Machine-learning-informed parameter estimation improves the reliability of spinal cord diffusion MRI
arxiv(2023)
摘要
Purpose: We address the challenge of inaccurate parameter estimation in diffusion MRI when the signal-to-noise ratio (SNR) is very low, as in the spinal cord. The accuracy of conventional maximum-likelihood estimation (MLE) depends highly on initialisation. Unfavourable choices could result in suboptimal parameter estimates. Current methods to address this issue, such as grid search (GS) can increase computation time substantially. Methods: We propose a machine learning (ML) informed MLE approach that combines conventional MLE with ML approaches synergistically. ML-based methods have been developed recently to improve the speed and precision of parameter estimation. However, they can generate high systematic bias in estimated parameters when SNR is low. In the proposed ML-MLE approach, an artificial neural network model is trained to provide sensible initialisation for MLE efficiently, with the final solution determined by MLE, avoiding biases typically affecting pure ML estimations. Results: Using parameter estimation of neurite orientation dispersion and density imaging as an example, simulation and in vivo experiments suggest that the ML-MLE method can reduce outlier estimates from conventional MLE in white matter voxels affected by CSF contamination. It also accelerates computation compared to GS-MLE. Conclusion: The ML-MLE method can improve the reliability of parameter estimation with reduced computation time compared to GS-MLE, making it a practical tool for diffusion dataset with low SNR.
更多查看译文
关键词
spinal cord diffusion mri,parameter estimation,machine-learning-informed machine-learning-informed
AI 理解论文
溯源树
样例
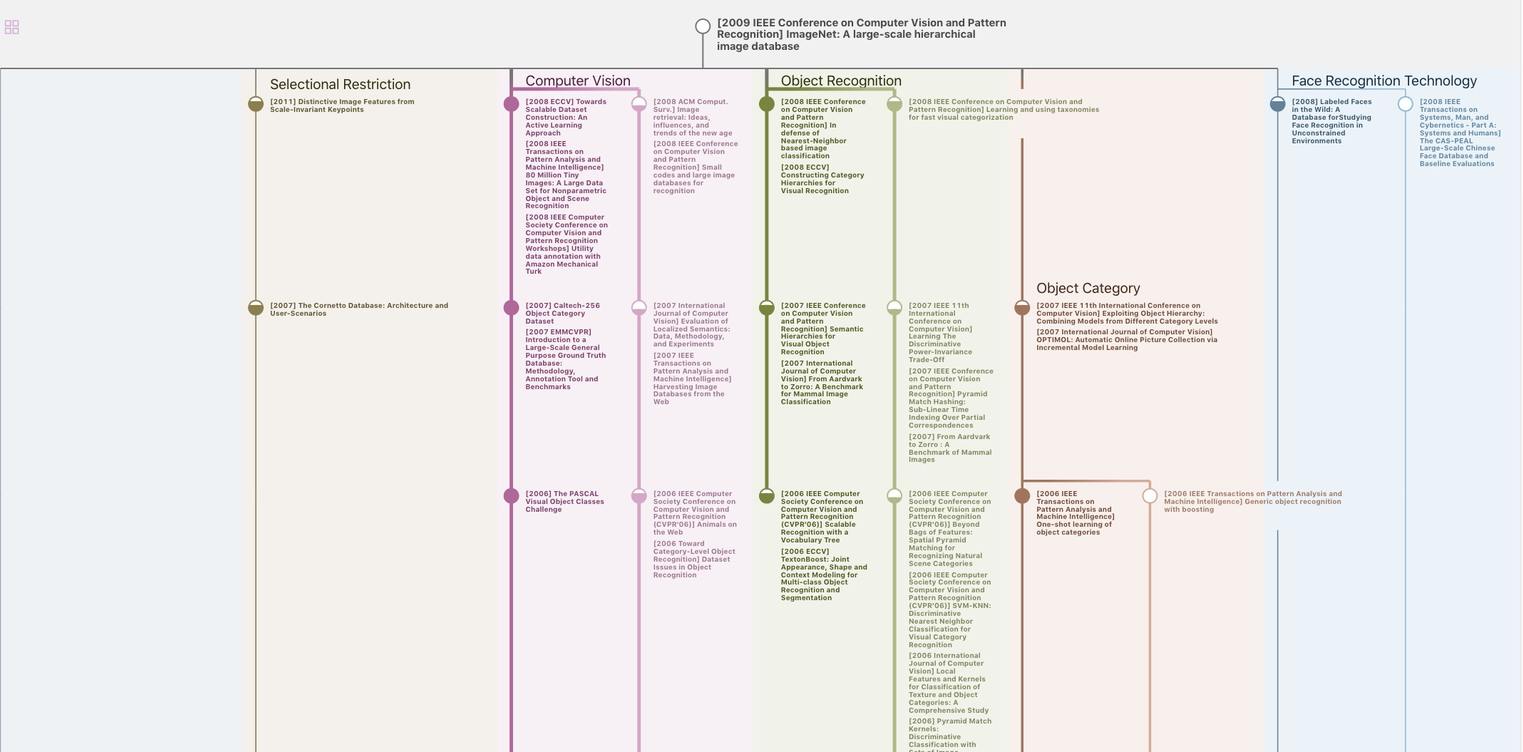
生成溯源树,研究论文发展脉络
Chat Paper
正在生成论文摘要