Euclid Preparation XXIX: Forecasts for 10 different higher-order weak lensing statistics
arxiv(2023)
摘要
Recent cosmic shear studies have shown that higher-order statistics (HOS) developed by independent teams now outperform standard two-point estimators in terms of statistical precision thanks to their sensitivity to the non-Gaussian features of large-scale structure. The aim of the Higher-Order Weak Lensing Statistics (HOWLS) project is to assess, compare, and combine the constraining power of $10$ different HOS on a common set of $Euclid$-like mocks, derived from N-body simulations. In this first paper of the HOWLS series we compute the non-tomographic ($\Omega_{\rm m}$, $\sigma_8$) Fisher information for one-point probability distribution function, peak counts, Minkowski functionals, Betti numbers, persistent homology Betti numbers and heatmap, and scattering transform coefficients, and compare them to the shear and convergence two-point correlation functions in the absence of any systematic bias. We also include forecasts for three implementations of higher-order moments, but these cannot be robustly interpreted as the Gaussian likelihood assumption breaks down for these statistics. Taken individually, we find that each HOS outperforms the two-point statistics by a factor of around $2$ in the precision of the forecasts with some variations across statistics and cosmological parameters. When combining all the HOS, this increases to a $4.5$ times improvement, highlighting the immense potential of HOS for cosmic shear cosmological analyses with $Euclid$. The data used in this analysis are publicly released with the paper.
更多查看译文
关键词
euclid preparation xxix,forecasts,higher-order
AI 理解论文
溯源树
样例
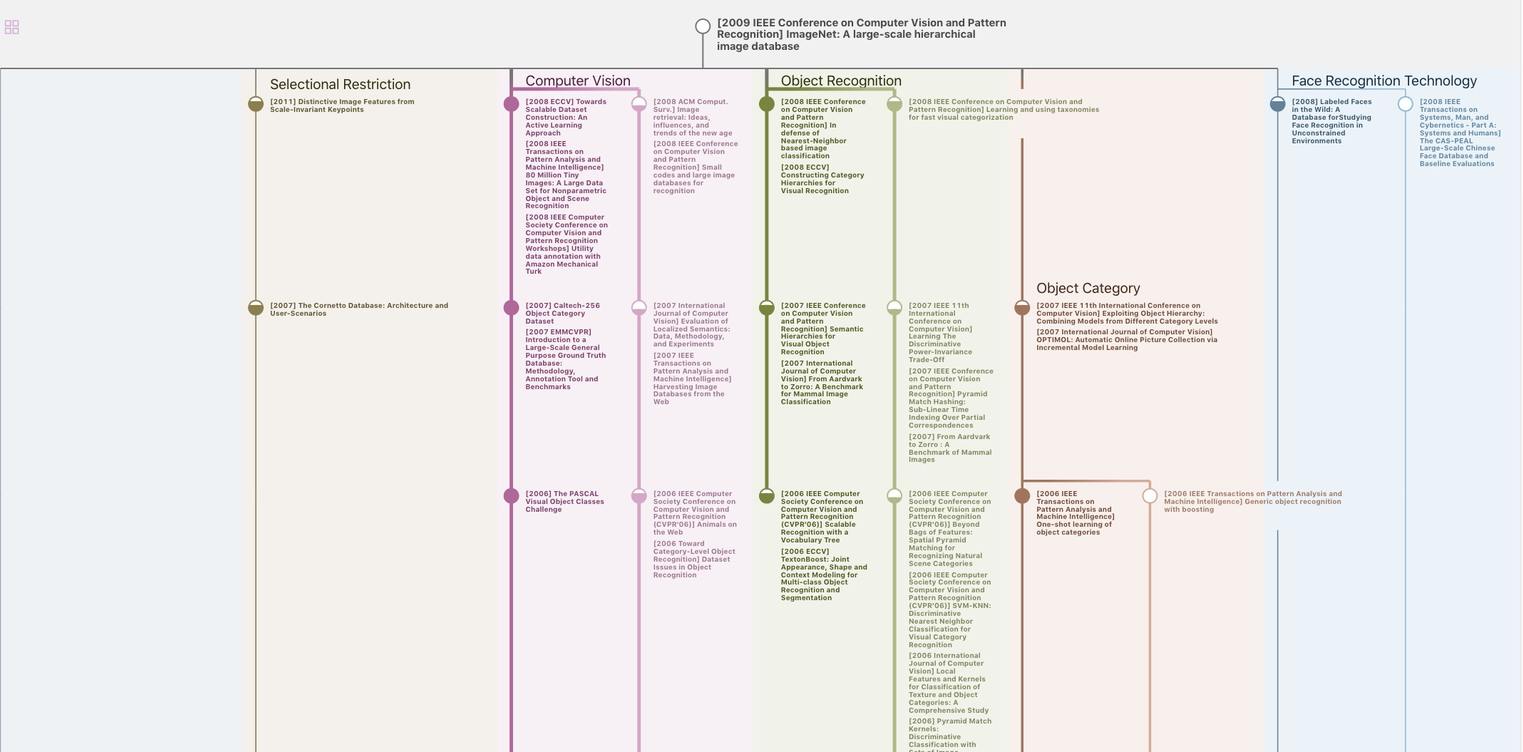
生成溯源树,研究论文发展脉络
Chat Paper
正在生成论文摘要