Learning Control-Oriented Dynamical Structure from Data
arxiv(2023)
摘要
Even for known nonlinear dynamical systems, feedback controller synthesis is a difficult problem that often requires leveraging the particular structure of the dynamics to induce a stable closed-loop system. For general nonlinear models, including those fit to data, there may not be enough known structure to reliably synthesize a stabilizing feedback controller. In this paper, we propose a novel nonlinear tracking controller formulation based on a state-dependent Riccati equation for general nonlinear control-affine systems. Our formulation depends on a nonlinear factorization of the system of vector fields defining the control-affine dynamics, which we show always exists under mild smoothness assumptions. We discuss how this factorization can be learned from a finite set of data. On a variety of simulated nonlinear dynamical systems, we demonstrate the efficacy of learned versions of our controller in stable trajectory tracking. Alongside our method, we evaluate recent ideas in jointly learning a controller and stabilizability certificate for known dynamical systems; we show empirically that such methods can be data-inefficient in comparison.
更多查看译文
关键词
dynamical structure,learning,control-oriented
AI 理解论文
溯源树
样例
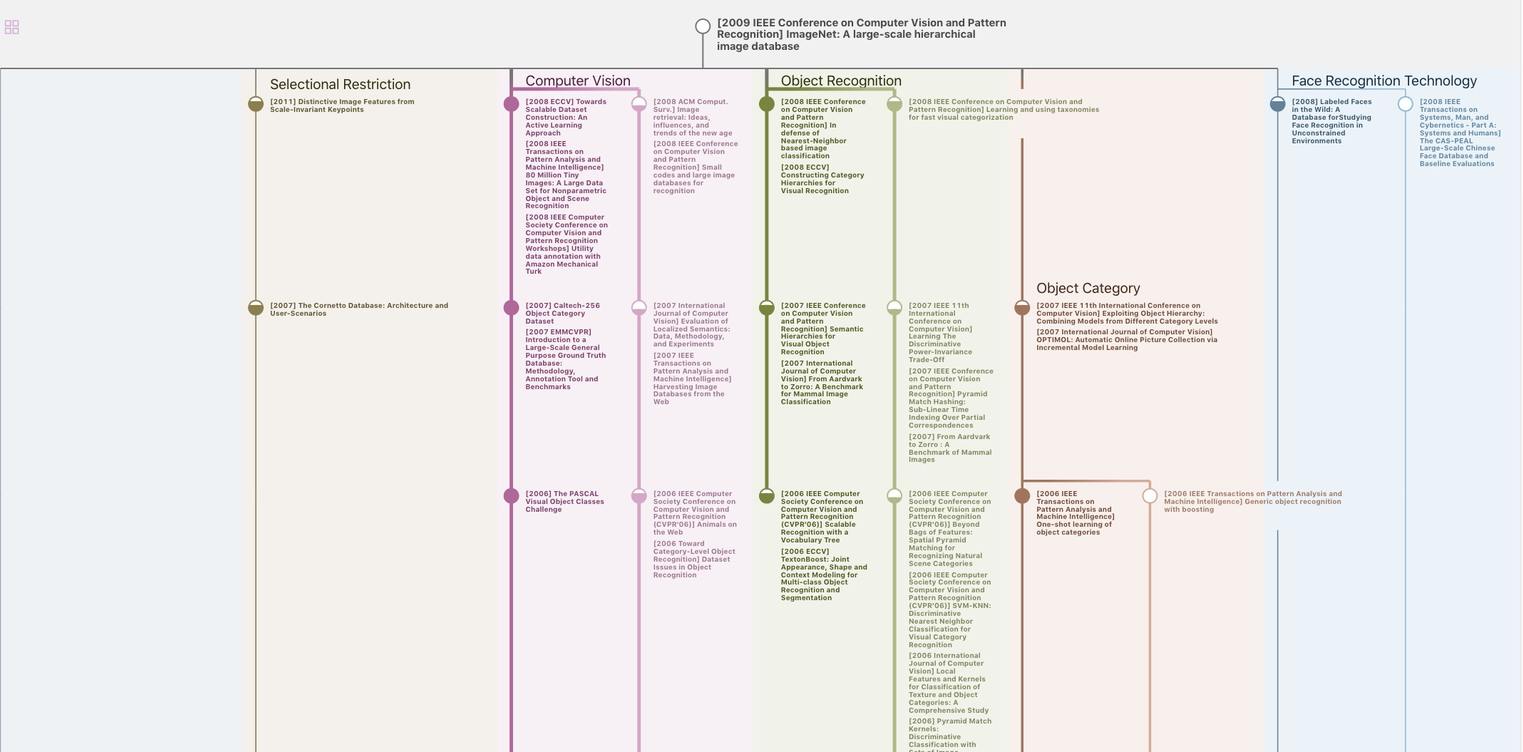
生成溯源树,研究论文发展脉络
Chat Paper
正在生成论文摘要