Bidirectional Propagation for Cross-Modal 3D Object Detection
ICLR 2023(2023)
摘要
Recent works have revealed the superiority of feature-level fusion for cross-modal 3D object detection, where fine-grained feature propagation from 2D image pixels to 3D LiDAR points has been widely adopted for performance improvement. Still, the potential of heterogeneous feature propagation between 2D and 3D domains has not been fully explored. In this paper, in contrast to existing pixel-to-point feature propagation, we investigate an opposite point-to-pixel direction, allowing point-wise features to flow inversely into the 2D image branch. Thus, when jointly optimizing the 2D and 3D streams, the gradients back-propagated from the 2D image branch can boost the representation ability of the 3D back-bone network working on LiDAR point clouds. Then, combining pixel-to-point and point-to-pixel information flow mechanisms, we further construct an interactive bidirectional feature propagation framework, dubbed BiProDet. In addition to the architectural design, we also propose normalized local coordinates map estimation, a new 2D auxiliary task for the training of the 2D image branch, which facilitates learning local spatial-aware features from the image modality and implicitly enhances the overall 3D detection performance. Extensive experiments and ablation studies validate the effectiveness of our method. Notably, we rank 1st on the highly competitive KITTI benchmark on the cyclist class by the time of submission. We also uploaded the source code in the supplementary material, which will be publicly available.
更多查看译文
关键词
Cross-modal,3D Object Detection,3D Point Cloud,Deep Learning
AI 理解论文
溯源树
样例
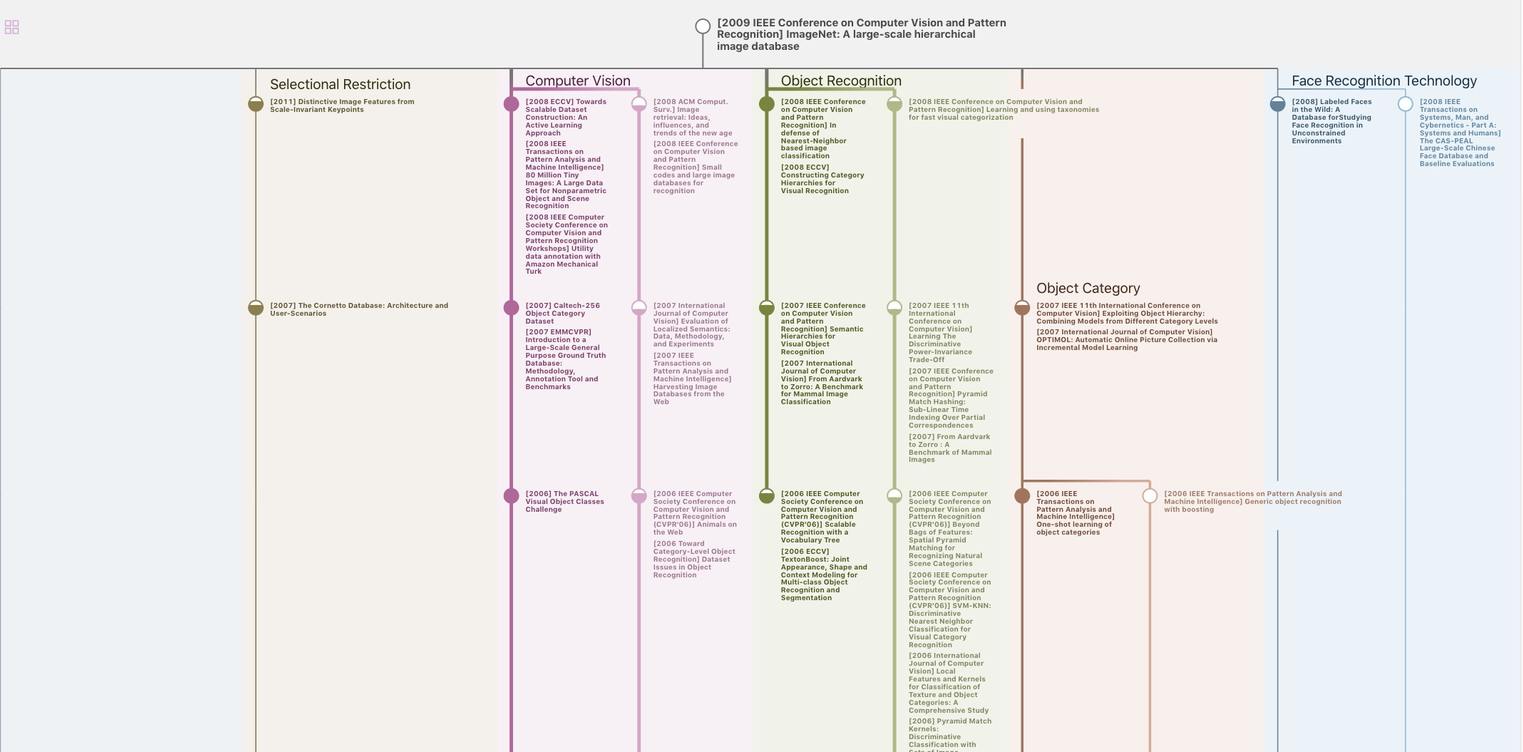
生成溯源树,研究论文发展脉络
Chat Paper
正在生成论文摘要