MFIALane: Multiscale Feature Information Aggregator Network for Lane Detection.
IEEE Trans. Intell. Transp. Syst.(2022)
摘要
Lane detection differs from general object detection in that lane lines are usually long and narrow in the road image, and more attention to image features at different scales is required to reason about lane lines under occlusion, degradation, and bad weather. However, most existing semantic segmentation-based lane detection methods focus on solving the convolutional receptive field through aggregating information vertically and horizontally in the same feature map, which may ignore important information contained in multi-scale features. Besides, the high-level semantic information of whether the lane exists is not fully utilized, as they often add a module at the final stage of the network output to determine whether the lane exists, which is a dispensable for their network. Based on the above analysis, we design a novel lane detection network based on semantic segmentation which consists of a Multi-scale Feature Information Aggregator (MFIA) module and a Channel Attention (CA) module. Many experiments on the TRLane dataset, the generated Lane dataset, BDD100K dataset, TuSimple dataset, VIL-100 dataset and CULane dataset show that our approach can achieve the state-of-the-art performance (our code will be available at https://github.com/Cuibaby/MFIALane). In addition, considering that different perceptual tasks in autonomous driving are able to share the feature extraction network, we also conduct the experiment for drivable area segmentation on BDD100K dataset. Our approach also achieves good results compared to many existing methods, showing that our proposed model is capable of simultaneously handling multiple perceptual tasks in autonomous driving scenarios.
更多查看译文
关键词
Lane detection,Feature extraction,Semantics,Task analysis,Decoding,Mathematical models,Proposals,Deep learning,lane detection,semantic segmentation,autonomous driving
AI 理解论文
溯源树
样例
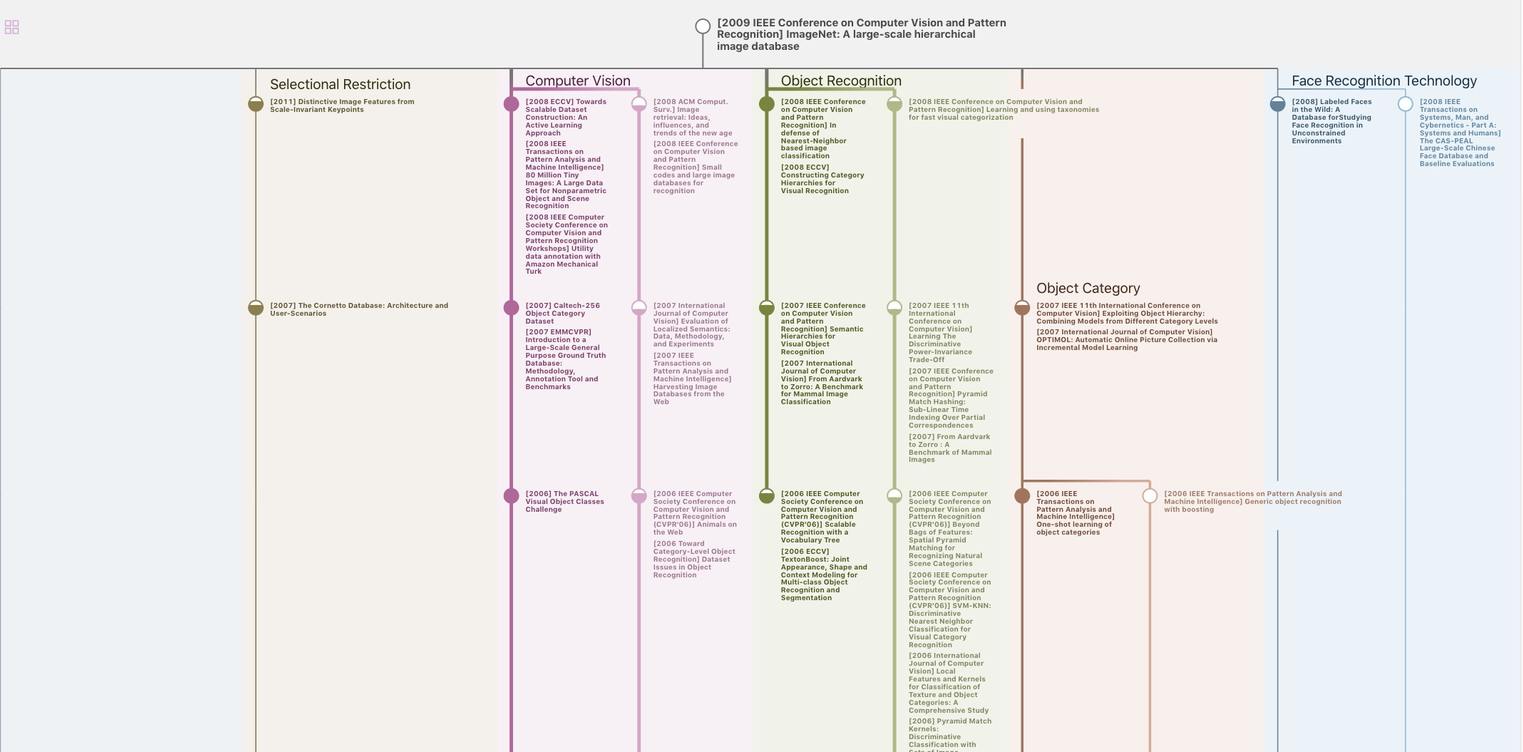
生成溯源树,研究论文发展脉络
Chat Paper
正在生成论文摘要