Human MotionFormer: Transferring Human Motions with Vision Transformers
ICLR 2023(2023)
摘要
We transfer motions from a target dynamic person to a source static one. An accurate matching between the source and the target human subjects improves the transferred motion quality. This matching paradigm shall be effective to capture both large and subtle motions changes between the target person and the source person, which are challenging for existing methods with CNNs. In this paper, we propose Human MotionFormer, a hierarchical ViT framework for motion transfer between two human subjects. Our MotionFormer leverages the local and the global perceptions (via convolutions and cross-attentions) to capture varying human motions. Specifically, our MotionFormer consists of two ViT encoders to extract input features (i.e., target pose image and a source human image), and a ViT decoder with several blocks for feature matching and motion transfer. The feature matching process is conducted in both motion warping and generation branches within each decoder block, where the target pose feature is set as Query and the source person feature is set as Key and Value. Then, the cross attention maps computed based on the Query, Key and Value are utilized for feature matching. Furthermore, we introduce a convolution layer to improve the local perception after the global cross attention computations. During model training, we propose a mutual learning loss to enable the co-supervision between motion warping and generation branches for consistent motion representations, which benefit the output transferred human motions. To this end, our MotionFormer leverages the local and global perceptions and introduces the mutual learning loss to improve transferred motion results. These designs empower our method to utilize only one source image for motion transfer and get rid of model finetuning. The experimental results qualitatively and quantitatively show that our Human MotionFormer sets the new state-of-the-art performance.
更多查看译文
AI 理解论文
溯源树
样例
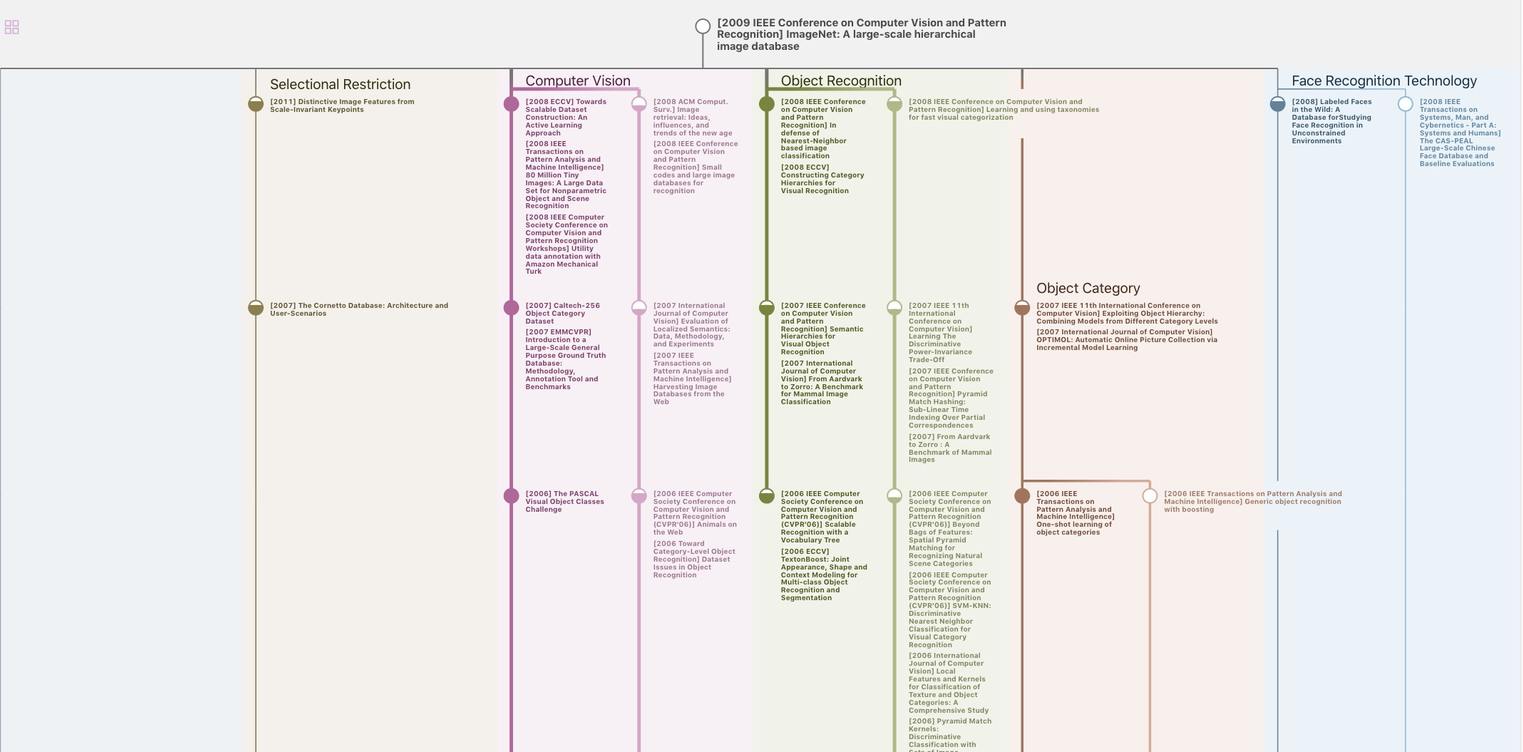
生成溯源树,研究论文发展脉络
Chat Paper
正在生成论文摘要