Capturing the Motion of Every Joint: 3D Human Pose and Shape Estimation with Independent Tokens
ICLR 2023(2023)
摘要
In this paper we present a novel method to estimate 3D human pose and shape from monocular videos. This task requires directly recovering pixel-alignment 3D human pose and body shape from monocular images or videos, which is challenging due to its inherent ambiguity. To improve precision, existing methods highly rely on the initialized mean pose and shape as prior estimates and parameter regression with an iterative error feedback manner. In addition, video-based approaches model the overall changes over the image-level features to temporally enhance the single-frame feature, but fail to capture the rotational motion at the joint level, and cannot guarantee local temporal consistency. To address these issues, we propose a novel Transformer-based model with a design of independent tokens. First, we introduce three types of tokens independent of the image feature: \textit{joint rotation tokens, shape token, and camera token}.
By progressively interacting with image features through Transformer layers, these tokens learn to encode 3D rotations of human joints, body shape, and position information, while adapting to a given image and learning priors between joint rotation angles from large-scale data. Second, benefiting from the proposed token-based representation, we further use a temporal model to focus on capturing the rotational temporal information of each joint, which is empirically conducive to preventing large jitters in local parts. Despite being conceptually simple, the proposed method attains superior performances on the 3DPW and Human3.6M datasets. Using ResNet-50 and Transformer architectures, it achieves 42.0 mm error on the PA-MPJPE metric of the challenging 3DPW, outperforming state-of-the-art counterparts by a large margin.
更多查看译文
关键词
3D human pose and shape estimation,3d human reconstruction,transformer,independent tokens,temporal modeling,joint rotational motion
AI 理解论文
溯源树
样例
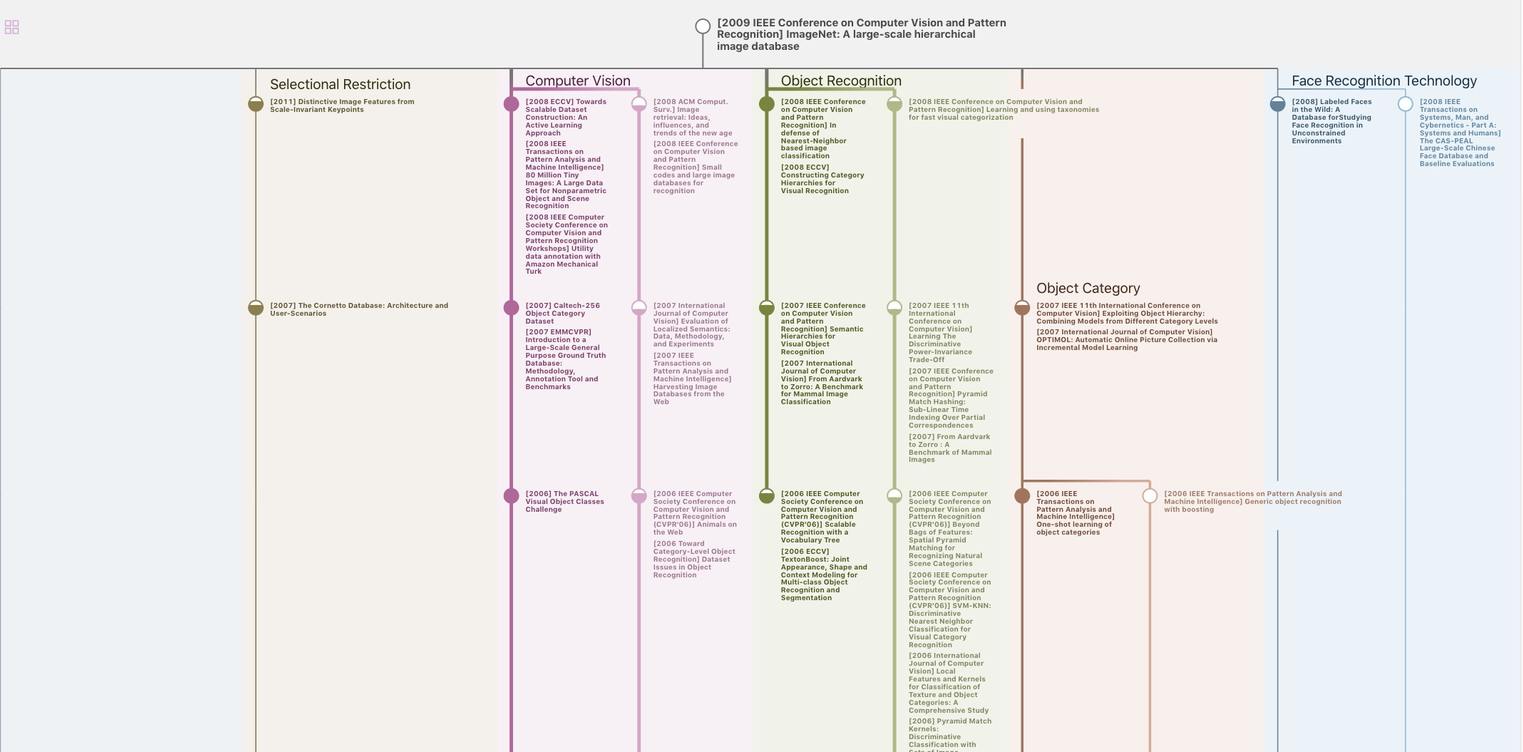
生成溯源树,研究论文发展脉络
Chat Paper
正在生成论文摘要