Estimating counterfactual treatment outcomes over time in multi-vehicle simulation.
SIGSPATIAL/GIS(2022)
摘要
Evaluation of intervention in a multi-agent system, e.g., when humans should intervene in autonomous driving systems, is challenging in various engineering and scientific fields. Estimating the individual treatment effect (ITE) using counterfactual long-term prediction is practical to evaluate such interventions. However, most of the conventional frameworks did not consider the time-varying complex structure of multi-agent relationships and covariate counterfactual prediction. Here we propose an interpretable, counterfactual recurrent network in multi-agent systems to estimate the effect of the intervention. Our model leverages graph variational recurrent neural networks and theory-based computation with domain knowledge for the ITE estimation framework based on long-term prediction of multi-agent covariates and outcomes, which can confirm the circumstances under which the intervention is effective. On simulated models of an automated vehicle with time-varying confounders, we show that our methods achieved lower estimation errors in counterfactual covariates.
更多查看译文
AI 理解论文
溯源树
样例
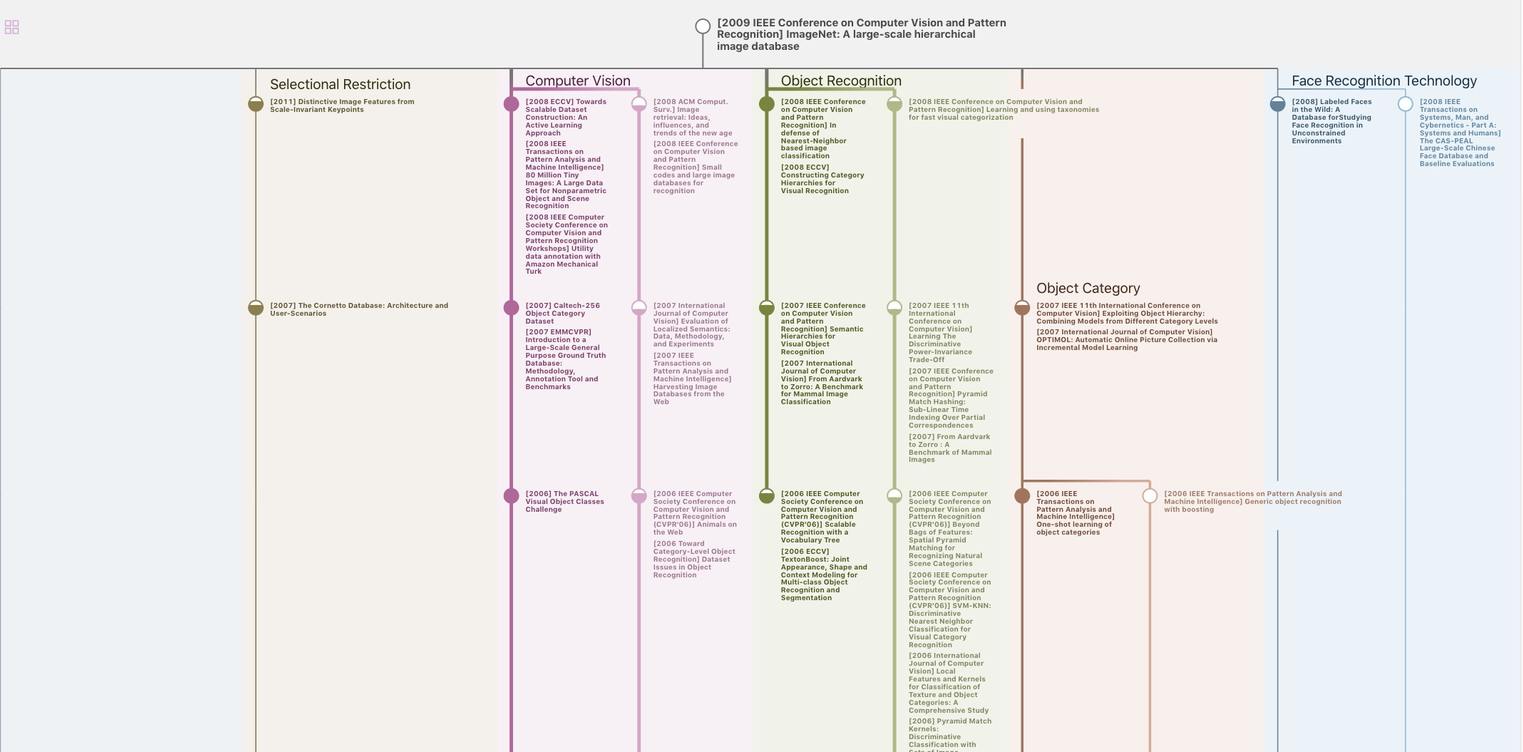
生成溯源树,研究论文发展脉络
Chat Paper
正在生成论文摘要