AutoMF: Spatio-temporal Architecture Search for The Meteorological Forecasting Task.
ICPR(2022)
摘要
Despite years of studies, meteorological forecasting with deep learning still faces several challenges, including the multi-modal correlation, the spatio-temporal dependency, the spatial heterogeneity, and the temporal periodicity. Though with elaborate design, manually designed networks adopted by current methods could be far from optimal in modeling the spatiotemporal dynamics of meteorological data. In this work, we propose AutoMF, i.e. a multi-level architecture search framework for the Meteorological Forecasting task. Working in a data-driven paradigm, AutoMF is capable of generating suitable convolution networks and spatio-temporal networks to capture the multimodal correlation and the spatio-temporal dependency. Based on this framework, we develop a differential sampling-based architecture search method to optimize the architecture, and introduce the progressive search strategy to facilitate the search process. Furthermore, the spatial heterogeneity and temporal periodicity are explicitly modeled through integrating corresponding status indicators. Extensive experiments exhibit the capability of the proposed method.
更多查看译文
关键词
forecasting,architecture,search,spatio-temporal
AI 理解论文
溯源树
样例
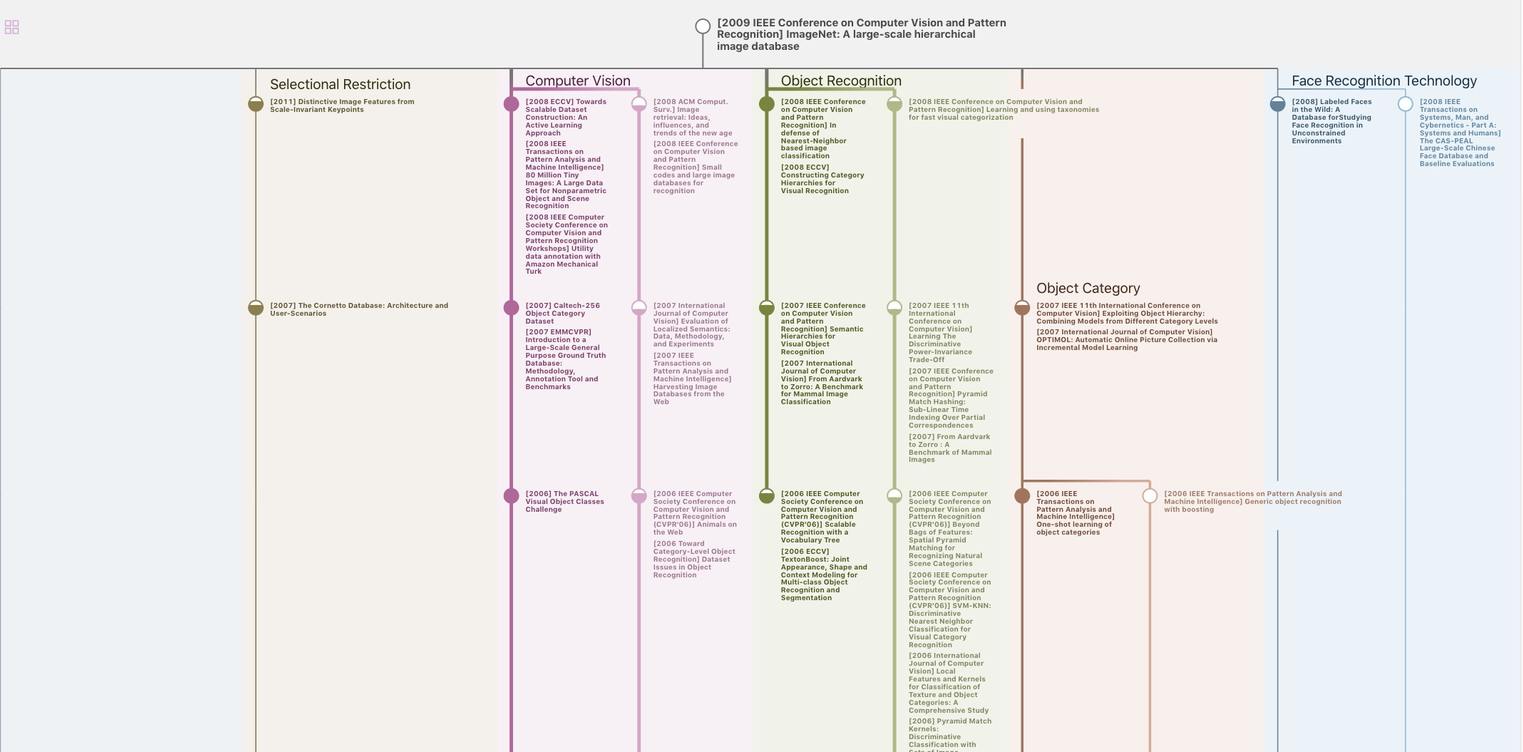
生成溯源树,研究论文发展脉络
Chat Paper
正在生成论文摘要