A Novel Data-Driven Emulator for Predicting Electromigration-Mediated Damage in Polycrystalline Interconnects
Journal of Electronic Materials(2023)
摘要
Electromigration (EM)-induced diffusional transport of metal atoms, which can manifest as the defects of grain boundary slits and voids in a metal line, often fail an entire electronic component. Formulating preventive strategies and their efficient implementation involves the analysis of failure mechanisms in 4D microstructures via tedious in situ x-ray tomography characterization as well as large-scale phase-field simulations, both of which are resource-intensive. Given this limitation, we report a data-driven emulation (DDE) technique, which couples machine learning with microstructure modeling, to enable a high-throughput and accurate prediction of grain boundary slit evolution in progressively degrading Cu interconnects under EM. In this context, the effectiveness of the stepwise linear regression approach which is the cornerstone of DDE has been quantified. We also analyze the importance of training dataset choice that significantly impacts the convergence between emulated and the phase-field simulated slit evolution dynamics. Finally, we also discuss the DDE-based insights related to the predominance of one or more descriptors in determining the slit evolution dynamics, which cannot be otherwise obtained directly from phase-field simulations.
更多查看译文
关键词
Machine learning, electromigration, interconnects, microstructure emulation
AI 理解论文
溯源树
样例
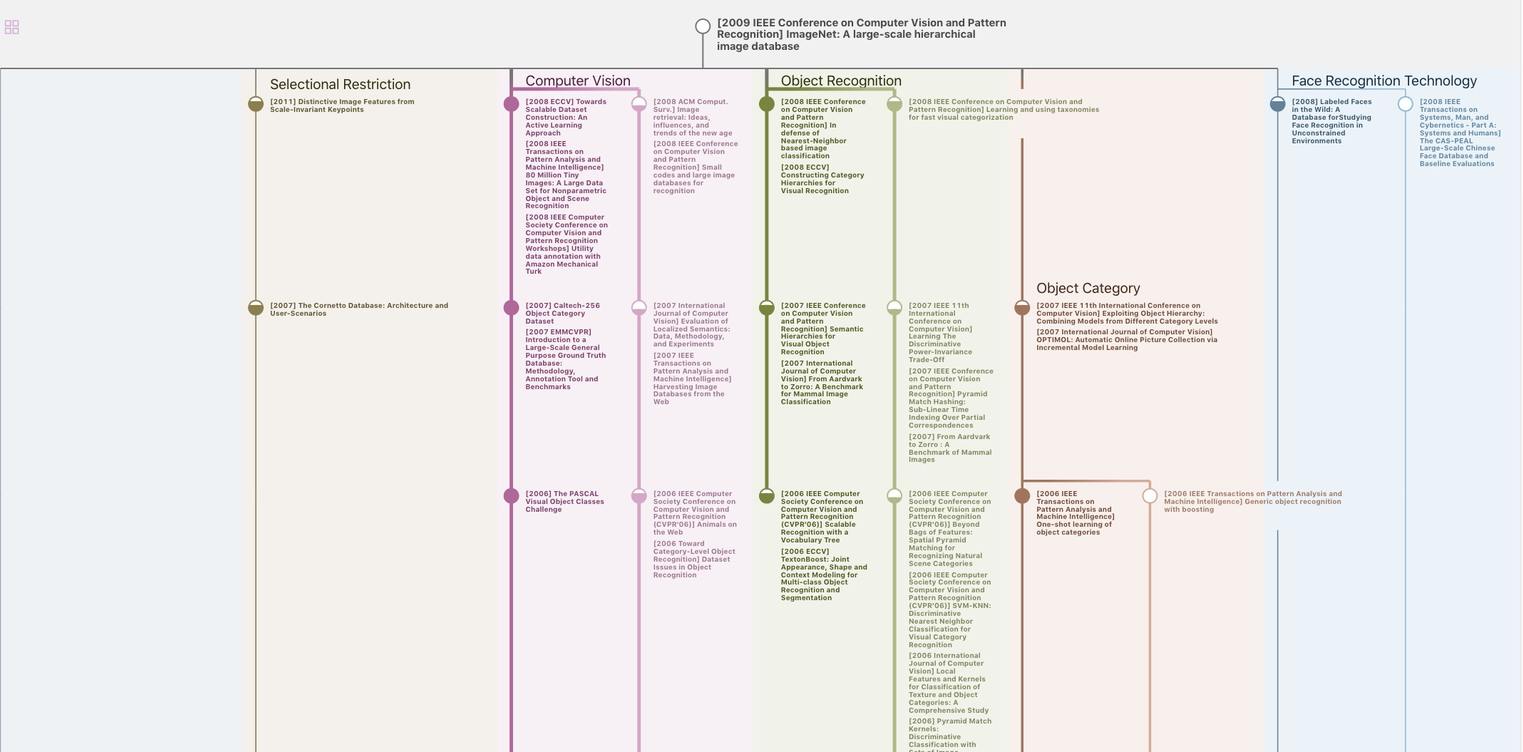
生成溯源树,研究论文发展脉络
Chat Paper
正在生成论文摘要