CAT: Re-Conv Attention in Transformer for Visual Question Answering.
ICPR(2022)
摘要
Visual Question Answering (VQA) is a challenging task that obtains the correct answer based on an image and a question related to the picture. Images often contain more information and local spatial relationships than text in this task. However, many current VQA models only utilize the original Transformer to capture the global relationships when performing image processing while ignoring the equally important local relationships. This paper proposes a novel Re-Conv Attention in the Transformer module (CAT) to solve the above problem. Specifically, self-attention is first used to extract the correlation between features (the global relationship). Then, depthwise separable convolution is utilized to extract exciting local information. Finally, the weight generated by the local essential information works on the global relationship extracted by self-attention, developing the local-guided global feature, which constitutes our re-attention mechanism, so that the module can capture the global and local relationships simultaneously. We combine the re-attention mechanism, FFN, and Layer-norm to form CAT. To validate CAT, we conduct extensive experiments on six benchmark datasets of VQA, Image-Text Matching (ITM), and Referring Expression Comprehension (REC) and achieve superior performance gains than the standard Transformer and a bunch of stats-of-the-art methods.
更多查看译文
关键词
Visual question answering, Transformer, Depthwise sparable convolution, Multi-modal task
AI 理解论文
溯源树
样例
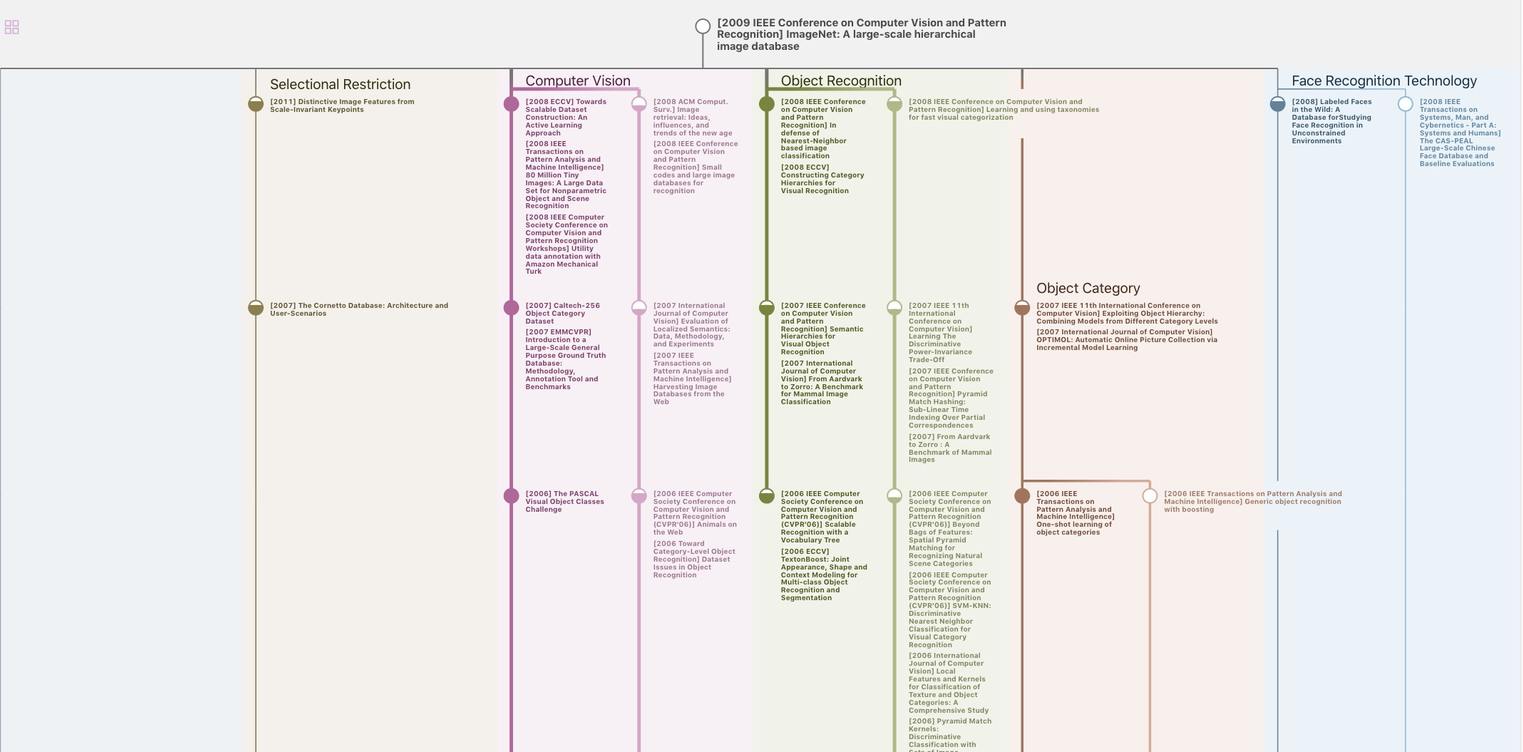
生成溯源树,研究论文发展脉络
Chat Paper
正在生成论文摘要