Latent energy preserving embedding for unsupervised feature selection.
Digit. Signal Process.(2022)
摘要
As is a fundamental yet challenging topic in machine learning and pattern recognition, feature selection has received much attention. Since data are often unlabeled in real-world applications, unsupervised feature selection (UFS) methods have aroused extensive attention. In this article, we present a novel latent energy preserving embedding (LEPE) method for UFS. First, we rewrite subspace learning into the form of energy preserving. Then, a novel self-representation learning strategy is utilized in the feature selection framework, in which the low-rank and sparse constraints are imposed on the representation matrix. In addition, we utilize a graph Laplacian to mine the manifold information of data. Meanwhile, we use an l(2,1)-norm for feature selection. To validate the effectiveness of the proposed LEPE method, we conduct extensive experiments on six real-world datasets. Experimental results illustrate the effectiveness of the proposed method. (C) 2022 Elsevier Inc. All rights reserved.
更多查看译文
关键词
Feature selection,Energy preserving,Graph regularization,Self-representation
AI 理解论文
溯源树
样例
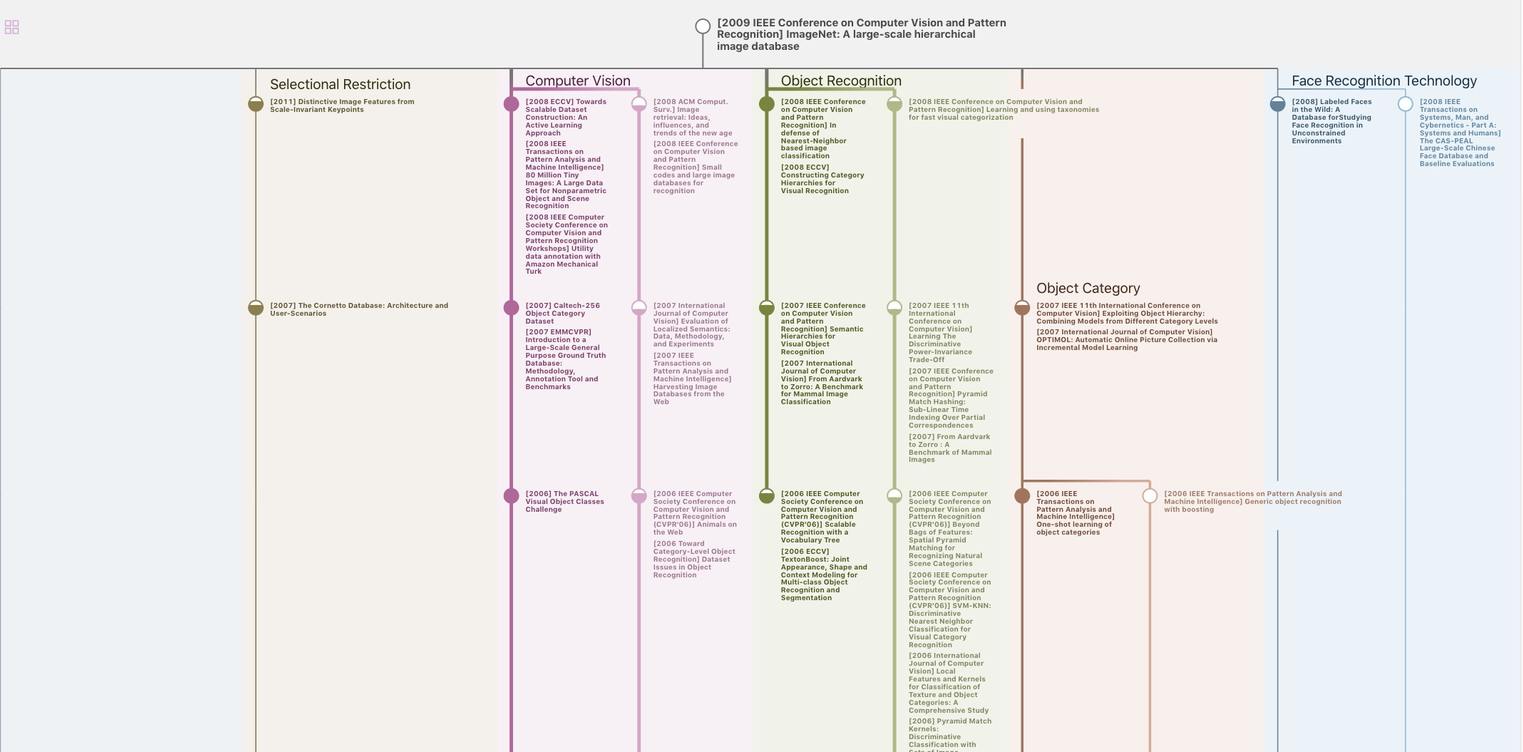
生成溯源树,研究论文发展脉络
Chat Paper
正在生成论文摘要