Learning cost action planning models with perfect precision via constraint propagation
Information Sciences(2023)
摘要
Data-driven AI is rapidly gaining importance. In the context of AI planning, a constraint programming formulation for learning action models in a data-driven fashion is proposed. Data comprises plan observations, which are automatically transformed into a set of planning constraints which need to be satisfied. The formulation captures the essence of the action model and unifies functionalities that are individually supported by other learning approaches, such as costs, noise/uncertainty on actions, information on intermediate state observations and mutex reasoning.Reliability is a key concern in data-driven learning, but existing approaches usually learn action models that can be imprecise, where imprecision here is an error indicator of learning something incorrect. On the contrary, the proposed approach guarantees reliability in terms of perfect precision by using constraint propagation. This means that what is learned is 100% correct (i.e., error-free), not only for the initial observations, but also for future observations. To our knowledge, this is a novelty in action model learning literature. Although perfect precision might potentially limit the amount of learned information, the exhaustive experiments over 20 planning domains show that such amount is comparable, and even better, to ARMS and FAMA, two state-of-the-art benchmarks in action model learning.
更多查看译文
关键词
Learning,Cost action model,Classical planning,Constraint programming,Reliability,Precision
AI 理解论文
溯源树
样例
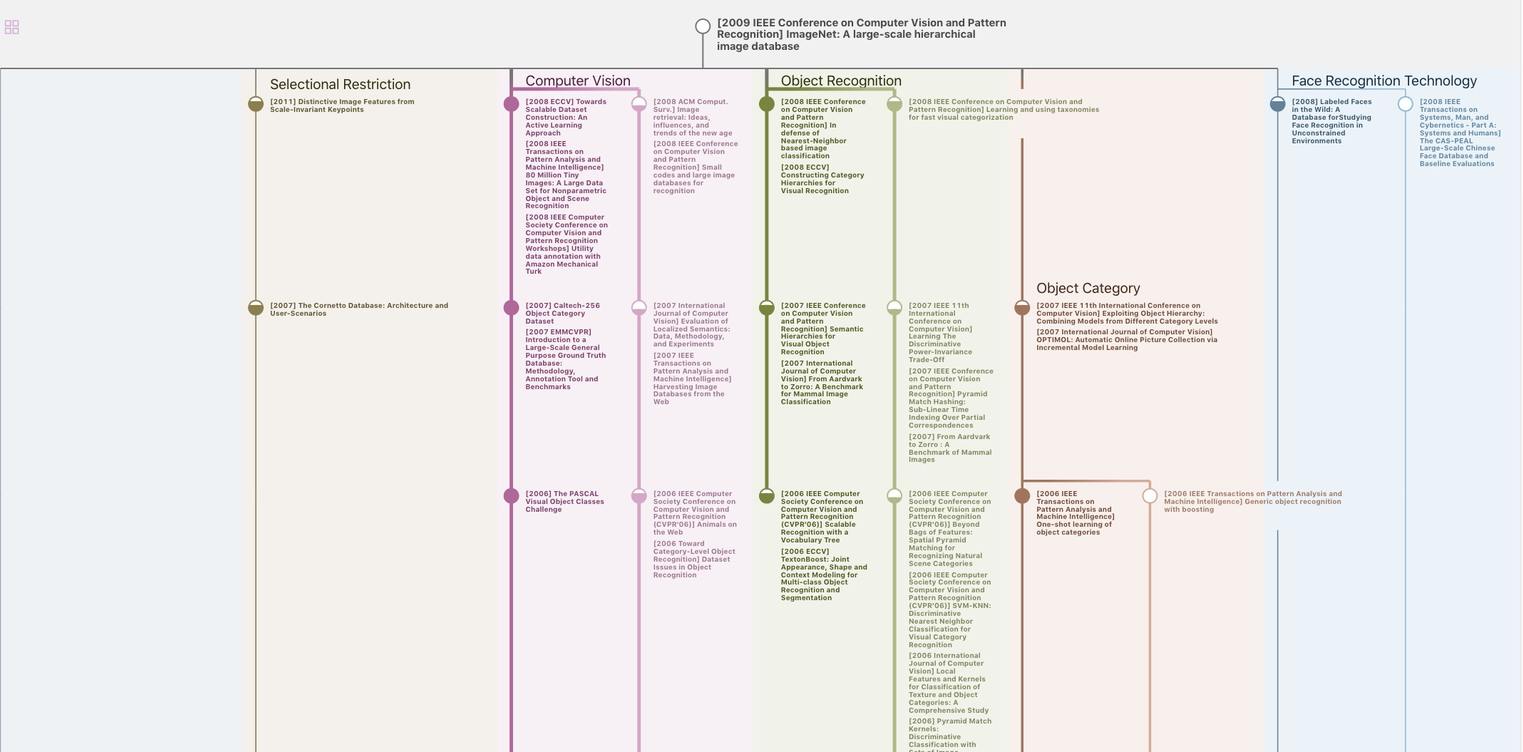
生成溯源树,研究论文发展脉络
Chat Paper
正在生成论文摘要