Interpretable Debiasing of Vectorized Language Representations with Iterative Orthogonalization
ICLR 2023(2023)
摘要
We propose a new mechanism to augment a word vector embedding representation that offers improved bias removal while retaining the key information—resulting in improved interpretability of the representation. Rather than removing the information associated with a concept that may induce bias, our proposed method identifies two concept subspaces and makes them orthogonal. The resulting representation has these two concepts uncorrelated. Moreover, because they are orthogonal, one can simply apply a rotation on the basis of the representation so that the resulting subspace corresponds with coordinates. This explicit encoding of concepts to coordinates works because they have been made fully orthogonal, which previous approaches do not achieve. Furthermore, we show that this can be extended to multiple subspaces. As a result, one can choose a subset of concepts to be represented transparently and explicitly, while the others are retained in the mixed but extremely expressive format of the representation.
更多查看译文
关键词
bias,fairness,ethics,debiasing,static embeddings,pre-trained contextualized embeddings,natural language processing
AI 理解论文
溯源树
样例
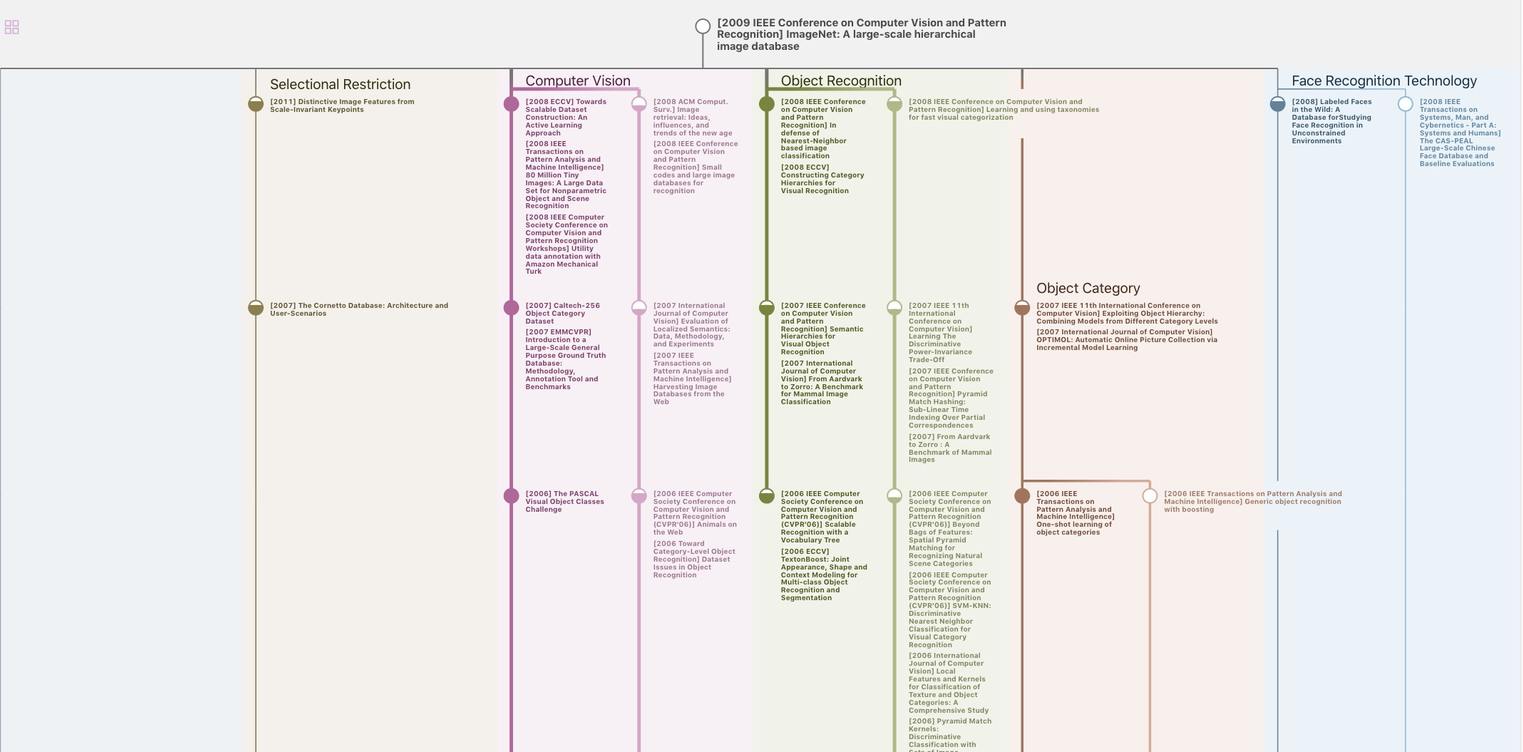
生成溯源树,研究论文发展脉络
Chat Paper
正在生成论文摘要