Automated Fish Classification Using Unprocessed Fatty Acid Chromatographic Data: A Machine Learning Approach.
AI(2022)
摘要
Fish is approximately 40% edible fillet. The remaining 60% can be processed into low-value fertilizer or high-value pharmaceutical-grade omega-3 concentrates. High-value manufacturing options depend on the composition of the biomass, which varies with fish species, fish tissue and seasonally throughout the year. Fatty acid composition, measured by Gas Chromatography, is an important measure of marine biomass quality. This technique is accurate and precise, but processing and interpreting the results is time-consuming and requires domain-specific expertise. The paper investigates different classification and feature selection algorithms for their ability to automate the processing of GasChromatography data. Experiments found that SVM could classify compositionally diverse marine biomass based on raw chromatographic fatty acid data. The SVM model is interpretable through visualization which can highlight important features for classification. Experiments demonstrated that applying feature selection significantly reduced dimensionality and improved classification performance on high-dimensional low sample-size datasets. According to the reduction rate, feature selection could accelerate the classification system up to four times.
更多查看译文
关键词
AI applications, Classification, Feature selection, High-dimensional data, Particle swarm optimization, Multidisciplinary, Gas chromatography, Fatty acid
AI 理解论文
溯源树
样例
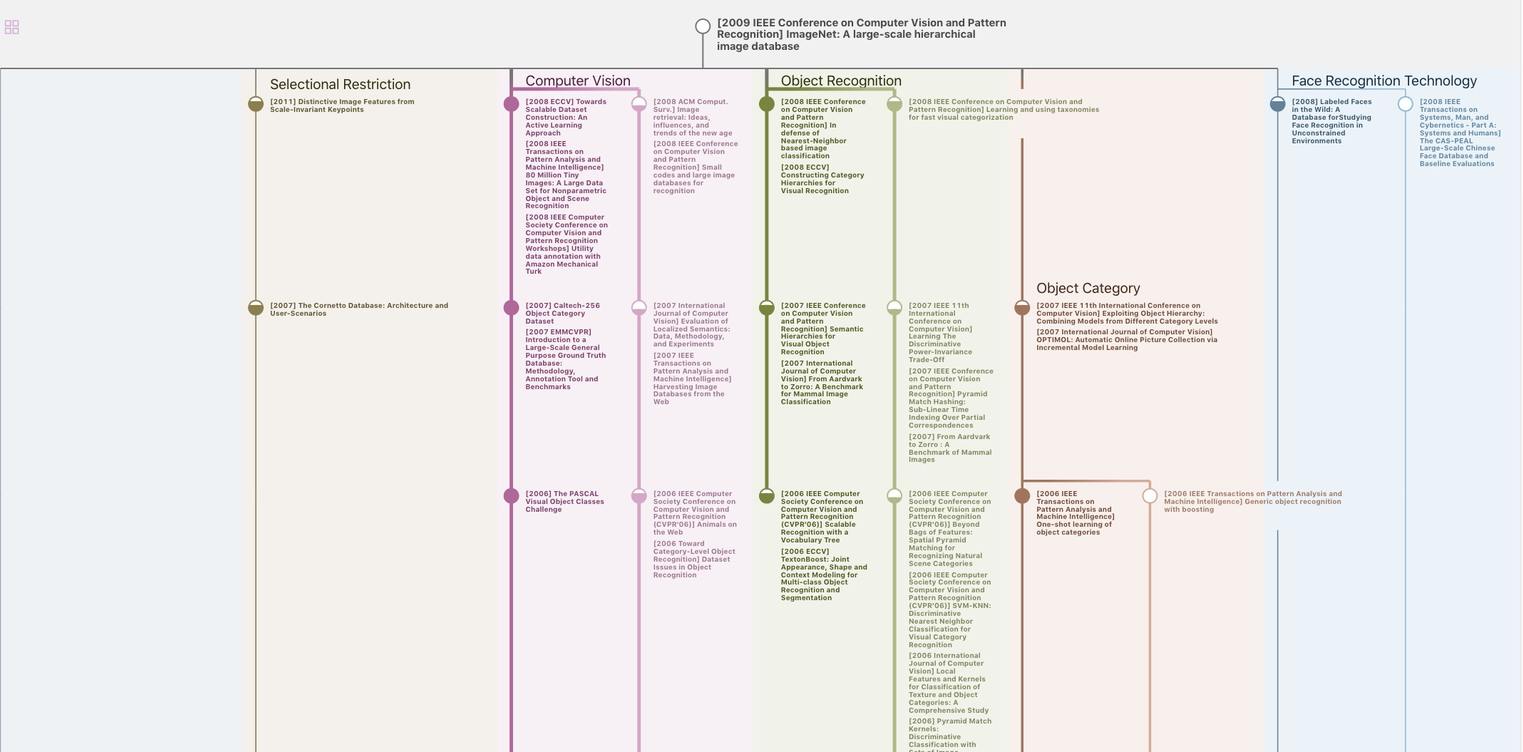
生成溯源树,研究论文发展脉络
Chat Paper
正在生成论文摘要