A Large-Scale Ensemble Learning Framework for Demand Forecasting
2022 IEEE International Conference on Data Mining (ICDM)(2022)
摘要
Demand forecasting is a crucial component of supply chain management for revenue optimization and inventory planning. Traditional time series forecasting methods, however, have resulted in small models with limited expressive power because they have difficulty in scaling their model size up while maintaining high accuracy. In this paper, we propose Forecasting orchestra (Forchestra), a simple but powerful ensemble framework capable of accurately predicting future demand for a diverse range of items. Forchestra consists of two parts: 1) base predictors and 2) a neural conductor. For a given time series, each base predictor outputs its respective forecast based on historical observations. On top of the base predictors, the neural conductor adaptively assigns the importance weight for each predictor by looking at the representation vector provided by a representation module. Finally, Forchestra aggregates the predictions by the weights and constructs a final prediction. In contrast to previous ensemble approaches, the neural conductor and all base predictors of Forchestra are trained in an end-to-end manner; this allows each base predictor to modify its reaction to different inputs, while supporting other predictors and constructing a final prediction jointly. We empirically show that the model size is scalable to up to 0.8 billion parameters ($\approx$400-layer LSTM). The proposed method is evaluated on our proprietary E-Commerce (100K) and the public M5(30K) datasets, and it outperforms existing forecasting models with a significant margin. In addition, we observe that our framework generalizes well to unseen data points when evaluated in a zeroshot fashion on downstream datasets. Last but not least, we present extensive qualitative and quantitative studies to analyze how the proposed model outperforms baseline models and differs from conventional ensemble approaches. The code is available at https://github.com/young-j-parld22-ICDM-Forchestra.
更多查看译文
关键词
demand forecasting,learning,large-scale large-scale
AI 理解论文
溯源树
样例
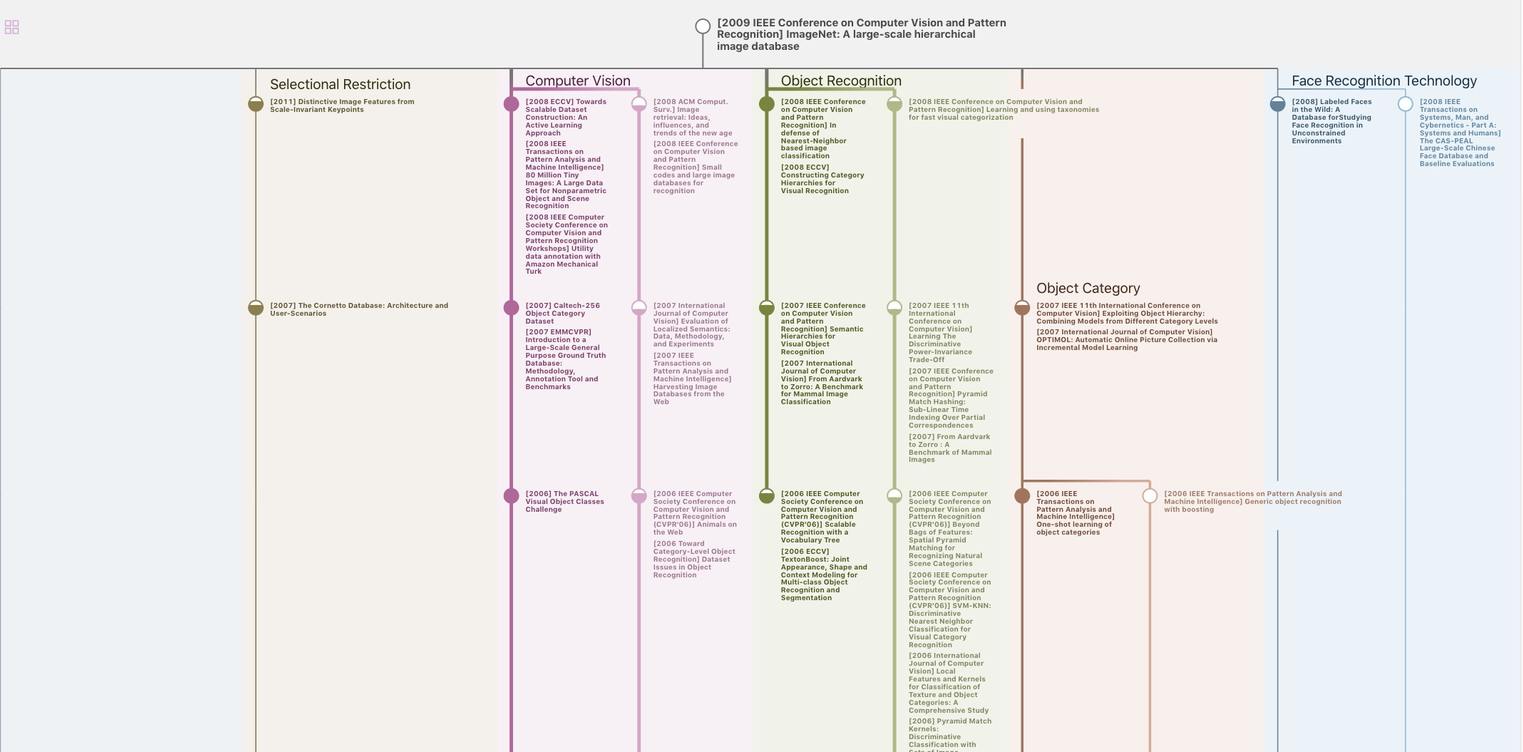
生成溯源树,研究论文发展脉络
Chat Paper
正在生成论文摘要