Cross-Domain Learning in Deep HAR Models via Natural Language Processing on Action Labels.
ISVC (1)(2022)
摘要
Nowadays, deep learning approaches lead the state-of-the-art scores in human activity recognition (HAR). However, the supervised nature of these approaches still relies heavily on the size and the quality of the available training datasets. The complexity of activities of existing HAR video datasets ranges from simple coarse actions, such as sitting, to complex activities, consisting of multiple actions with subtle variations in appearance and execution. For the latter, the available datasets rarely contain adequate data samples. In this paper, we propose an approach to exploit the action-related information in action label sentences to combine HAR datasets that share a sufficient amount of actions with high linguistic similarity in their labels. We evaluate the effect of inter- and intra-dataset label linguistic similarity rate in the process of a crossdataset knowledge distillation. In addition, we propose a deep neural network design that enables joint learning and leverages, for each dataset, the additional training data from the other dataset, for actions with high linguistic similarity. Finally, in a series of quantitative and qualitative experiments, we show that our approach improves the performance for both datasets, compared to a single dataset learning scheme.
更多查看译文
关键词
Human action recognition, Natural language processing, Deep learning, Video understanding
AI 理解论文
溯源树
样例
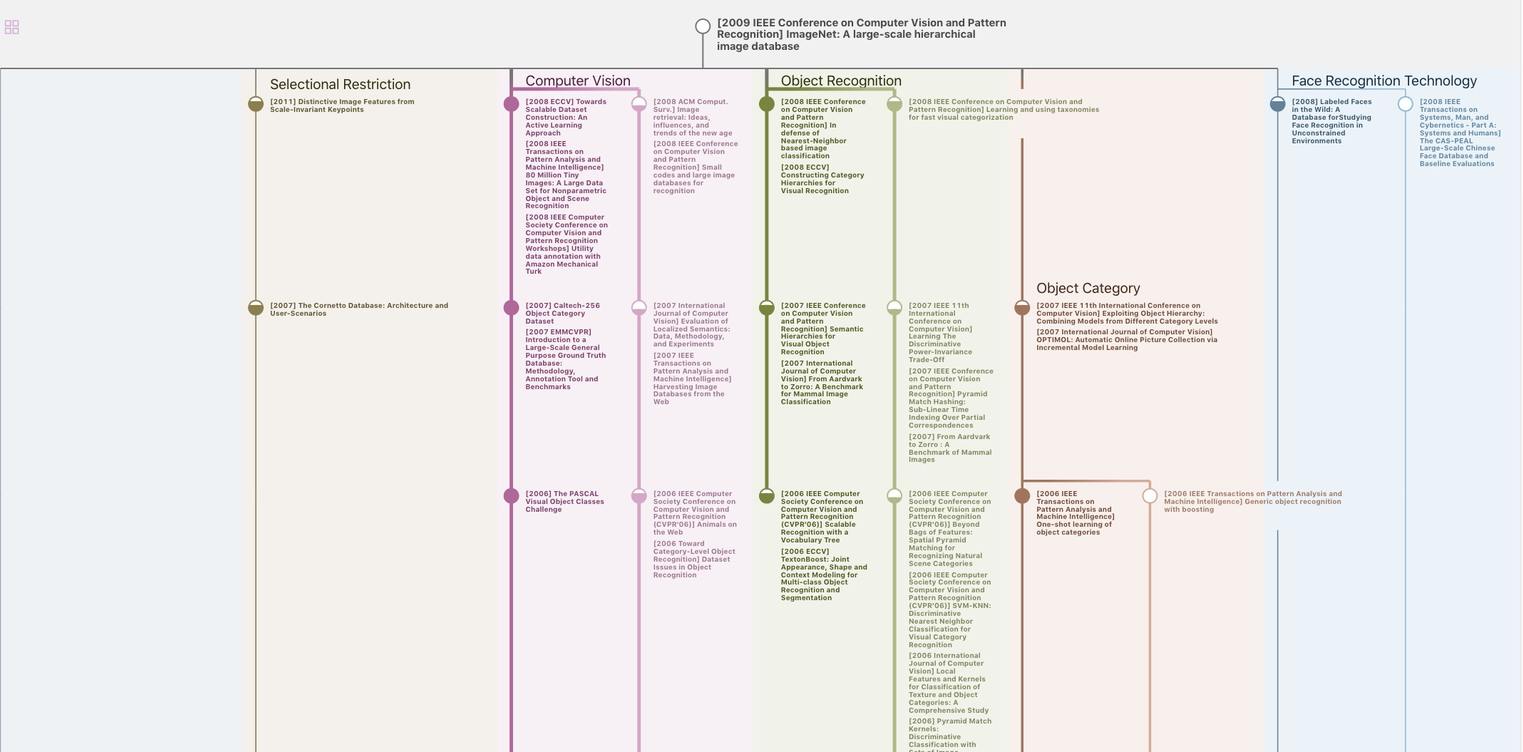
生成溯源树,研究论文发展脉络
Chat Paper
正在生成论文摘要