Prototype Augmentation with Dummy Samples.
ICPR(2022)
摘要
Meta-learning is proven to be powerful for helping models make quick adaptations to new tasks. However, the lack of data severely constrains the further improvement. As data augmentation has been an effective and commonly used approach to reach state-of-art performance in image classification tasks, different strategies for applying data augmentation to meta-learning have emerged. One common combination of data augmentation and meta-learning is performing different transformations on images(e.g. horizontal flip, color jitter, and random crop) before feeding them to the feature extractor. Another way is using generative models, such as GAN, to expand the available dataset and alleviate the negative effects of lack of data. These methods either have limited boosts or have difficulties to converge during training and require significant extra computation. In this paper, we propose a novel method using a modified Variational Auto Encoder(VAE) to generate dummy samples from the support set. Our data augmentation method is performed in the feature space to reduce computation steps. Combined with prototypical networks we call our method as Prototype Augmentation with Dummy Samples(PADS). Experiments are carried out both in standard few-shot image classification and cross-domain scenarios and we achieved significant improvement with comparison to the baseline.
更多查看译文
关键词
cross-domain scenarios,data augmentation method,feature extractor,generative models,horizontal flip color jitter,image classification tasks,meta-learning,modified variational autoencoder,PADS,prototype augmentation with dummy samples,standard few-shot image classification,VAE
AI 理解论文
溯源树
样例
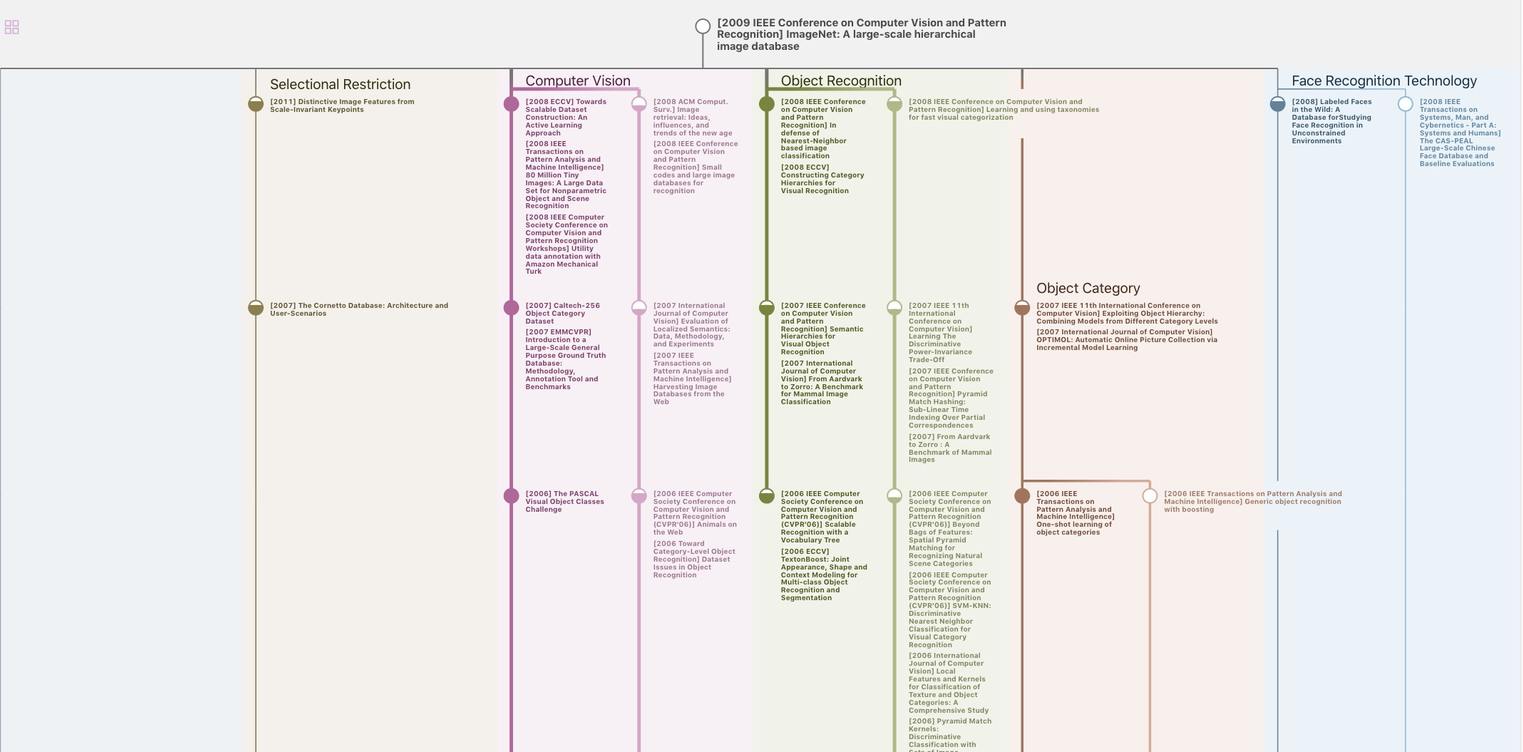
生成溯源树,研究论文发展脉络
Chat Paper
正在生成论文摘要