A Cybertwin-Driven Intelligent Offloading Method for IoV Applications Using DRL in Smart Cities
DASC/PiCom/CBDCom/CyberSciTech(2022)
摘要
Internet of Vehicle (IoV) is considered as the enabling platform to improve the traffic condition in cities. Meanwhile, a variety of promising applications such as self-driving and route planning are realized, which generates various latency-critic tasks. These latency-critic tasks present high demands on computing capacity, while resource-restricted vehicles could not satisfy those requirements. Mobile edge computing (MEC) is emerging as a potential solution to mitigate resource-limited vehicles from latency-critic tasks, which enables vehicles to offload tasks to nearby road-side units. Besides, cybertwin can abstract IoV system into a digital entity to facilitate obtaining information and management. However, it is still challenging to leverage cybertwin technology to optimize offloading policy. To this end, we design a deep reinforcement learning method to train the optimal decision-making agent by a cybertwin-driven IoV system, which aims to minimize the system cost under latency constraints. The experimental results illustrate that the agent trained by our proposed method outperforms the other methods.
更多查看译文
关键词
intelligent offloading method,smart cities,iov applications,drl,cybertwin-driven
AI 理解论文
溯源树
样例
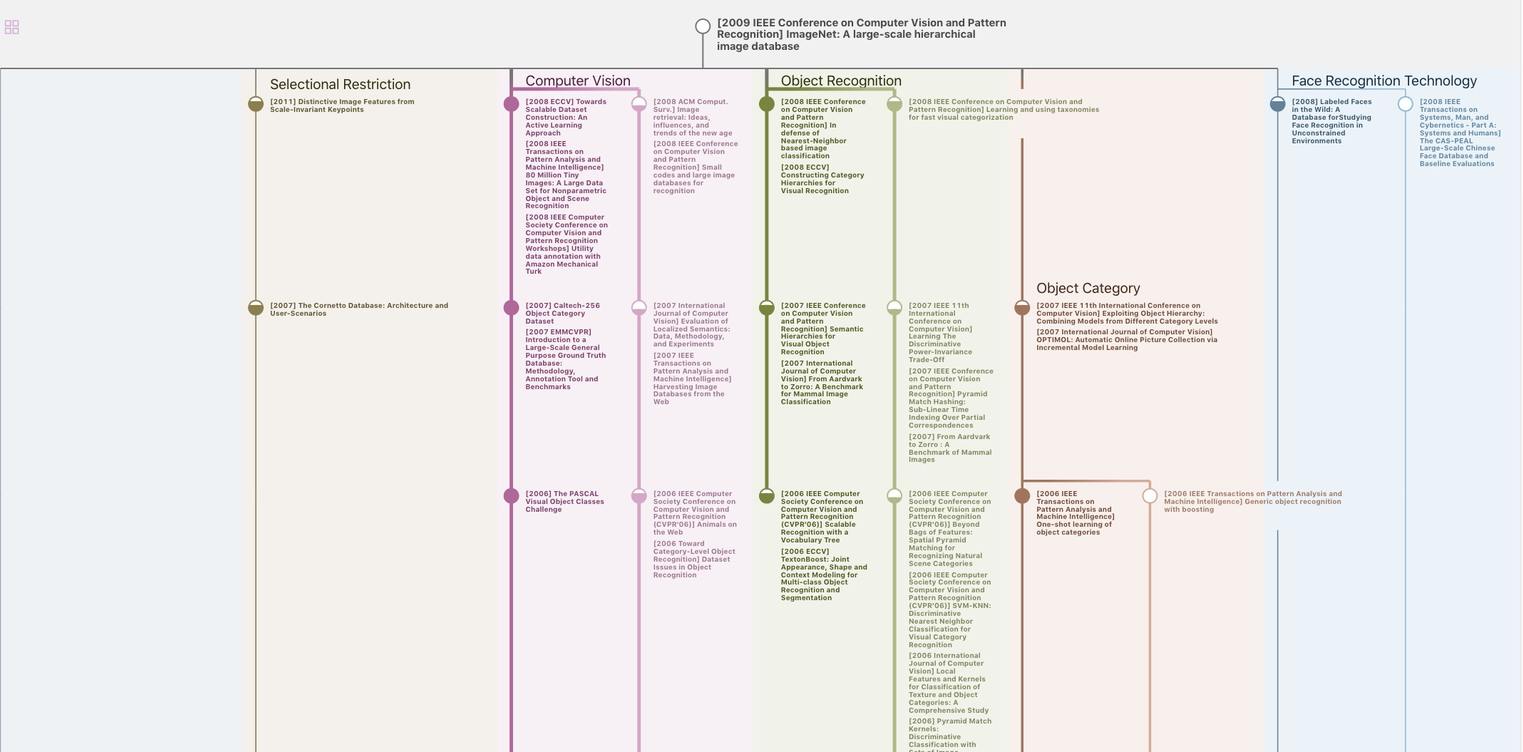
生成溯源树,研究论文发展脉络
Chat Paper
正在生成论文摘要