Denoising Diffusion Samplers
ICLR 2023(2023)
摘要
Denoising diffusion models are a popular class of generative models providing state-of-the-art results in many domains. One adds gradually noise to data using a diffusion to transform the data distribution into a Gaussian distribution. Samples from the generative model are then obtained by simulating an approximation of the time-reversal of this diffusion initialized by Gaussian samples. Practically, the intractable score terms appearing in the time-reversed process are approximated using score matching techniques. We explore here a similar idea to sample approximately from unnormalized probability density functions and estimate their normalizing constants. We consider a process where the target density diffuses towards a Gaussian. Denoising Diffusion Samplers (DDS) are obtained by approximating the corresponding time-reversal. While score matching is not applicable in this context, we can leverage many of the ideas introduced in generative modeling for Monte Carlo sampling. Existing theoretical results from denoising diffusion models also provide theoretical guarantees for DDS. We discuss the connections between DDS, optimal control and Schr\"odinger bridges and finally demonstrate DDS experimentally on a variety of challenging sampling tasks.
更多查看译文
关键词
diffusion models,importance sampling,monte carlo,variational inference
AI 理解论文
溯源树
样例
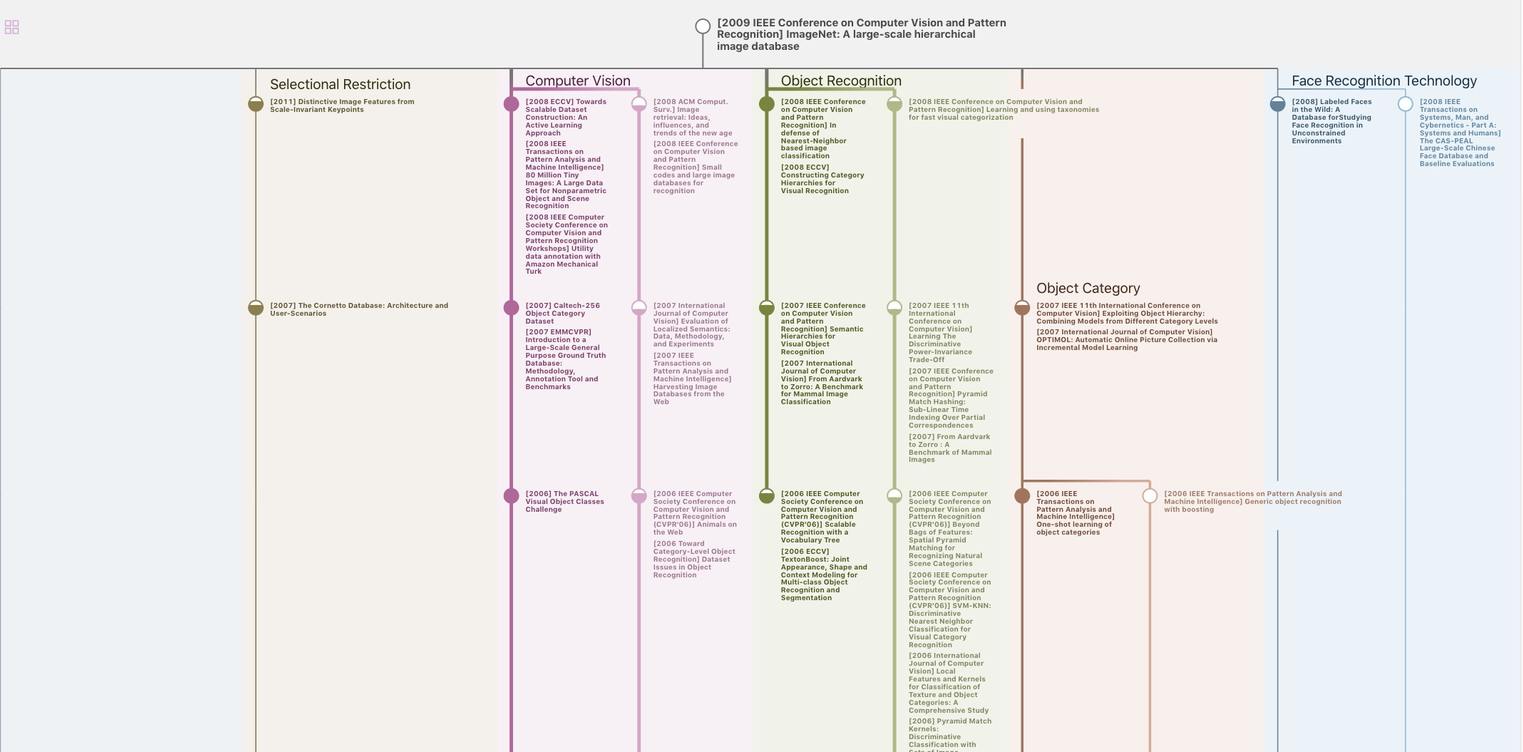
生成溯源树,研究论文发展脉络
Chat Paper
正在生成论文摘要