GAIN: On the Generalization of Instructional Action Understanding
ICLR 2023(2023)
摘要
Despite the great success achieved in instructional action understanding by deep learning and mountainous data, deploying trained models to the unseen environment still remains a great challenge, since it requires strong generalizability of models from in-distribution training data to out-of-distribution (OOD) data. In this paper, we introduce a benchmark, named GAIN, to analyze the GeneralizAbility of INstructional action understanding models. In GAIN, we reassemble steps of existing instructional video training datasets to construct the OOD tasks and then collect the corresponding videos. We evaluate the generalizability of models trained on in-distribution datasets with the performance on OOD videos and observe a significant performance drop. We further propose a simple yet effective approach, which cuts off the excessive contextual dependency of action steps by performing causal inference, to provide a potential direction for enhancing the OOD generalizability. In the experiments, we show that this simple approach can improve several baselines on both instructional action segmentation and detection tasks. We expect the introduction of the GAIN dataset will promote future in-depth research on the generalization of instructional video understanding.
更多查看译文
关键词
Action Analysis,Instructional Video,OOD Generalization
AI 理解论文
溯源树
样例
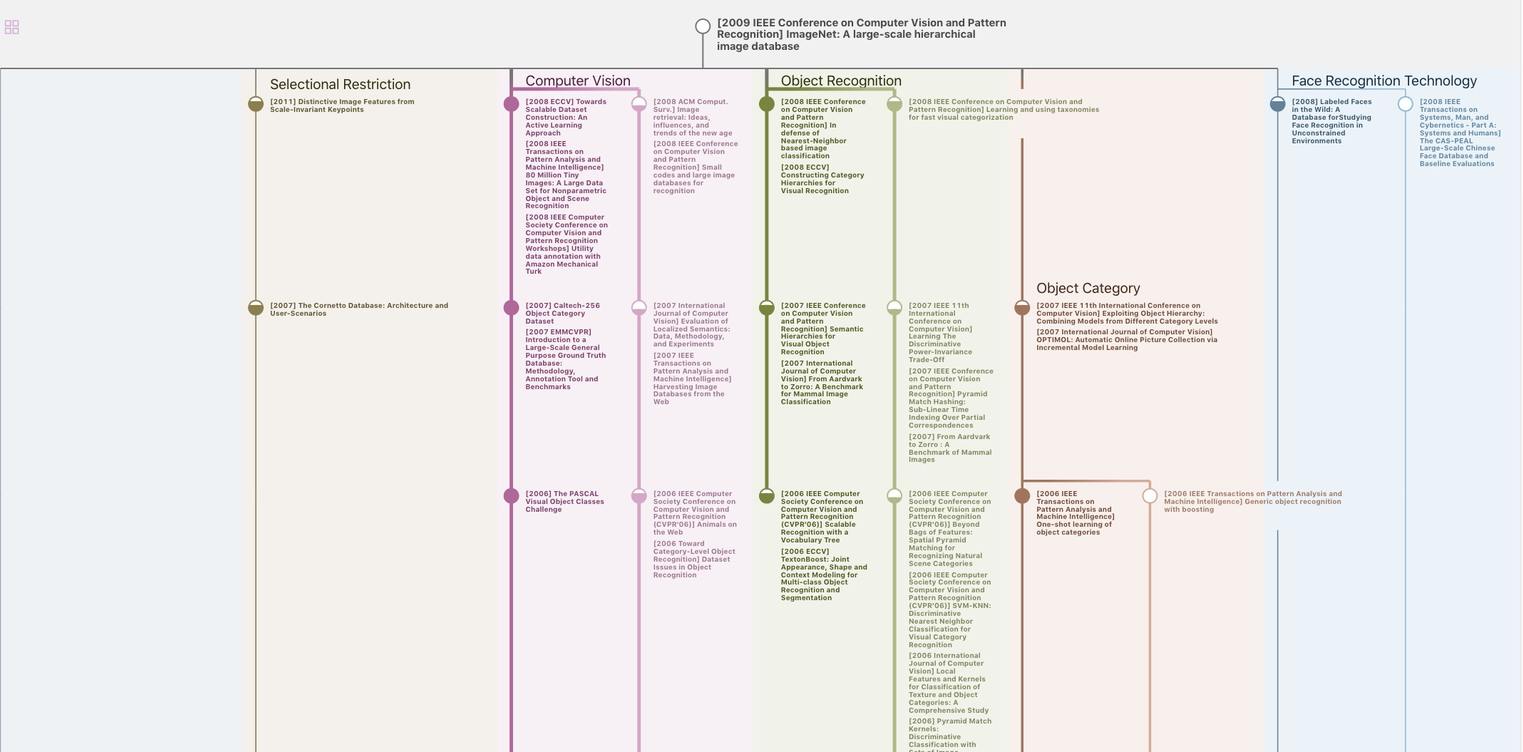
生成溯源树,研究论文发展脉络
Chat Paper
正在生成论文摘要