Sparse and Hierarchical Masked Modeling for Convolutional Representation Learning
ICLR 2023(2023)
摘要
This paper presents a simple yet powerful framework to pre-train convolutional network (convnet) with Sparse masKed modeling. SparK addresses key challenges in applying transformer-specialized masked modeling to convolutional models: (i) convolution operation cannot handle irregular, random-masked input; (ii) the single-scale nature of existing masked modeling is inconsistent with convnet's hierarchical structure. For (i), we sparsely gather the unmasked pixels to a sparse image and use sparse convolution for encoding. For the later, we develop a hierarchical encoder-decoder to reconstruct from multi-scale encoded features to fully exploit the advantage of hierarchy. As the first hierarchical masked modeling method designed for convnets, SparK exploits their untapped potential. On three downstream tasks, SparK surpasses both state-of-the-art contrastive learning and \textit{transformer-based} masked modeling by similarly large margins (around +1.0%). Improvements on object detection and instance segmentation are more substantial (>1.0%), verifying strong transferability of features learned by SparK. We also demonstrate SparK's favorable scaling behavior by observing more gains on larger models. Taken all results together, a promising future of generative pre-training on convnets has been initially shown by SparK. Codes will be made publicly available.
更多查看译文
关键词
Self-Supervised Learning,Masked Autoencoding,Masked Pre-training,Masked Modeling,Convolutional Neural Networks
AI 理解论文
溯源树
样例
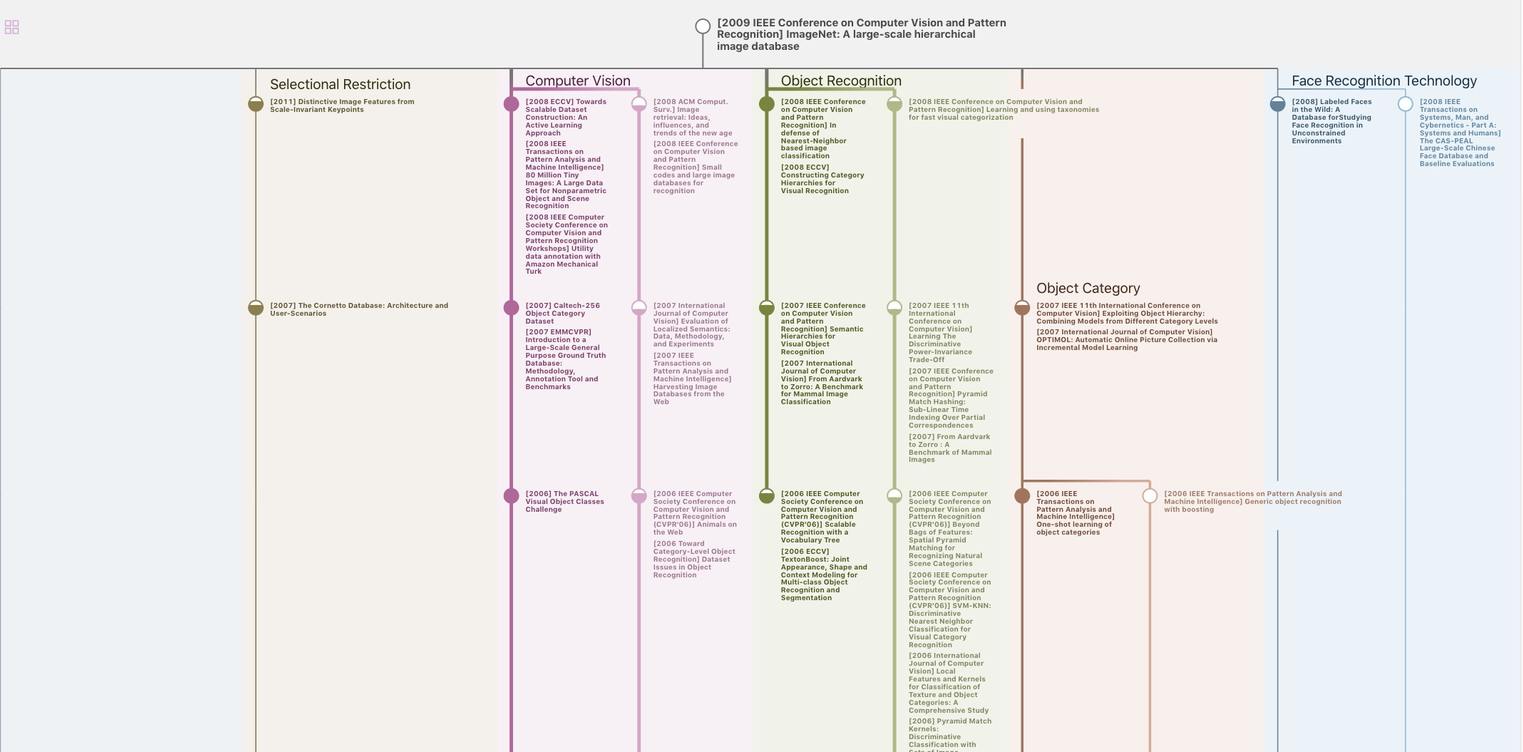
生成溯源树,研究论文发展脉络
Chat Paper
正在生成论文摘要