Orthogonal regularizers in deep learning: how to handle rectangular matrices?
ICPR(2022)
摘要
Orthogonal regularizers typically promote column orthonormality of some matrix W is an element of R-n (x) (p), by measuring the discrepancy between W.W and the identity according to some matrix norm. This paper explores the behavior of these regularizers when W is horizontal (n < p), so that column orthonormality cannot be achieved. Our motivation comes from orthogonal regularization of feed-forward neural networks: it is there desired to regularize all (vertical and horizontal) weight matrices of the model. One possible solution to address this issue is to transpose horizontal matrices before regularization. We prove that transposition is useless for the Frobenius norm (squared), as the corresponding regularizer promotes simultaneously orthonormality of the rows and of the columns ofW. On the other hand, we highlight important qualitative differences with newer regularizers, including the MC and SRIP orthogonal regularizers. We conclude the paper with some numerical results supporting our theoretical findings.
更多查看译文
关键词
orthogonal regularizers,rectangular matrices,deep learning
AI 理解论文
溯源树
样例
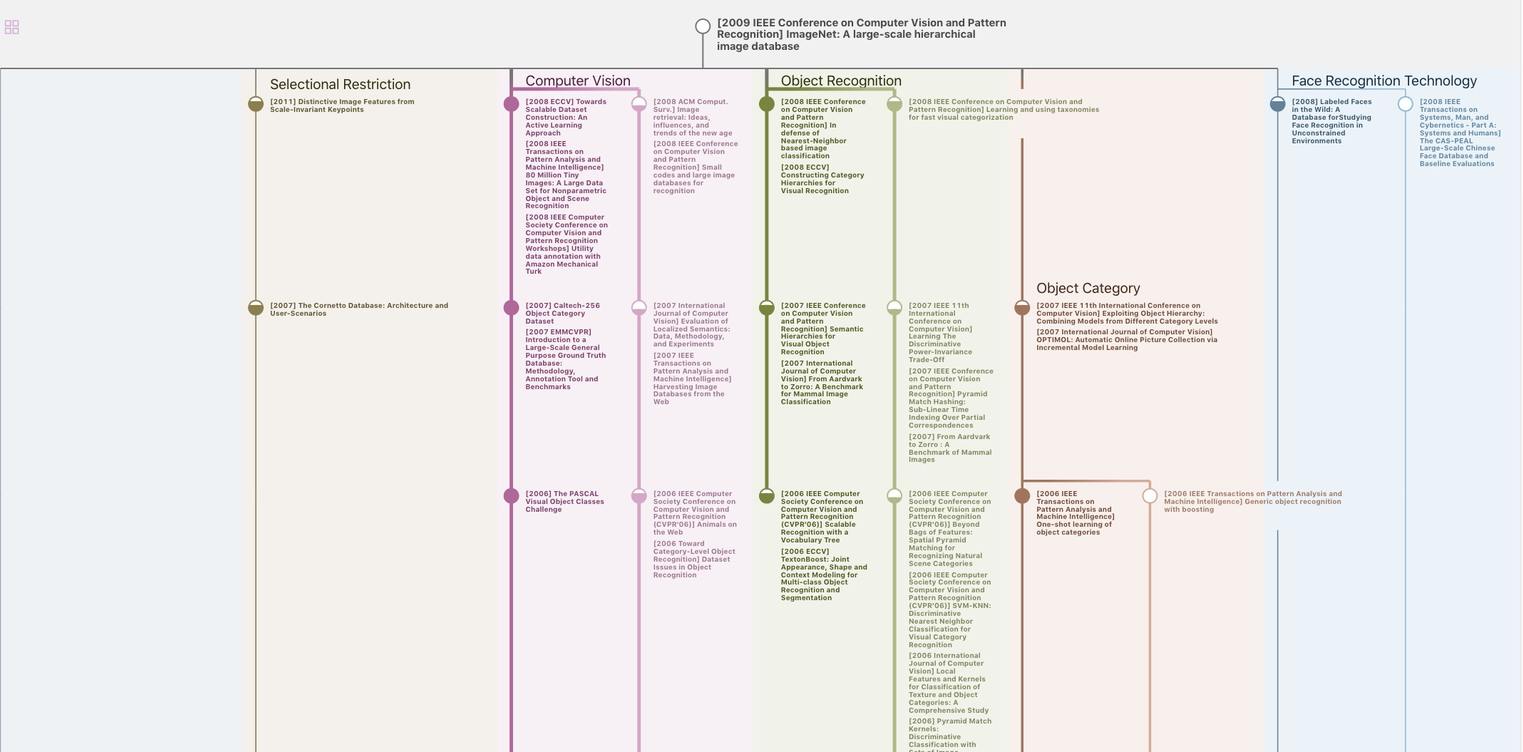
生成溯源树,研究论文发展脉络
Chat Paper
正在生成论文摘要