Towards Interpretable Deep Reinforcement Learning with Human-Friendly Prototypes
ICLR 2023(2023)
摘要
Despite recent success of deep learning models in research settings, their application in sensitive domains remains limited because of their opaque decision-making processes. Taking to this challenge, people have proposed various eXplainable AI (XAI) techniques designed to calibrate trust and understandability of black-box models, with the vast majority of work focused on supervised learning. Here, we focus on making an "interpretable-by-design" deep reinforcement learning agent which is forced to use human-friendly prototypes in its decisions, thus making its reasoning process clear. Our proposed method, dubbed Prototype-Wrapper Network (PW-Net), wraps around any neural agent backbone, and results indicate that it does not worsen performance relative to black-box models. Most importantly, we found in a user study that PW-Nets supported better trust calibration and task performance relative to standard interpretability approaches and black-boxes.
更多查看译文
关键词
Interpretable Machine Learning,Deep Reinforcement Learning,Prototypes,User Study
AI 理解论文
溯源树
样例
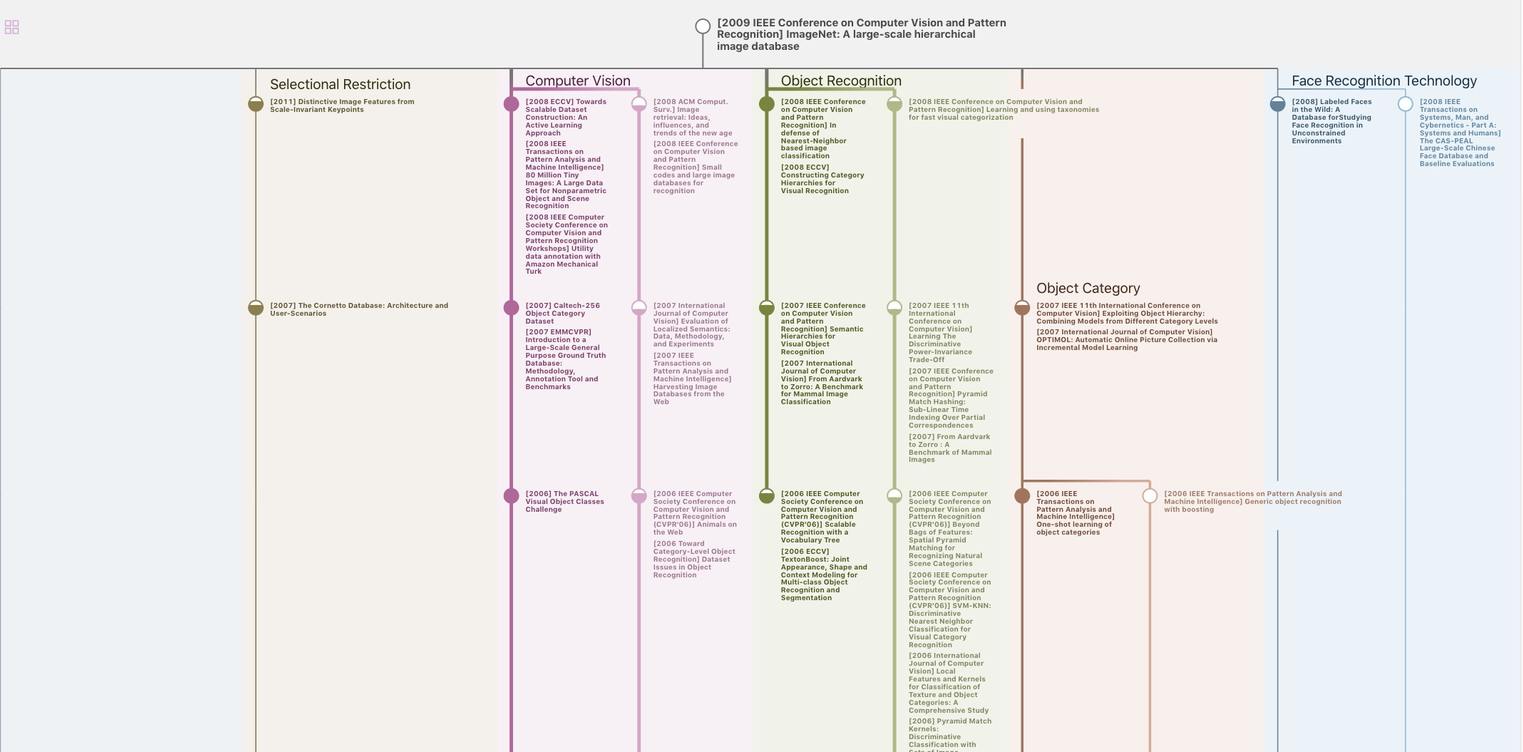
生成溯源树,研究论文发展脉络
Chat Paper
正在生成论文摘要