N-of-one differential gene expression without control samples using a deep generative model
biorxiv(2023)
摘要
Differential analysis of bulk RNA-seq data often suffers from lack of good controls. Here, we present a generative model that replaces controls, trained solely on healthy tissues. The unsupervised model learns a low-dimensional representation and can identify the closest normal representation for a given disease sample. This enables control-free, single-sample differential expression analysis. In breast cancer, we demonstrate how our approach selects marker genes and outperforms a state-of-the-art method. Furthermore, significant genes identified by the model are enriched in driver genes across cancers. Our results show that the in silico closest normal provides a more favorable comparison than control samples.
更多查看译文
关键词
Deep generative models,Deep learning,Differential expression analysis,DEG,DEseq2,Transcriptomics
AI 理解论文
溯源树
样例
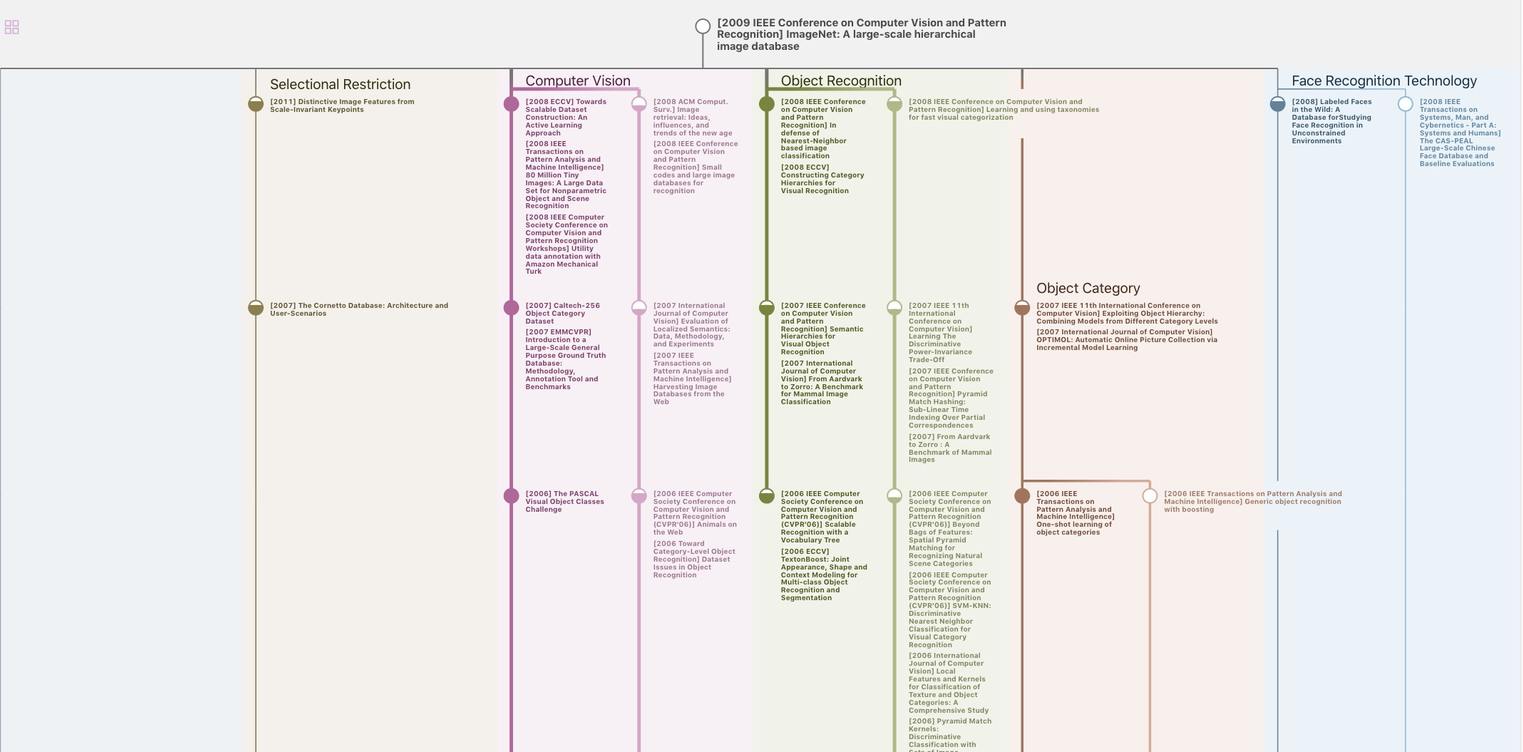
生成溯源树,研究论文发展脉络
Chat Paper
正在生成论文摘要