When Less May Be More: Exploring Similarity to Improve Experience Replay.
BRACIS (2)(2022)
摘要
We propose the COMPact Experience Replay (COMPER) as a reinforcement learning method that seeks to reduce the required number of experiences to agent training regarding the total accumulated rewards in the long run. COMPER uses temporal difference learning with predicted target values for sets of similar transitions and a new experience replay approach based on two memories of transitions. We present an assessment of two possible neural network architectures for the target network with a complete analysis of the memories' behavior, along with detailed results for 100,000 frames and about 25,000 iterations with a small experience memory on eight challenging 2600 Atari games on the Arcade Learning Environment (ALE). We also present results for a Deep Q-Network (DQN) agent with the same experimental protocol on the same set of games as a baseline. We demonstrate that COMPER can approximate a good policy from a small number of frame observations using a compact memory and learning the similar transitions' sets dynamics using a recurrent neural network.
更多查看译文
关键词
similarity,experience
AI 理解论文
溯源树
样例
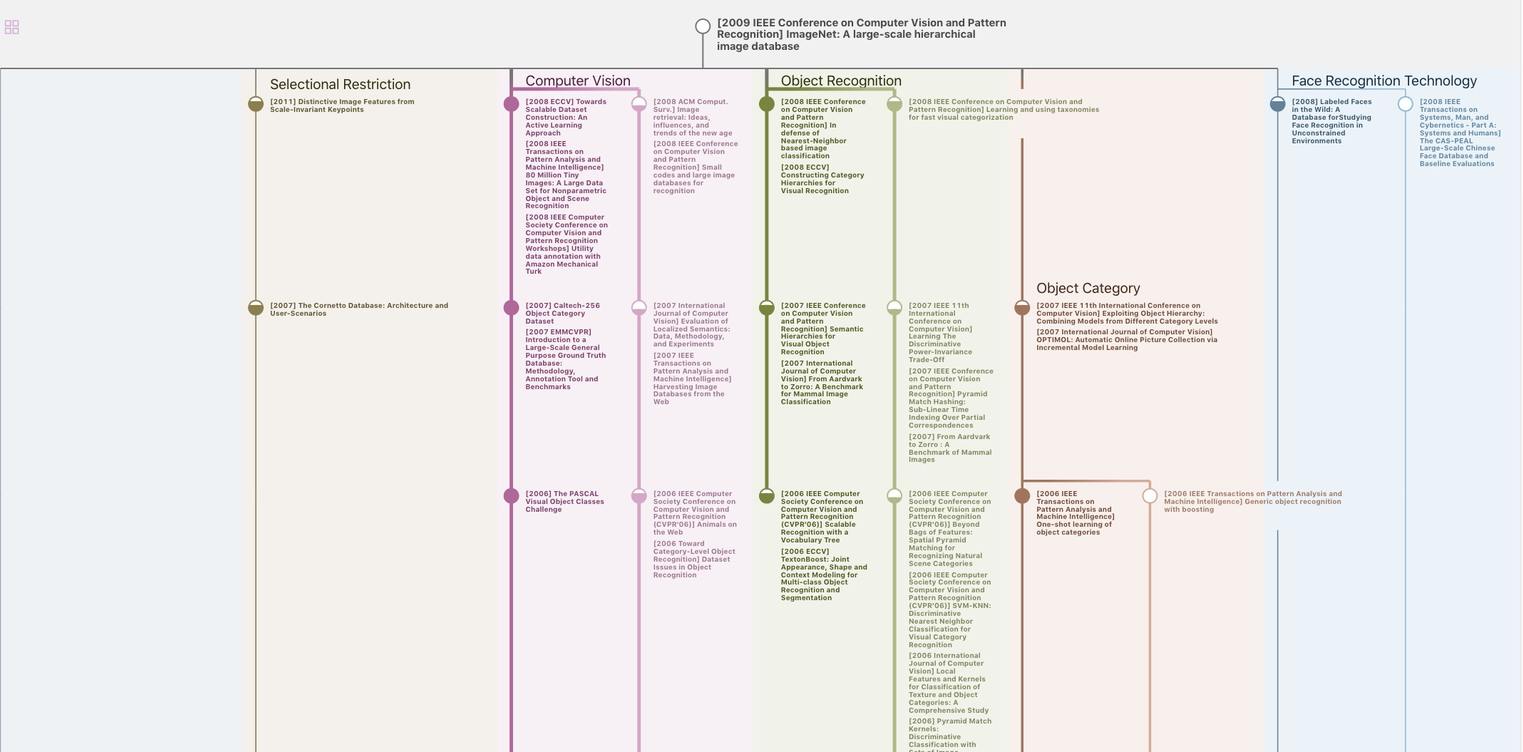
生成溯源树,研究论文发展脉络
Chat Paper
正在生成论文摘要