Tight Data Access Bounds for Private Top-k Selection.
arxiv(2023)
摘要
We study the top-$k$ selection problem under the differential privacy model: $m$ items are rated according to votes of a set of clients. We consider a setting in which algorithms can retrieve data via a sequence of accesses, each either a random access or a sorted access; the goal is to minimize the total number of data accesses. Our algorithm requires only $O(\sqrt{mk})$ expected accesses: to our knowledge, this is the first sublinear data-access upper bound for this problem. Accompanying this, we develop the first lower bounds for the problem, in three settings: only random accesses; only sorted acceses; a sequence of accesses of either kind. We show that, to avoid $\Omega(m)$ access cost, supporting \emph{either} kind of access, i.e. the freedom to mix, is necessary, and that in this case our algorithm's access cost is almost optimal.
更多查看译文
关键词
selection,access,bounds
AI 理解论文
溯源树
样例
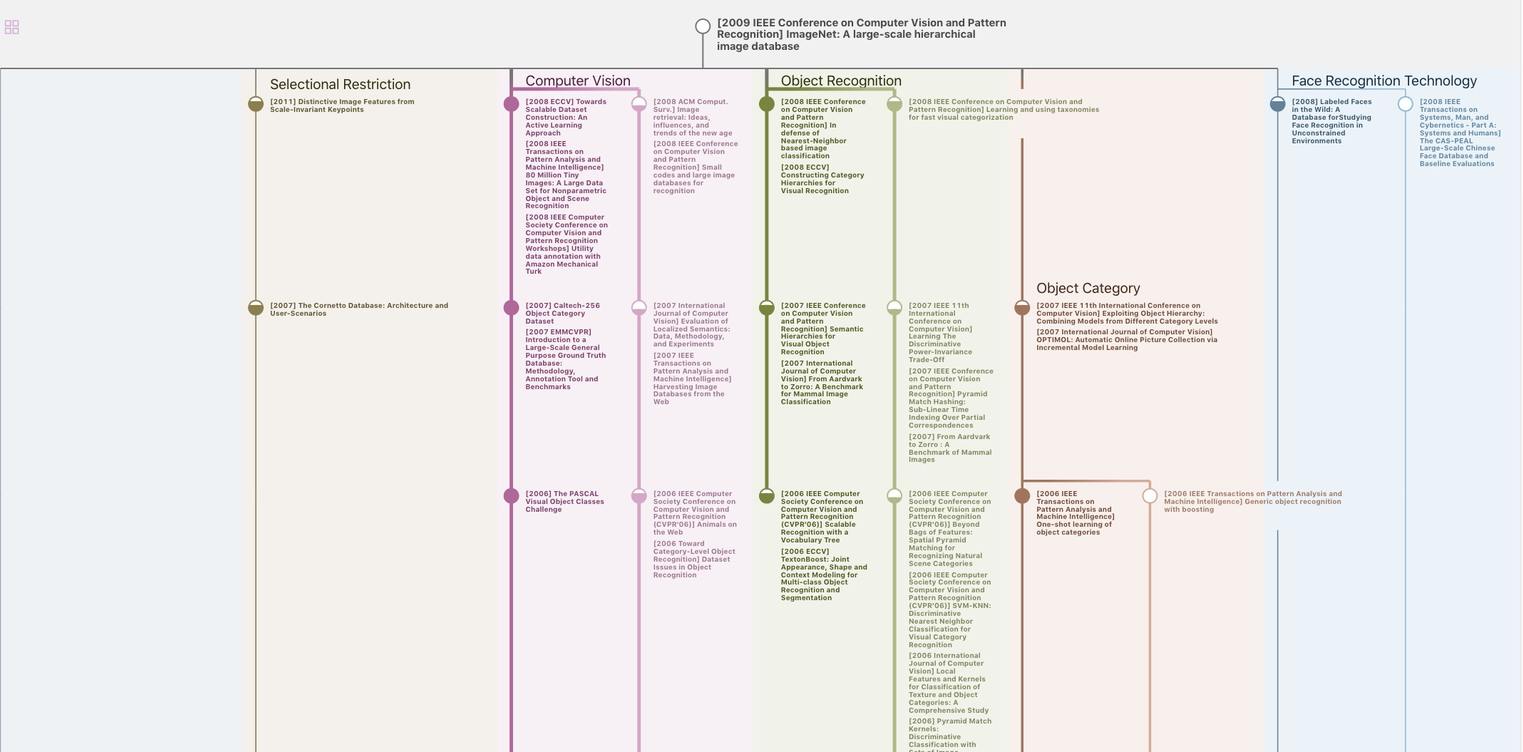
生成溯源树,研究论文发展脉络
Chat Paper
正在生成论文摘要