Function-Consistent Feature Distillation
ICLR 2023(2023)
摘要
As a commonly used technique in model compression of deep neural networks, feature distillation makes the student model mimic the intermediate features of the teacher model, in hopes that the underlying knowledge in the features could provide extra guidance to the student. Nearly all existing feature-distillation methods use L2 distance or its slight variants as the distance metric between teacher and student features. However, while L2 distance is isotropic w.r.t. all dimensions, the neural network’s operation on different dimensions is usually anisotropic, i.e., perturbations with the same 2-norm but in different dimensions of intermediate features lead to changes in the final output with largely different magnitude. Considering this, we argue that the similarity between teacher and student features should \textit{not} be measured merely based on their appearance (i.e. L2 distance), but should, more importantly, be measured by their difference in function, namely how the lateral parts of the network will read, decode, and process them. Therefore, we propose Function-Consistent Feature Distillation (FCFD), which explicitly optimizes the functional similarity between teacher and student features. The core idea of FCFD is to make teacher and student features not only numerically similar, but more importantly produce similar outputs when fed to the lateral part of the same network. With FCFD, the student mimics the teacher more faithfully and learns more from the teacher. Extensive experiments on image classification and object detection demonstrate the superiority of FCFD to existing methods. Furthermore, we can combine FCFD with many existing methods to obtain even higher accuracy. Codes will be publicly released soon.
更多查看译文
关键词
knowledge distillation,feature distillation,function consistency
AI 理解论文
溯源树
样例
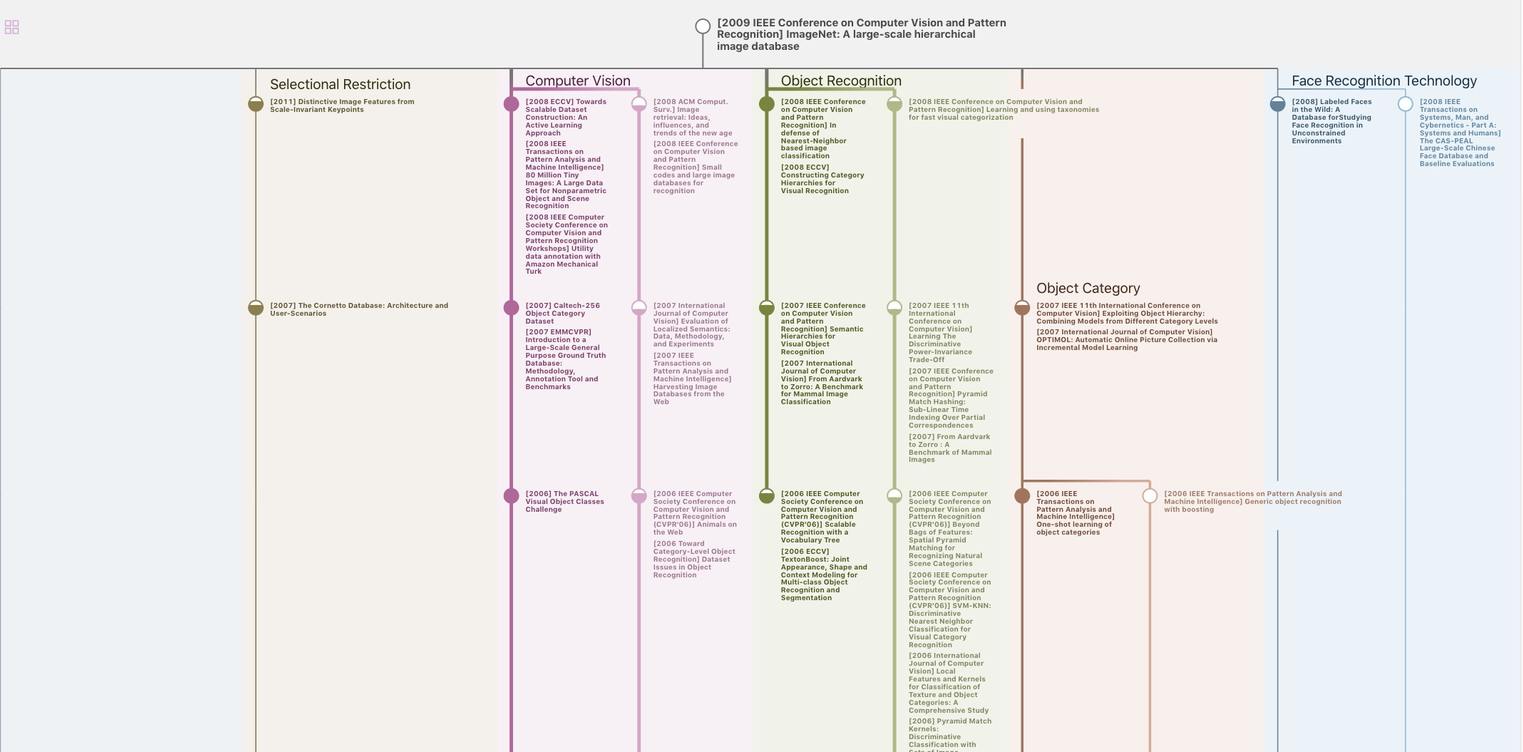
生成溯源树,研究论文发展脉络
Chat Paper
正在生成论文摘要