RoPAWS: Robust Semi-supervised Representation Learning from Uncurated Data
ICLR 2023(2023)
摘要
Semi-supervised learning aims to train a model using limited labels. State-of-the-art semi-supervised methods for image classification such as PAWS rely on self-supervised representations learned with large-scale unlabeled but curated data. However, PAWS is often less effective when using real-world unlabeled data that is uncurated, e.g., contains out-of-class data. We propose RoPAWS, a robust extension of PAWS that can work with real-world unlabeled data. We first reinterpret PAWS as a generative classifier that models densities using kernel density estimation. From this probabilistic perspective, we calibrate its prediction based on the densities of labeled and unlabeled data, which leads to a simple closed-form solution from the Bayes' rule. We demonstrate that RoPAWS significantly improves PAWS for uncurated Semi-iNat by +5.3% and curated ImageNet by +0.4%.
更多查看译文
关键词
semi-supervised learning,representation learning,uncurated data
AI 理解论文
溯源树
样例
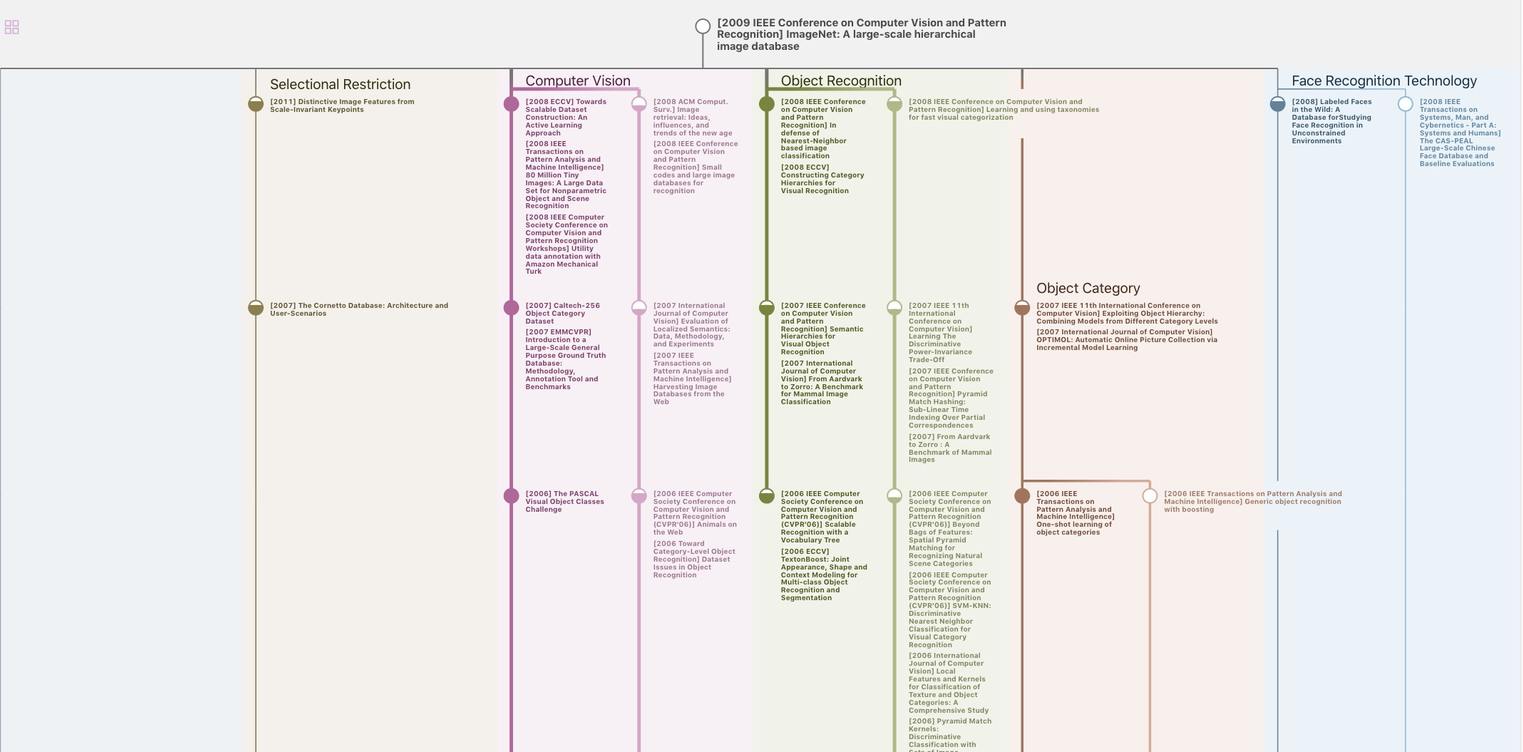
生成溯源树,研究论文发展脉络
Chat Paper
正在生成论文摘要