On the Role of Morphological Information for Contextual Lemmatization
arxiv(2023)
摘要
Lemmatization is a Natural Language Processing (NLP) task which consists of producing, from a given inflected word, its canonical form or lemma. Lemmatization is one of the basic tasks that facilitate downstream NLP applications, and is of particular importance for high-inflected languages. Given that the process to obtain a lemma from an inflected word can be explained by looking at its morphosyntactic category, including fine-grained morphosyntactic information to train contextual lemmatizers has become common practice, without analyzing whether that is the optimum in terms of downstream performance. Thus, in this paper we empirically investigate the role of morphological information to develop contextual lemmatizers in six languages within a varied spectrum of morphological complexity: Basque, Turkish, Russian, Czech, Spanish and English. Furthermore, and unlike the vast majority of previous work, we also evaluate lemmatizers in out-of-domain settings, which constitutes, after all, their most common application use. The results of our study are rather surprising: (i) providing lemmatizers with fine-grained morphological features during training is not that beneficial, not even for agglutinative languages; (ii) in fact, modern contextual word representations seem to implicitly encode enough morphological information to obtain good contextual lemmatizers without seeing any explicit morphological signal; (iii) the best lemmatizers out-of-domain are those using simple UPOS tags or those trained without morphology; (iv) current evaluation practices for lemmatization are not adequate to clearly discriminate between models.
更多查看译文
关键词
morphological information
AI 理解论文
溯源树
样例
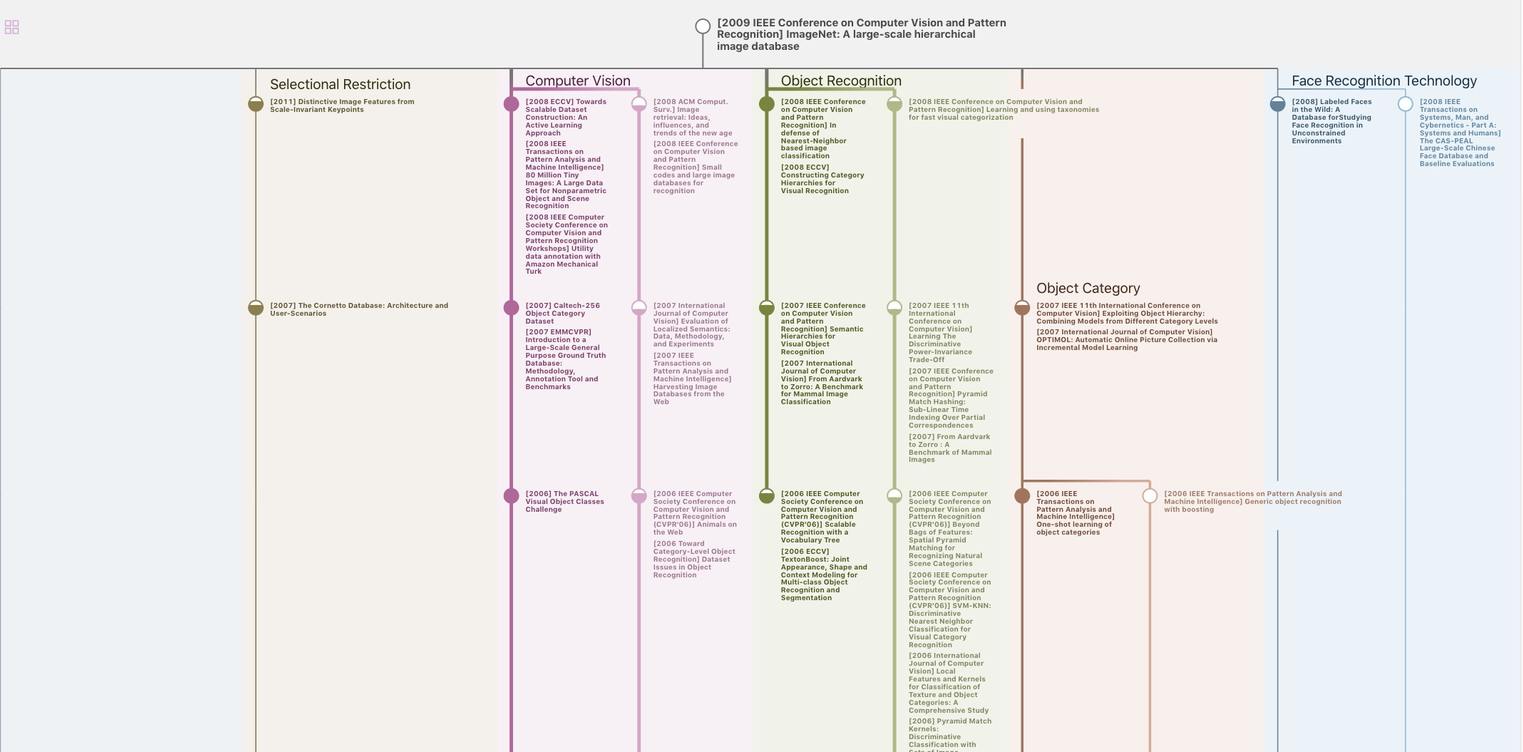
生成溯源树,研究论文发展脉络
Chat Paper
正在生成论文摘要