Recognition of grammatical classes of overt speech using electrophysiological signals and machine learning
2022 IEEE 4th International Conference on BioInspired Processing (BIP)(2022)
摘要
In this paper, we propose the use of overt speech in grammatical class classification using electroencephalography (EEG) and electromyography (EMG) signals as a first approach to attempt speech decoding in patients with bulbar amyotrophic lateral sclerosis (ALS). The work was carried out with 4 healthy participants, 32 EEG channels evenly distributed over the scalp and 4 EMG channels located in the zygomaticus major and triangular muscles were recorded during Spanish word production. Different classification algorithms, including a neural network, were evaluated in two groups of grammatical classes: adverbs vs. nouns. In EEG signals, 92.6±2.09% accuracy was achieved, while with EMG, 94.84±4.71%. These results show that it is possible to recognize grammatical classes in overt speech using EEG or EMG signals. The best performance in the EEG and EMG signals was obtained with the linear SVM classifier. Based on the classification accuracy of each class in each of the classification models, it is concluded that it is more convenient to use EMG signals in overt speech concerning EEG; however, if we want to decode the attempted speech in ALS patients using non-invasive methods, it is necessary to use the information provided by the EEG signals.
更多查看译文
关键词
Electroencephalogram (EEG),electromyogram (EMG),decoding speech,machine learning
AI 理解论文
溯源树
样例
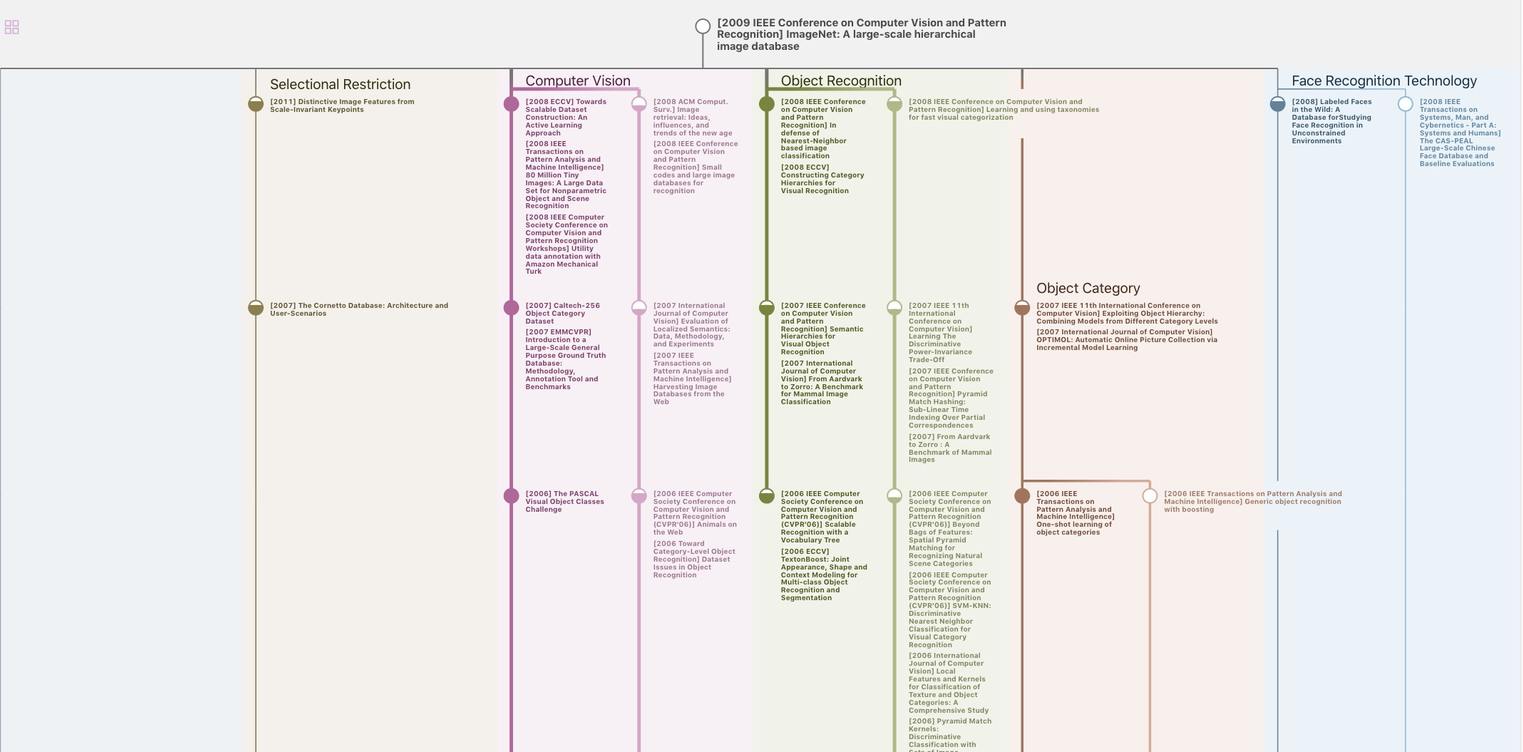
生成溯源树,研究论文发展脉络
Chat Paper
正在生成论文摘要