On The Specialization of Neural Modules
ICLR 2023(2023)
摘要
A number of machine learning models have been proposed with the goal of achieving systematic generalization: the ability to reason about new situations by combining aspects of previous experiences. These models leverage compositional architectures which aim to learn specialized modules dedicated to structures in a task that can be composed to solve novel problems with similar structures. While the compositionality of these architectures is guaranteed by design, the modules specializing is not. Here we theoretically study the ability of network modules to specialize to useful structures in a dataset and achieve systematic generalization. To this end we introduce a minimal space of datasets motivated by practical systematic generalization benchmarks. From this space of datasets we present a mathematical definition of systematicity and study the learning dynamics of linear neural modules when solving components of the task. Our results shed light on the difficulty of module specialization, what is required for modules to successfully specialize, and the necessity of modular architectures to achieve systematicity. Finally, we confirm that the theoretical results in our tractable setting generalize to more complex datasets and non-linear architectures.
更多查看译文
关键词
Systematic Generalization,Linear Neural Networks,Neural Module Networks
AI 理解论文
溯源树
样例
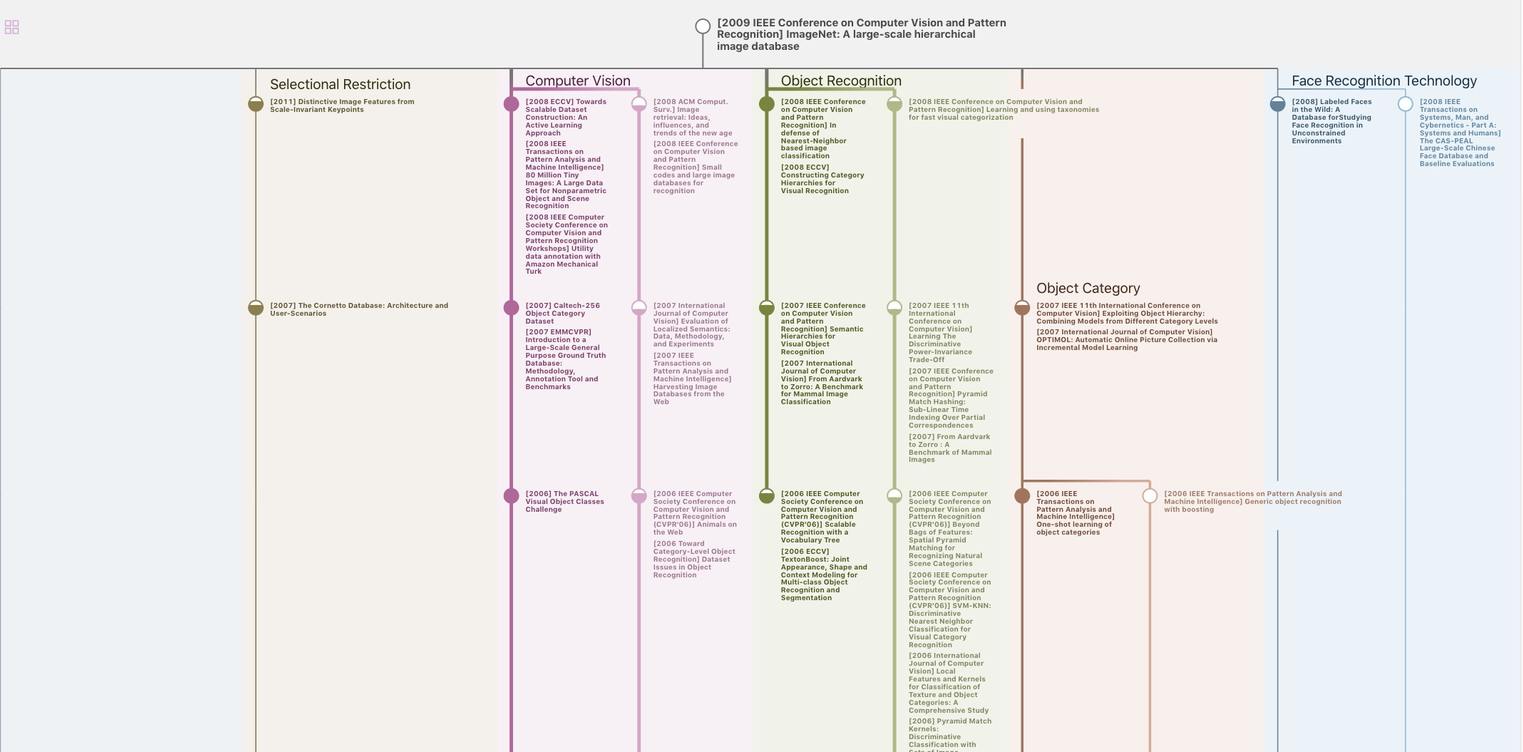
生成溯源树,研究论文发展脉络
Chat Paper
正在生成论文摘要