Planning Goals for Exploration
ICLR 2023(2023)
摘要
Dropped into an unknown environment, what should an agent do to quickly learn about the environment and how to accomplish diverse tasks within it? We address this question within the goal-conditioned reinforcement learning paradigm, by identifying how the agent should set its goals at training time to maximize exploration. We propose "planning exploratory goals" (PEG), a method that sets goals for each training episode to directly optimize an intrinsic exploration reward. PEG first chooses goal commands such that the agent's goal-conditioned policy, at its current level of training, will end up in states with high exploration potential. It then launches an exploration policy starting at those promising states. To enable this direct optimization, PEG learns world models and adapts sampling-based planning algorithms to "plan goal commands". In challenging simulated robotics environments including a multi-legged ant robot in a maze, and a robot arm on a cluttered tabletop, PEG exploration enables more efficient and effective training of goal-conditioned policies relative to baselines and ablations. Our ant successfully navigates a long maze, and the robot arm successfully builds a stack of three blocks upon command. Project website: https://sites.google.com/view/exploratory-goals
更多查看译文
关键词
model-based reinforcement learning,exploration,goal-conditioned reinforcement learning,planning,intrinsic motivation,reinforcement learning
AI 理解论文
溯源树
样例
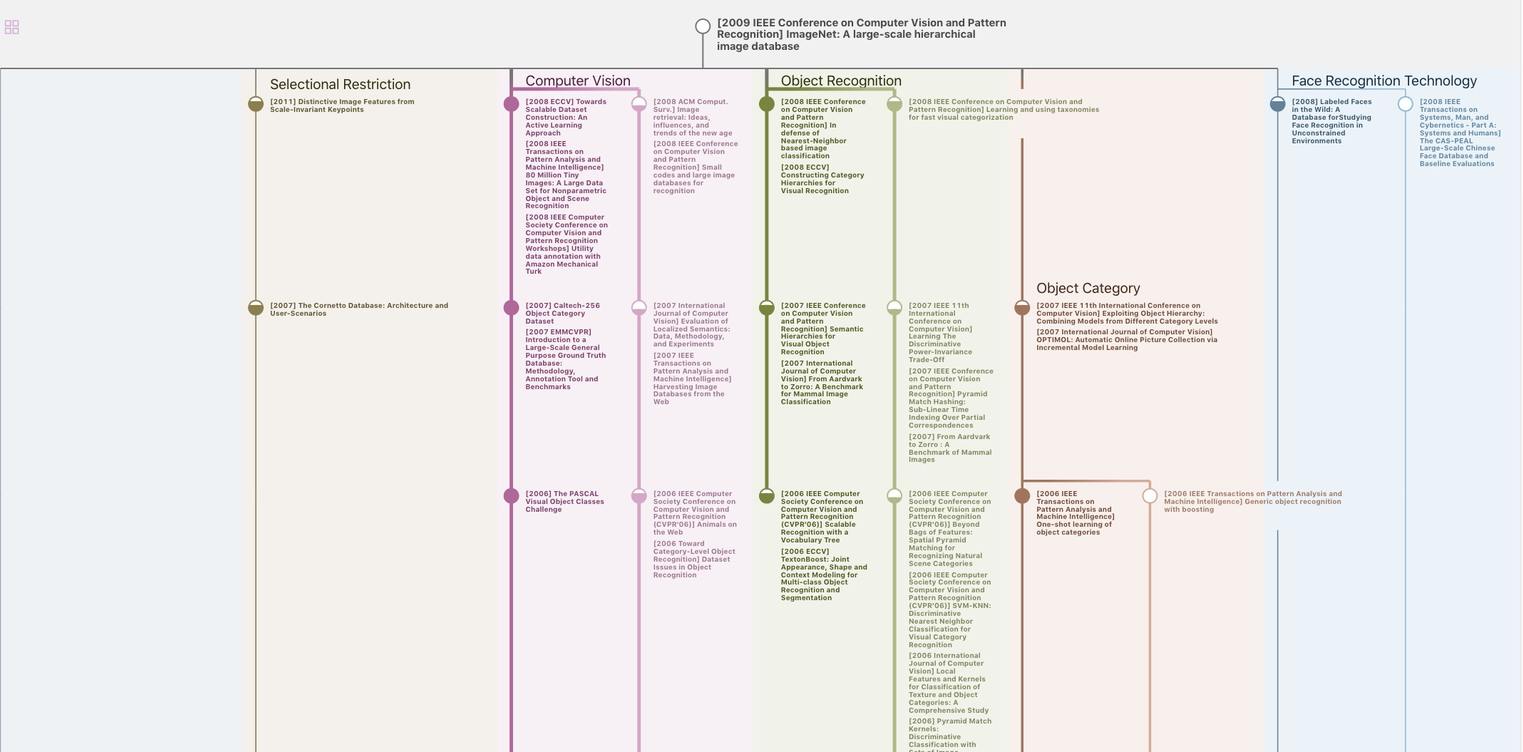
生成溯源树,研究论文发展脉络
Chat Paper
正在生成论文摘要