FedGait: A Benchmark for Federated Gait Recognition.
ICPR(2022)
摘要
Gait recognition has been greatly improved by deep learning and can achieve a relative high accuracy. The advances depend on the data size of gait. However, due to public concerns on privacy and regulations and laws from different countries, it is very difficult and almost impossible to collect a huge centralized gait database for algorithm training. Federated learning is a distributed machine learning technique for privacy-preserving, and can help to solve the problem. We propose a federated gait recognition benchmark, FedGait, to train algorithms using distributed gait data. It is the first benchmark on gait recognition to the best of our knowledge. FedGait can utilizes the gait videos available on multiple clients to learn a robust and generalized model. Based on the real-world gait scenarios, we introduce two federated gait recognition scenarios: institution-based scenario (IBS) and device-based scenario (DBS). Compared with centralized training, federated learning will encounter more serious heterogeneous data and data imbalance problems. We employ four popular databases for experiments, CASIA-B, CASIA-E, ReSGait and OU-MVLP, are involved in FedGait to investigate the problems in federated learning. We hope FedGait is a good start to solve data privacy problem in gait recognition.
更多查看译文
关键词
CASIA-B,CASIA-E,centralized training,data imbalance problems,data privacy problem,DBS,deep learning,device-based scenario,distributed gait data,distributed machine learning technique,federated gait recognition benchmark,federated gait recognition scenarios,federated learning,FedGait,gait recognition,gait videos,huge centralized gait database,IBS,institution-based scenario,OU-MVLP,real-world gait scenarios,ReSGait,serious heterogeneous data
AI 理解论文
溯源树
样例
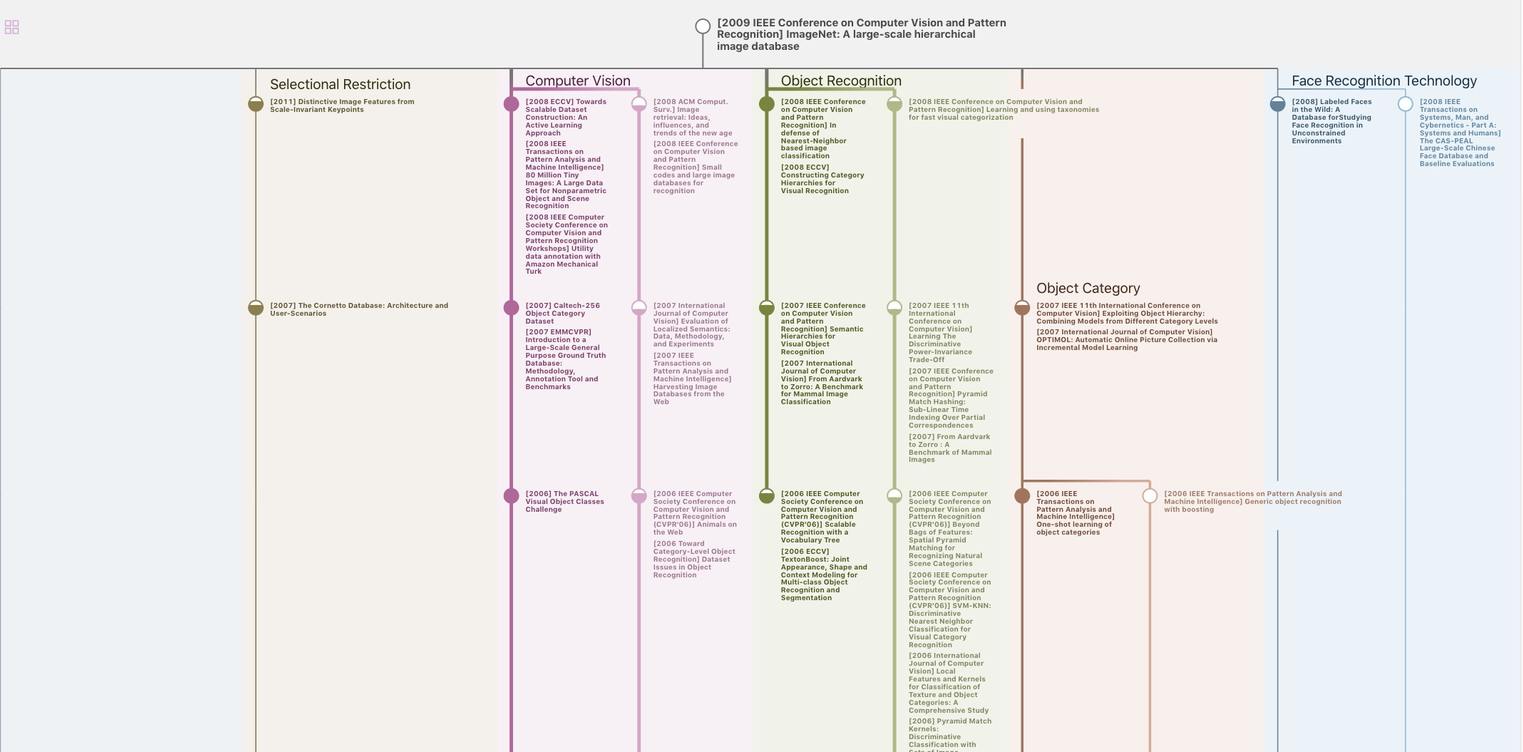
生成溯源树,研究论文发展脉络
Chat Paper
正在生成论文摘要