Neural Implicit Shape Editing using Boundary Sensitivity
ICLR 2023(2023)
摘要
Neural fields are receiving increased attention as a geometric representation due to their ability to compactly store detailed and smooth shapes and easily undergo topological changes.
Compared to classic geometry representations, however, neural representations do not allow the user to exert intuitive control over the shape.
Motivated by this, we leverage \emph{boundary sensitivity} to express how perturbations in parameters move the shape boundary.
This allows to interpret the effect of each learnable parameter and study achievable deformations.
With this, we perform \emph{geometric editing}: finding a parameter update which best approximates a globally prescribed deformation.
Prescribing the deformation only locally allows to deform the rest of the shape according to some prior, such as \emph{semantics} or \emph{deformation} rigidity.
Different to previous efforts, our method is model-agnostic and can be applied to a pre-trained NN and update it in-place.
Furthermore, we show how boundary sensitivity helps optimize and constrain objectives (such as surface area and volume), which are difficult to compute without first converting to another representation, such as a mesh.
更多查看译文
关键词
shape,sensitivity
AI 理解论文
溯源树
样例
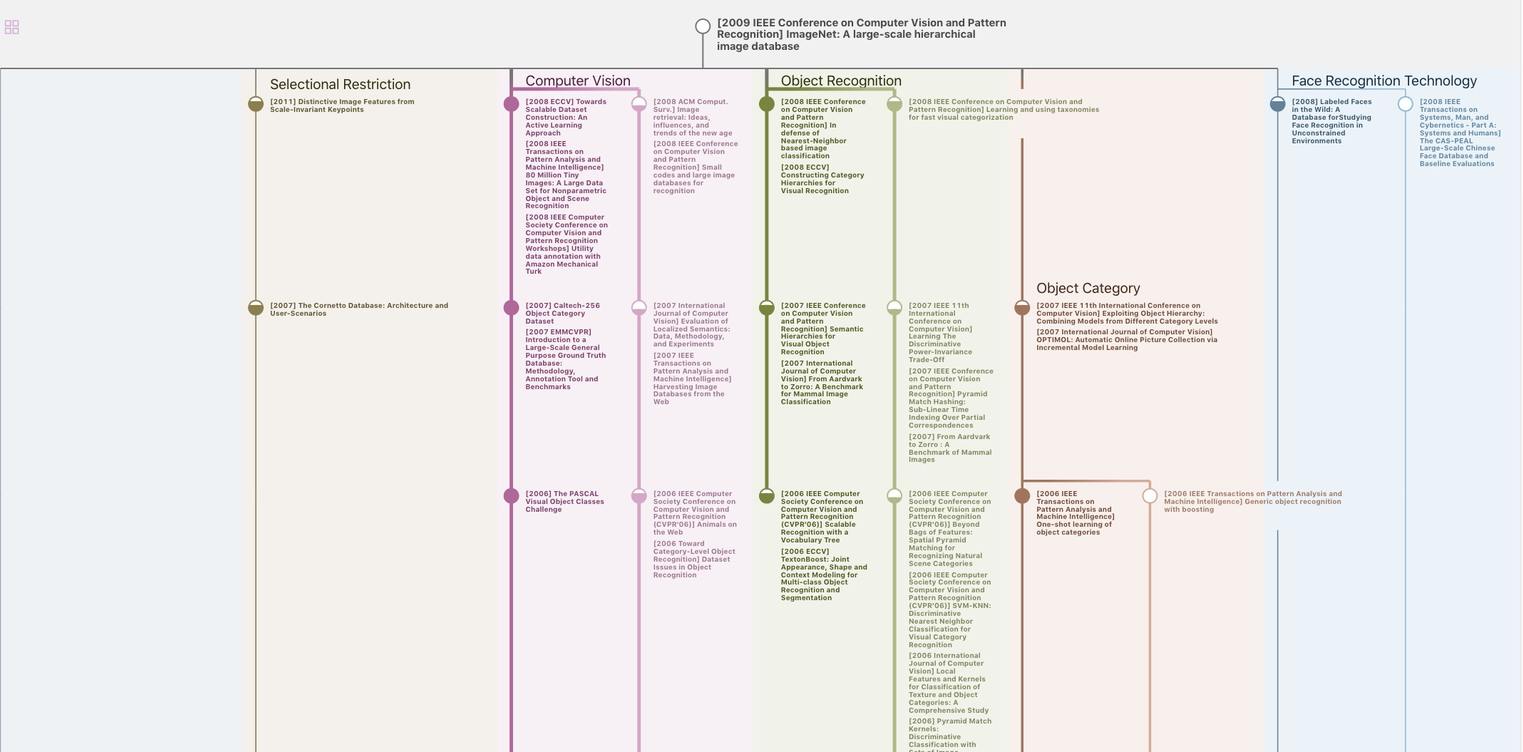
生成溯源树,研究论文发展脉络
Chat Paper
正在生成论文摘要