TextSRNet: Scene Text Super-Resolution Based on Contour Prior and Atrous Convolution.
ICPR(2022)
摘要
Low-resolution (LR) scene text images are often encountered in real application scenarios. It is difficult to recognize these scene texts due to the loss of their character details. Super-resolution (SR) is an intuitive alternative to solve this problem. Unlike generic SR, which mainly focuses on image quality, text SR focuses on the accuracy of the downstream recognition tasks. Although several scene text SR methods have been recently proposed, they ignore retaining character contours that are meaningful fir character readability and poorly perform on long texts. Therefore, we proposes a new scene text SR network called TextSRNet. In order to get fine character details, we adopt the segmentation maps of scene text images as the prior knowledge of character contours and embed it into the proposed TextSRNet. In addition, we incorporate Sobel loss to enhance the shape boundaries of characters. To improve the robustness of the model for long texts, we adopt a text atrous spatial pyramid pooling module. The proposed TextSRNet is trained and evaluated on 'fextZoom, a real-world scene text SR dataset. Extensive experiments demonstrate that our TextSRNet outperforms existing methods in terms of both recognition accuracy and image quality.
更多查看译文
关键词
atrous convolution,character contours,character readability,contour prior,downstream recognition tasks,fine character details,image quality,low-resolution scene text images,real-world scene text SR dataset,scene text SR network,scene text superresolution,segmentation map,Sobel loss,text atrous spatial pyramid pooling module,TextSRNet,TextZoom
AI 理解论文
溯源树
样例
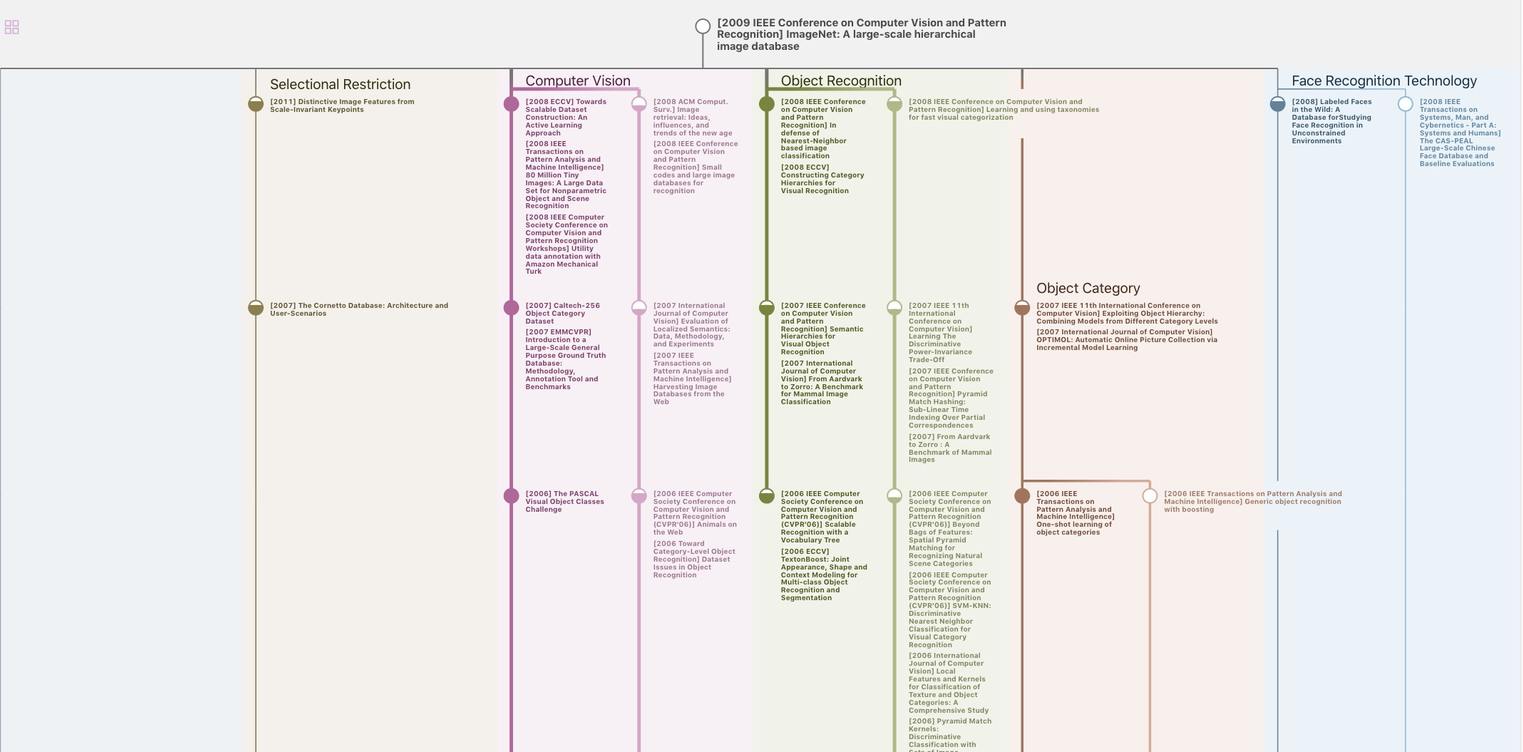
生成溯源树,研究论文发展脉络
Chat Paper
正在生成论文摘要