Exponential Generalization Bounds with Near-Optimal Rates for $L_q$-Stable Algorithms.
ICLR 2023(2023)
摘要
The \emph{stability} of learning algorithms to changes in the training sample has been actively studied as a powerful proxy for reasoning about generalization. Recently, exponential tail generalization and risk bounds with near-optimal rates have been obtained under the stringent and distribution-free notion of uniform stability~\citep{bousquet2020sharper,klochkov2021stability}. In the meanwhile, under the notion of $L_q$-stability, which is weaker and distribution dependent, exponential generalization bounds are also available yet so far only with sub-optimal rates. Therefore, a natural question we would like to address in this paper is whether it is possible to derive near-optimal exponential generalization bounds for $L_q$-stable learning algorithms. As the core contribution of the present work, we give an affirmative answer to this question by developing strict analogues of the near-optimal generalization and risk bounds of uniformly stable algorithms for $L_q$-stable algorithms. We demonstrate the power of our improved bounds by applying them to derive strong sparse excess risk bounds, under mild conditions, for computationally tractable sparsity estimation algorithms such as Iterative Hard Thresholding (IHT).
更多查看译文
关键词
$L_q$-stability,Uniform stability,Moments inequality,Exponential generalization bound,Excess risk,Sparsity
AI 理解论文
溯源树
样例
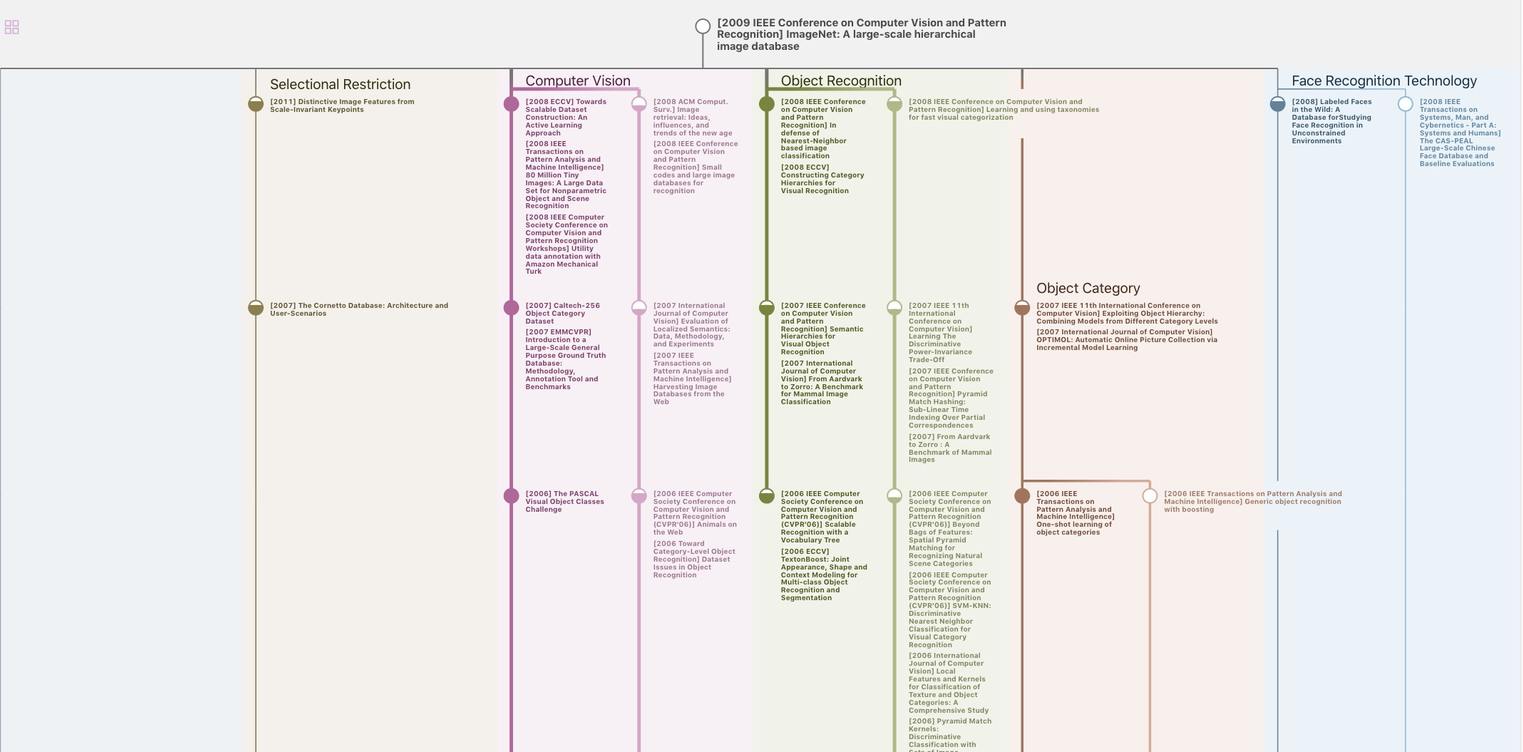
生成溯源树,研究论文发展脉络
Chat Paper
正在生成论文摘要