Assertion Inferring Mutants
arxiv(2023)
摘要
Specification inference techniques aim at (automatically) inferring a set of assertions that capture the exhibited software behaviour by generating and filtering assertions through dynamic test executions and mutation testing. Although powerful, such techniques are computationally expensive due to a large number of assertions, test cases and mutated versions that need to be executed. To overcome this issue, we demonstrate that a small subset, i.e., 12.95% of the mutants used by mutation testing tools is sufficient for assertion inference, this subset is significantly different, i.e., 71.59% different from the subsuming mutant set that is frequently cited by mutation testing literature, and can be statically approximated through a learning based method. In particular, we propose AIMS, an approach that selects Assertion Inferring Mutants, i.e., a set of mutants that are well-suited for assertion inference, with 0.58 MCC, 0.79 Precision, and 0.49 Recall. We evaluate AIMS on 46 programs and demonstrate that it has comparable inference capabilities with full mutation analysis (misses 12.49% of assertions) while significantly limiting execution cost (runs 46.29 times faster). A comparison with randomly selected sets of mutants, shows the superiority of AIMS by inferring 36% more assertions while requiring approximately equal amount of execution time. We also show that AIMS 's inferring capabilities are almost complete as it infers 96.15% of ground truth assertions, (i.e., a complete set of assertions that were manually constructed) while Random Mutant Selection infers 19.23% of them. More importantly, AIMS enables assertion inference techniques to scale on subjects where full mutation testing is prohibitively expensive and Random Mutant Selection does not lead to any assertion.
更多查看译文
关键词
mutants
AI 理解论文
溯源树
样例
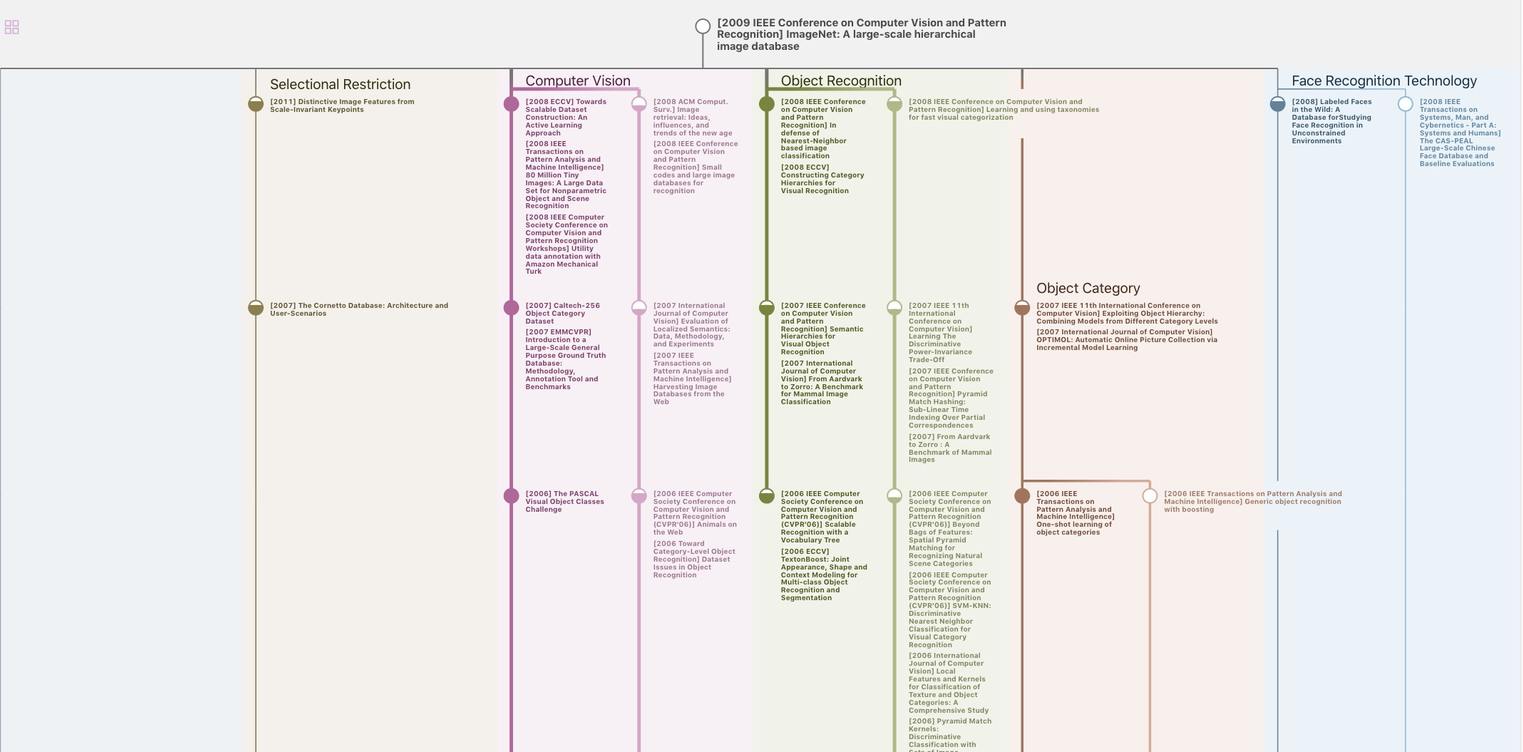
生成溯源树,研究论文发展脉络
Chat Paper
正在生成论文摘要