their n-gram frequency patterns. Our
comprehensive experiments reveal that in approximately 90% of cases,
word-level attacks lead to the generation of examples where the frequency of
n-grams decreases, a tendency we term as the n-gram Frequency Descend
(n-FD). This finding suggests a straightforward strategy to enhance model
robustness: training models using examples with n-FD. To examine the
feasibility of this strategy, we employed the n-gram frequency information,
as an alternative to conventional loss gradients, to generate perturbed
examples in adversarial training. The experiment results indicate that the
frequency-based approach performs comparably with the gradient-based approach
in improving model robustness. Our research offers a novel and more intuitive
perspective for understanding word-level textual adversarial attacks and
proposes a new direction to improve model robustness.
更多查看译文
AI 理解论文
溯源树
样例
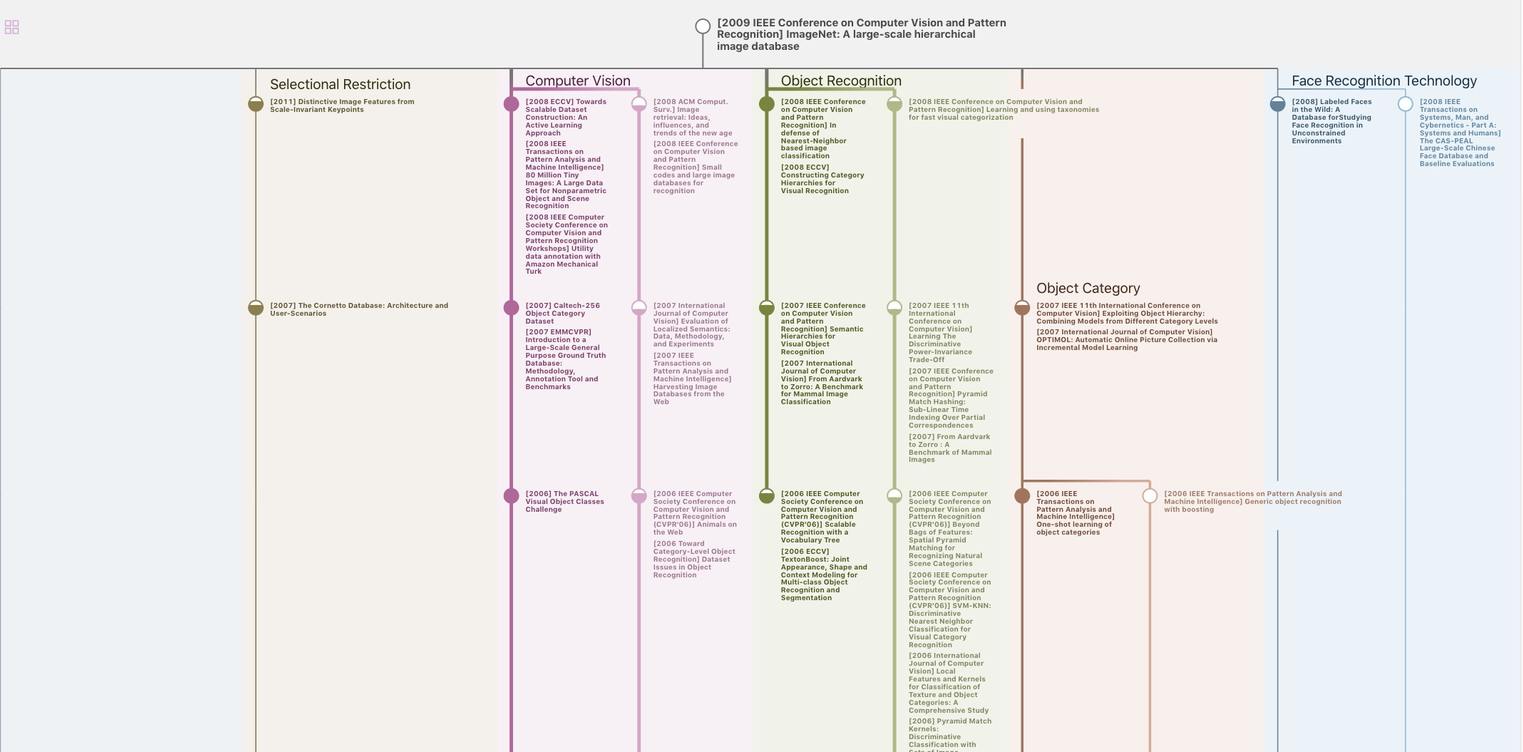
生成溯源树,研究论文发展脉络
Chat Paper
正在生成论文摘要