Self-Challenging Mask for Cross-Domain Few-Shot Classification.
ICPR(2022)
摘要
Few-shot classification (FSC) aims to recognize novel classes with few labeled samples in each class. Currently, meta-learning methods have achieved great success in few-shot classification tasks. However, most methods assume that base classes and novel classes share a single domain, and their performance can be greatly reduced when comes to domain-shift problem. To further improve the generalization of the existing FSC models, we propose a novel Self-Challenging Module including the Self-Challenging Mask and random noise. Self-Challenging Mask exploits the relationship between mid-level feature maps and high-level feature vectors to challenge (greatly weaken) the dominant mid-level local descriptors which are extracted in focus. Therefore, the model is forced to discover the residual information that correlates with the classification task from the remaining mid-level local descriptors. This method appears to expand the feature distribution for generalizing on unseen domains. We combine our method with three existing metric-based FSC model and conduct a large number of classification experiments in five datasets under the setting of cross-domain few-shot classification. The result shows that our Self-Challenging Module can significantly improve the classification accuracy in both seen and unseen domains.
更多查看译文
关键词
cross-domain few-shot classification,cross-domain FSC,domain-shift problem,high-level feature vectors,metric-based FSC model,mid-level feature maps,mid-level local descriptors,random noise,self-challenging mask,self-challenging module
AI 理解论文
溯源树
样例
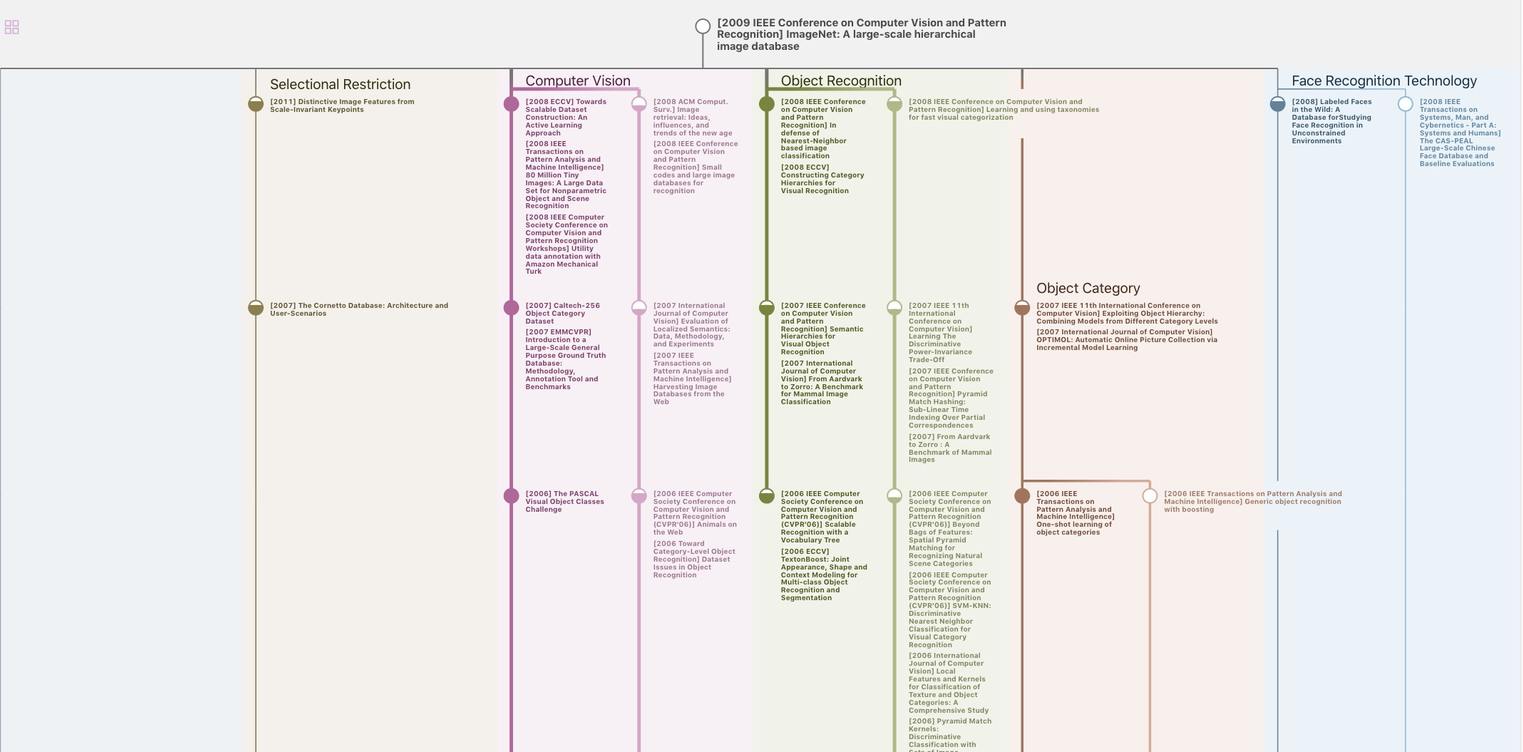
生成溯源树,研究论文发展脉络
Chat Paper
正在生成论文摘要