Robust Camera Pose Refinement for Multi-Resolution Hash Encoding
arxiv(2023)
摘要
Multi-resolution hash encoding has recently been proposed to reduce the computational cost of neural renderings, such as NeRF. This method requires accurate camera poses for the neural renderings of given scenes. However, contrary to previous methods jointly optimizing camera poses and 3D scenes, the naive gradient-based camera pose refinement method using multi-resolution hash encoding severely deteriorates performance. We propose a joint optimization algorithm to calibrate the camera pose and learn a geometric representation using efficient multi-resolution hash encoding. Showing that the oscillating gradient flows of hash encoding interfere with the registration of camera poses, our method addresses the issue by utilizing smooth interpolation weighting to stabilize the gradient oscillation for the ray samplings across hash grids. Moreover, the curriculum training procedure helps to learn the level-wise hash encoding, further increasing the pose refinement. Experiments on the novel-view synthesis datasets validate that our learning frameworks achieve state-of-the-art performance and rapid convergence of neural rendering, even when initial camera poses are unknown.
更多查看译文
关键词
robust camera pose refinement,multi-resolution
AI 理解论文
溯源树
样例
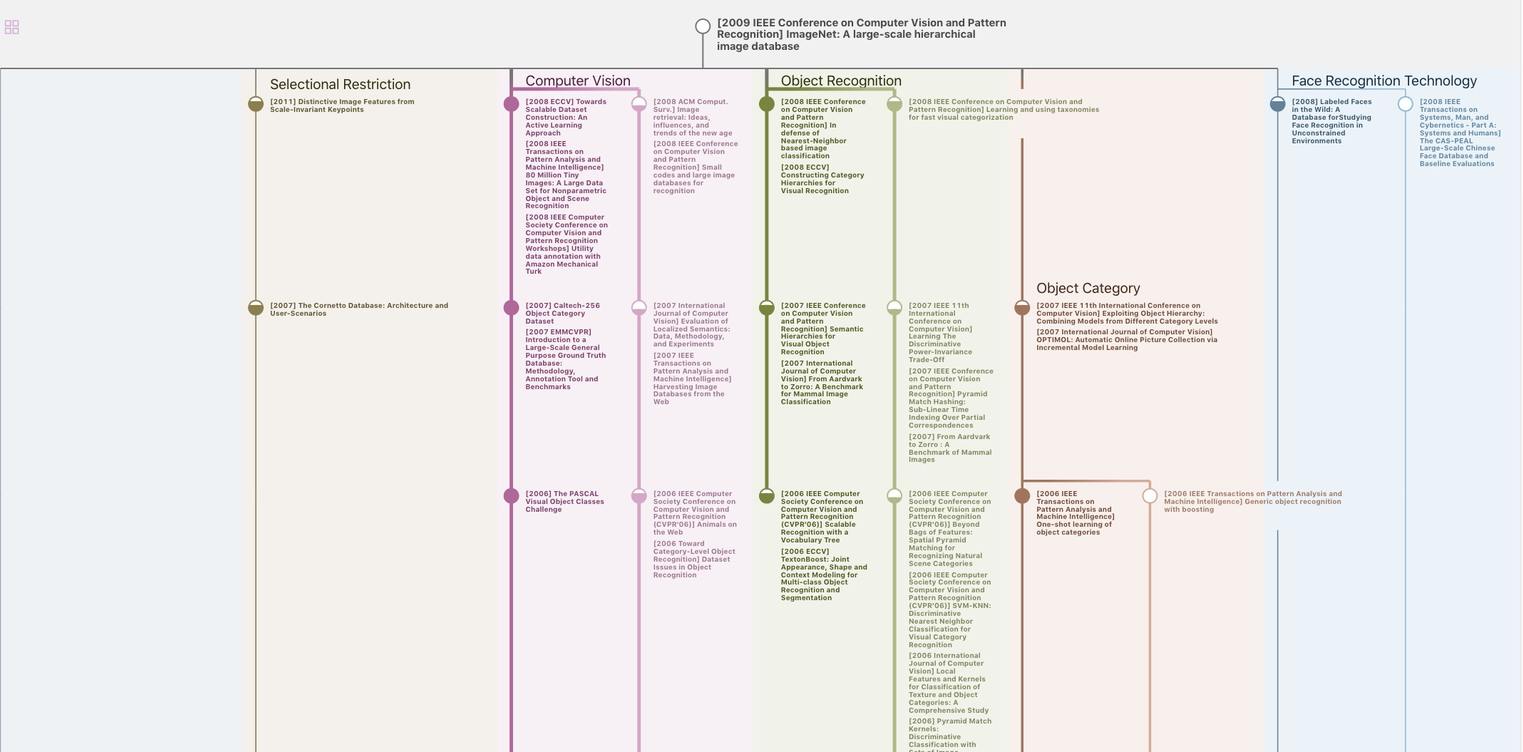
生成溯源树,研究论文发展脉络
Chat Paper
正在生成论文摘要