Dual collaboration for decentralized multi-source domain adaptation
Frontiers Inf. Technol. Electron. Eng.(2022)
摘要
The goal of decentralized multi-source domain adaptation is to conduct unsupervised multi-source domain adaptation in a data decentralization scenario. The challenge of data decentralization is that the source domains and target domain lack cross-domain collaboration during training. On the unlabeled target domain, the target model needs to transfer supervision knowledge with the collaboration of source models, while the domain gap will lead to limited adaptation performance from source models. On the labeled source domain, the source model tends to overfit its domain data in the data decentralization scenario, which leads to the negative transfer problem. For these challenges, we propose dual collaboration for decentralized multi-source domain adaptation by training and aggregating the local source models and local target model in collaboration with each other. On the target domain, we train the local target model by distilling supervision knowledge and fully using the unlabeled target domain data to alleviate the domain shift problem with the collaboration of local source models. On the source domain, we regularize the local source models in collaboration with the local target model to overcome the negative transfer problem. This forms a dual collaboration between the decentralized source domains and target domain, which improves the domain adaptation performance under the data decentralization scenario. Extensive experiments indicate that our method outperforms the state-of-the-art methods by a large margin on standard multi-source domain adaptation datasets.
更多查看译文
关键词
Multi-source domain adaptation,Data decentralization,Domain shift,Negative transfer
AI 理解论文
溯源树
样例
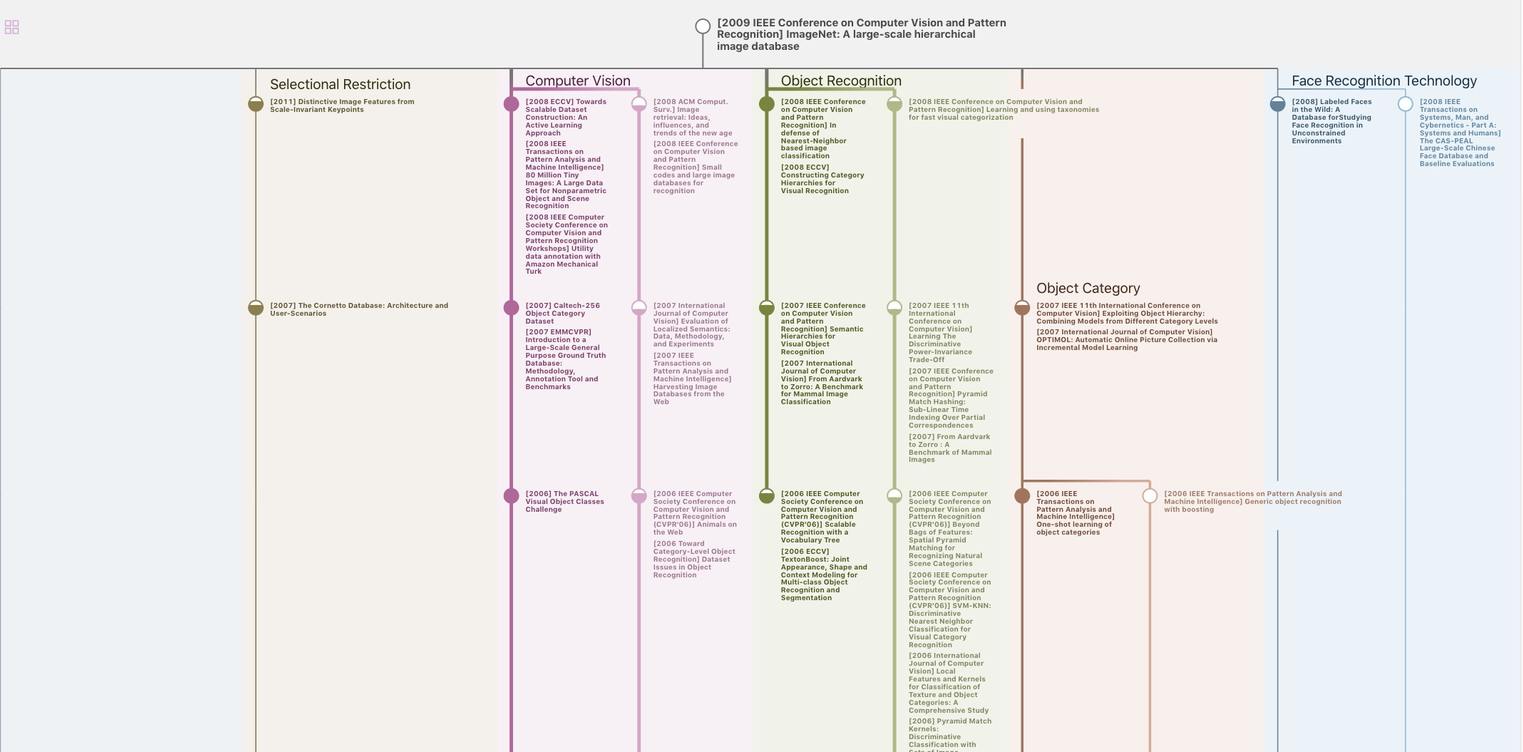
生成溯源树,研究论文发展脉络
Chat Paper
正在生成论文摘要