Contrastive Alignment of Vision to Language Through Parameter-Efficient Transfer Learning
ICLR 2023(2023)
摘要
The creation of contrastive vision-language models has traditionally required aligning a vision model with a language model by updating all of their parameters through gradient descent. It is not known if contrastive vision-language models (e.g. CLIP) can be created by a small number of parameter updates to already-trained language and vision models. The literature describes techniques that can create vision-language models by updating a small number of parameters in a language model, but these require already aligned visual representations and are non-contrastive, hence unusable for latency-sensitive applications such as neural search. We explore the feasibility and benefits of parameter-efficient contrastive vision-language alignment through transfer learning: creating a model such as CLIP by minimally updating an already-trained vision and language model. We find that a minimal set of parameter updates (<7\%) can achieve the same performance as full-model training, and updating specific components (<1\% of parameters) can match 75\% of full-model training. We describe a series of experiments: we show that existing knowledge is conserved more strongly in parameter-efficient training and that parameter-efficient scaling scales with model and dataset size. We show evidence of an intriguing asymmetry in the vision and language models, and how it affects alignment. Where paired-image text data is scarce but strong multilingual language models exist (e.g. low resource languages), parameter-efficient training is even preferable to full-model training. Given a fixed compute budget, parameter-efficient training allows training larger models on the same hardware, achieving equivalent performance in less time. Parameter-efficient training hence constitutes an energy-efficient and effective training strategy for contrastive vision-language models that may be preferable to the current full-model training paradigm for common use cases.
更多查看译文
关键词
vision-language,CLIP,image-text retrieval,transformers
AI 理解论文
溯源树
样例
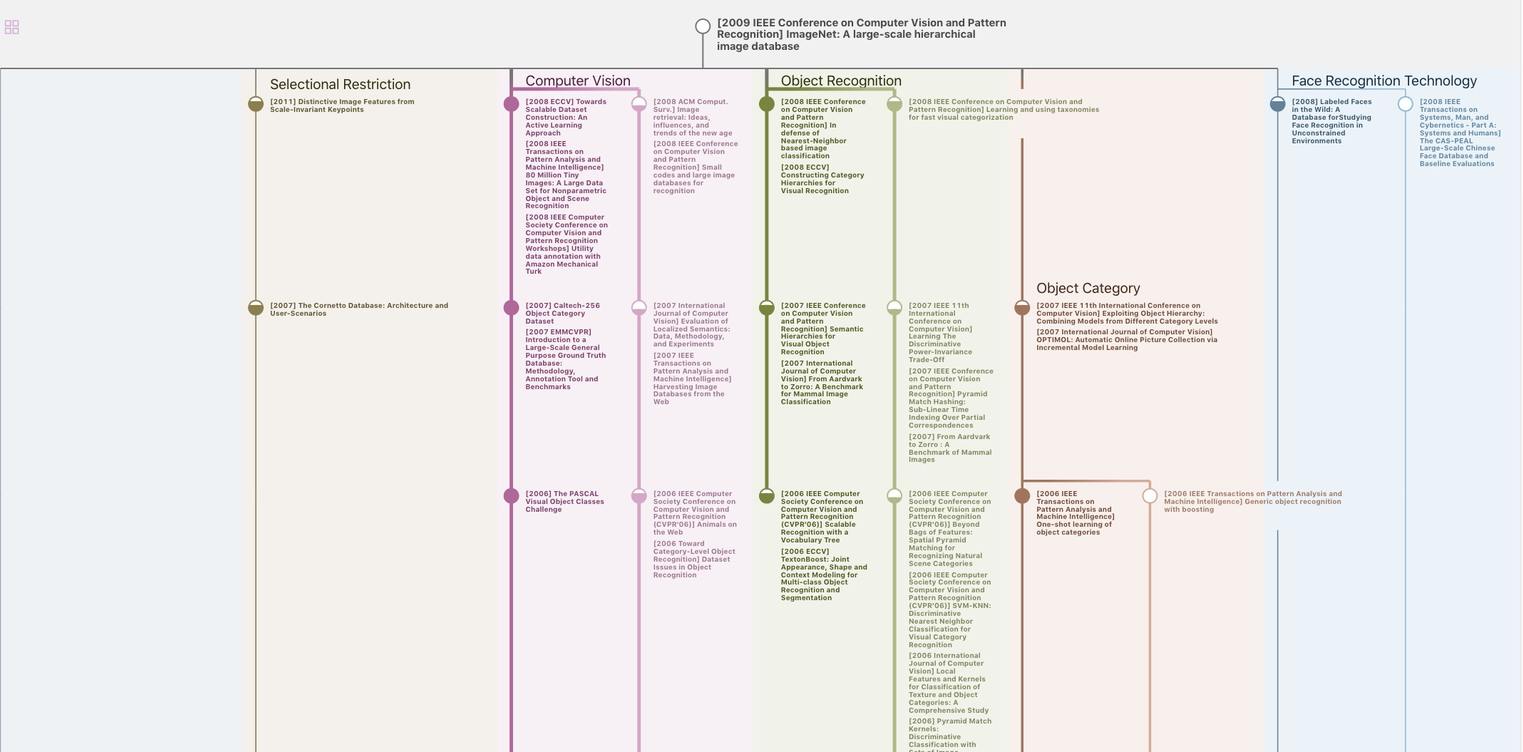
生成溯源树,研究论文发展脉络
Chat Paper
正在生成论文摘要